Using Automated Machine Learning to Predict COVID 19 Patient Survival: Identify Influential Biomarkers (Preprint)
JMIR Preprints(2020)
摘要
Background In a pandemic, it is important for clinicians to stratify patients and decide who receives limited medical resources. In this study, we used automated machine learning (autoML) to develop and compare between multiple machine learning (ML) models that predict the chance of patient survival from COVID-19 infection and identified the best-performing model. In addition, we investigated which biomarkers are the most influential in generating an accurate model. We believe an ML model such as this could be a useful tool for clinicians stratifying hospitalized SARS-CoV-2 patients.
Methods The data was retrospectively collected from Clinical Looking Glass (CLG) on all patients testing positive for COVID-19 through a nasopharyngeal specimen by real-time RT-PCR and admitted between 3/1/2020-7/3/2020 (4376 patients) at our institution. We collected 47 biomarkers from each patient within 36 hours before or after the index time: RT-PCR positivity, and tracked whether a patient survived or not for one month following this time. We utilized the autoML from H2O.ai, an open source package for R language. The autoML generated 20 ML models and ranked them by area under the precision-recall curve (AUCPR) on the test set. We selected the best model (model\_var\_47) and chose a threshold probability that maximized F2 score to make a binary classifier: dead or alive. Subsequently, we ranked the relative importance of variables that generated model\_var\_47 and chose the 10 most influential variables. Next, we reran the autoML with these 10 variables and likewise selected the model with the best AUCPR on the test set (model\_var\_10). Again, threshold probability that maximized F2 score for model\_var\_10 was chosen to make a binary classifier. We calculated and compared the sensitivity, specificity, and positive predicate value (PPV) for model\_var\_10 and model\_var\_47.
Results The best model that autoML generated using all 47 variables was the stacked ensemble model of all models (AUCPR = 0.836). The most influential variables were: systolic and diastolic blood pressure, age, respiratory rate, pulse oximetry, blood urea nitrogen, lactate dehydrogenase, d-dimer, troponin, and glucose. When the autoML was retrained with these 10 most important variables, it did not significantly affect the performance (AUCPR= 0.828). For the binary classifiers, sensitivity, specificity, and PPV of model\_var\_47 was 83.6%, 87.7%, and 69.8% respectively, while for model\_var\_10 they were 90.9%, 71.1%, and 51.8% respectively.
Conclusions By using autoML, we developed high-performing models that predict patient mortality from COVID-19 infection. In addition, we identified the most important biomarkers correlated with mortality. This ML model can be used as a decision supporting tool for medical practitioners to efficiently triage COVID-19 infected patients. From our literature review, this will be the largest COVID-19 patient cohort to train ML models and the first to utilize autoML. The COVID-19 survival calculator based on this study can be found at .
### Competing Interest Statement
The authors have declared no competing interest.
### Funding Statement
No funding.
### Author Declarations
I confirm all relevant ethical guidelines have been followed, and any necessary IRB and/or ethics committee approvals have been obtained.
Yes
The details of the IRB/oversight body that provided approval or exemption for the research described are given below:
Montefiore Medical Center
All necessary patient/participant consent has been obtained and the appropriate institutional forms have been archived.
Yes
I understand that all clinical trials and any other prospective interventional studies must be registered with an ICMJE-approved registry, such as ClinicalTrials.gov. I confirm that any such study reported in the manuscript has been registered and the trial registration ID is provided (note: if posting a prospective study registered retrospectively, please provide a statement in the trial ID field explaining why the study was not registered in advance).
Yes
I have followed all appropriate research reporting guidelines and uploaded the relevant EQUATOR Network research reporting checklist(s) and other pertinent material as supplementary files, if applicable.
Yes
Data is availably for reproducibility.
更多查看译文
关键词
survival,automated-machine
AI 理解论文
溯源树
样例
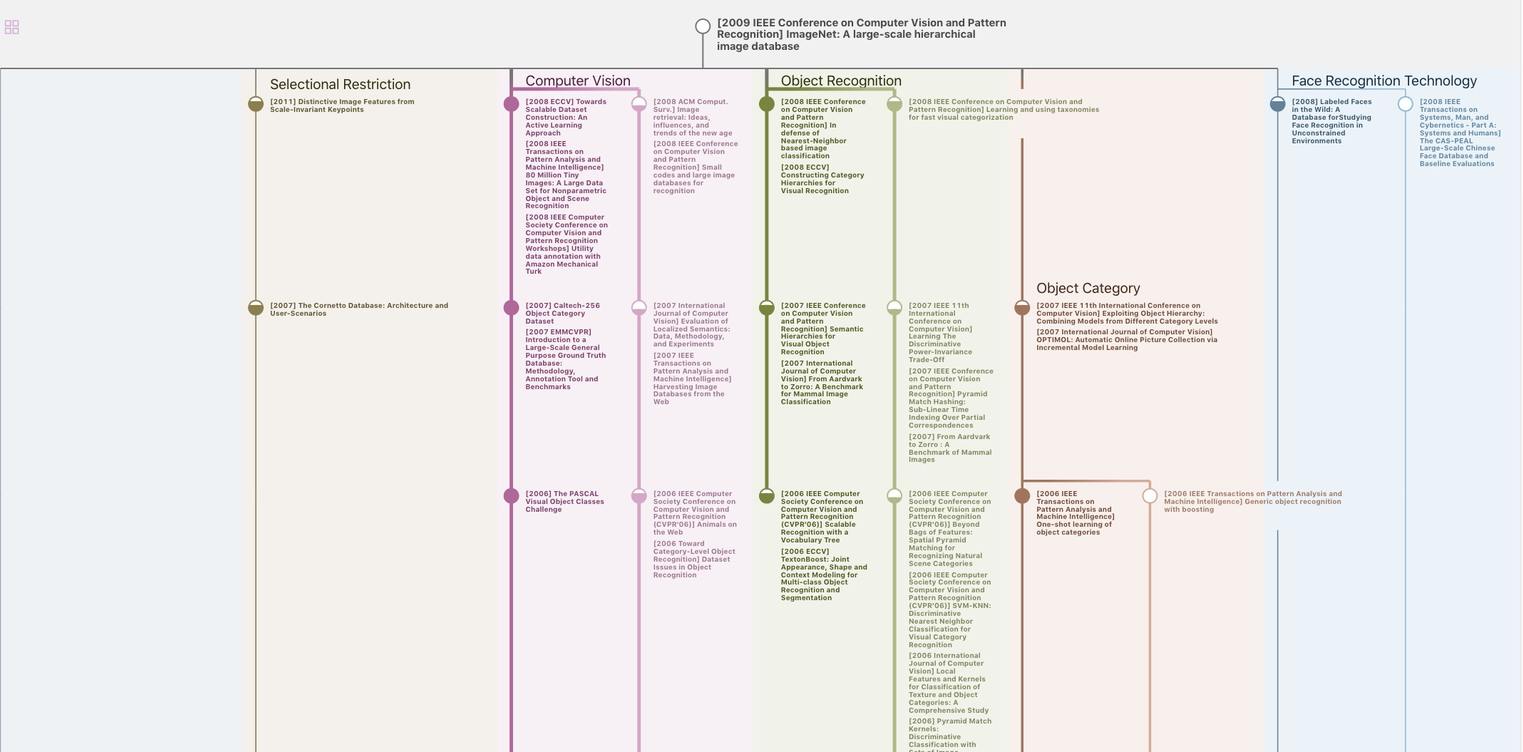
生成溯源树,研究论文发展脉络
Chat Paper
正在生成论文摘要