Polarimetric decomposition methods for LULC mapping using ALOS L-band PolSAR data in Western parts of Mizoram, Northeast India
SN Applied Sciences(2020)
摘要
The rapid advancement of remote sensing and availability of polarimetric SAR (PolSAR) data have facilitated to monitor the land use land cover (LULC) dynamics. In the recent past, polarimetric decomposition theorems are applied widely to perform LULC classification with the help of machine learning techniques. In this study, we utilized ALOS PALSAR-1 L-band quad polarimetric data for performing polarimetric decomposition, textural information extraction, and to generate LULC maps over the western part of Mizoram state, northeast India. The study area comprises three districts, namely Mamit, Lunglei, and Lawngtlai. We adopted two representative full-polarimetric decomposition models: classical model-based Freeman–Durden and Yamaguchi decomposition. These methods decompose the coherency matrix of PolSAR images into surface, double-bounce, and volume scattering. Textural measures, such as variance, contrast, entropy, homogeneity, dissimilarity, and uniformity are also retrieved using grey-level co-occurrence matrix (GLCM) for LULC classification. For LULC classification, we employed a support vector machine classifier and calculated the area statistics of LULC. The outcomes were checked with the help of confusion matrix derived for six classes, such as built-up, deciduous forest, evergreen forest, scrubland, bareland, and waterbody. Each LULC class is separated using the scattering properties of PolSAR images. Results exhibited that Yamaguchi four-component decomposition (overall accuracy 90% and kappa coefficient 0.88) gives relatively better LULC classification results than the Freeman–Durden three-component decomposition (overall accuracy 87% and kappa coefficient 0.84). Use of textural images of GLCM has supported the classification accuracy at par with the Yamaguchi model. Integration of polarimetric information offers a new dimension in LULC classification and produces high accuracy maps. This approach overcomes the limitations of optical data in cloud covering areas, and furthermore, it provides better classification accuracy.
更多查看译文
关键词
L-band SAR, Polarimetric SAR, Polarimetric decompositions, GLCM, Support vector machines, LULC mapping
AI 理解论文
溯源树
样例
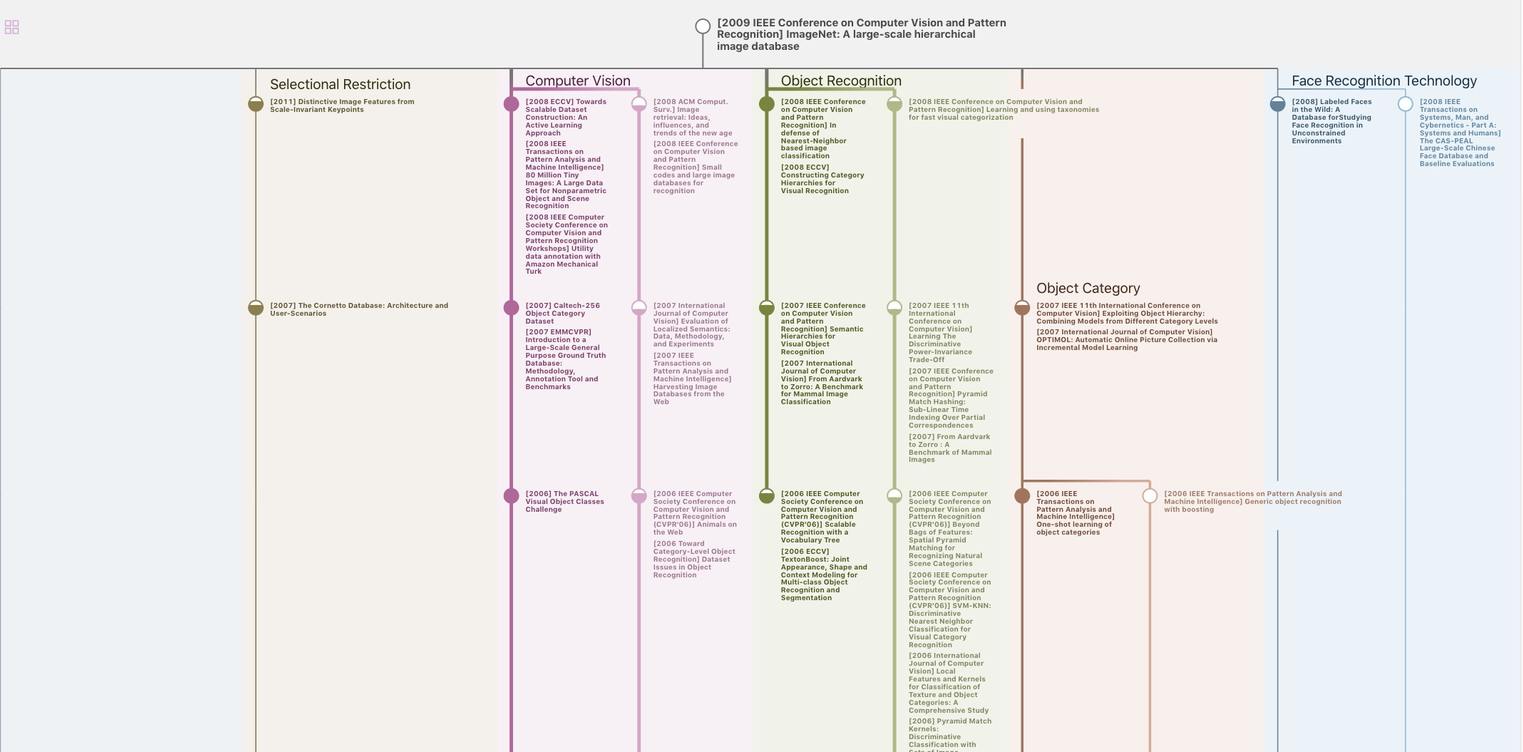
生成溯源树,研究论文发展脉络
Chat Paper
正在生成论文摘要