Multi-Scale One-Class Recurrent Neural Networks for Discrete Event Sequence Anomaly Detection
Knowledge Discovery and Data Mining(2021)
摘要
ABSTRACTDiscrete event sequences are ubiquitous, such as an ordered event series of process interactions in Information and Communication Technology systems. Recent years have witnessed increasing efforts in detecting anomalies with discrete event sequences. However, it remains an extremely difficult task due to several intrinsic challenges including data imbalance issues, discrete property of the events, and sequential nature of the data. To address these challenges, in this paper, we propose OC4Seq, a multi-scale one-class recurrent neural network for detecting anomalies in discrete event sequences. Specifically, OC4Seq integrates the anomaly detection objective with recurrent neural networks (RNNs) to embed the discrete event sequences into latent spaces, where anomalies can be easily detected. In addition, given that an anomalous sequence could be caused by either individual events, subsequences of events, or the whole sequence, we design a multi-scale RNN framework to capture different levels of sequential patterns simultaneously. We fully implement and evaluate OC4Seq on three real-world system log datasets. The results show that OC4Seq consistently outperforms various representative baselines by a large margin. Moreover, through both quantitative and qualitative analysis, the importance of capturing multi-scale sequential patterns for event anomaly detection is verified. To encourage reproducibility, we make the code and data publicly available.
更多查看译文
关键词
Anomaly detection, event sequence modeling, one-class recurrent neural network, multi-scale sequential pattern mining
AI 理解论文
溯源树
样例
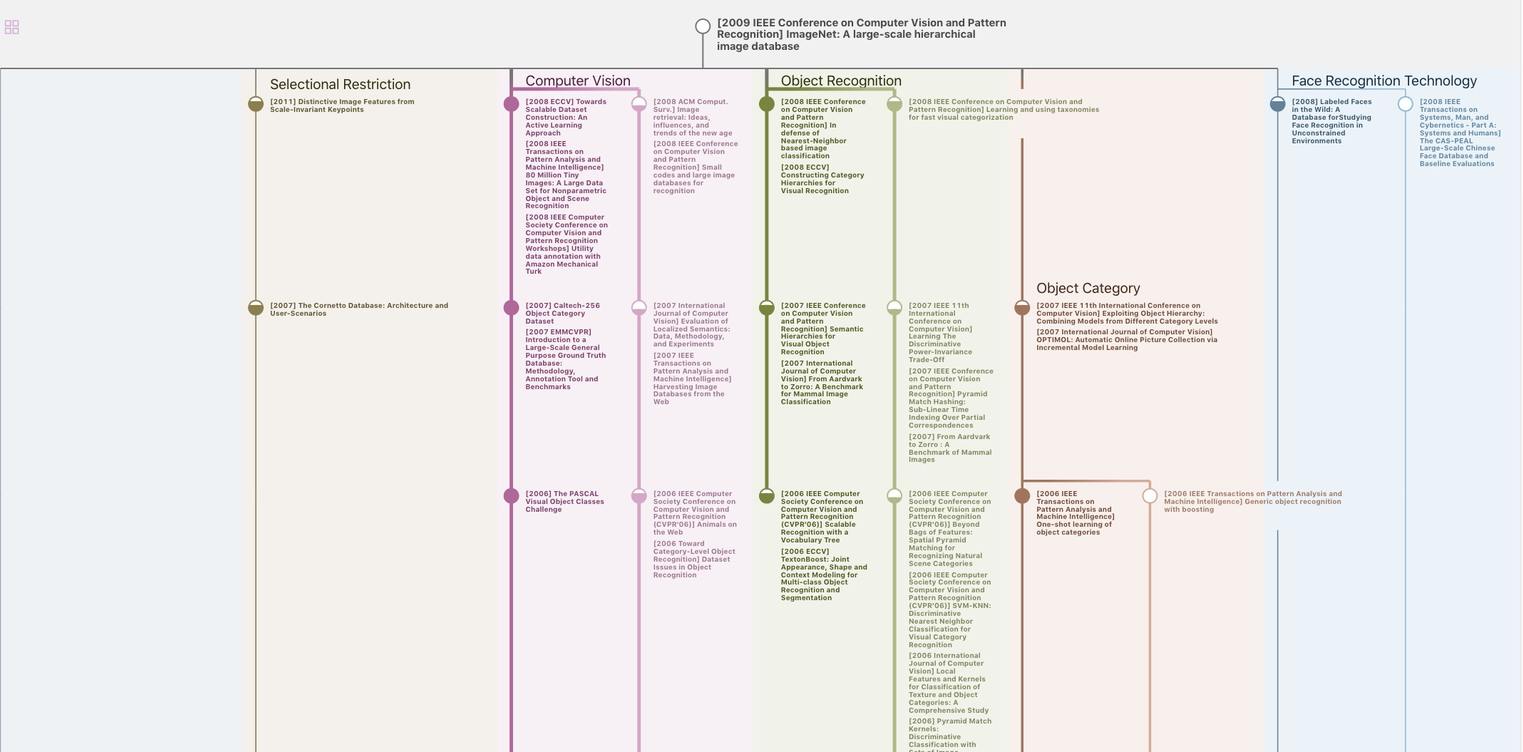
生成溯源树,研究论文发展脉络
Chat Paper
正在生成论文摘要