Adaptive balancing of exploration and exploitation around the edge of chaos in internal-chaos-based learning
Neural Networks(2020)
摘要
This paper addresses learning with exploration driven by chaotic internal dynamics of a neural network. Hoerzer et al. showed that a chaotic reservoir network (RN) can learn with exploration driven by external random noise and a sequential reward. In this paper, we demonstrate that a chaotic RN can learn without external noise because the output fluctuation originated from its internal chaotic dynamics functions as exploration. As learning progresses, the chaoticity decreases and the network can automatically switch from exploration mode to exploitation mode. Furthermore, the network can resume exploration when presented with a new situation. In addition, we found that even when the two parameters that influence the chaoticity are varied, learning performance always improves around the edge of chaos. From these results, we think that exploration is generated from internal chaotic dynamics, and exploitation appears in the process of forming attractors on the chaotic dynamics through learning. Consequently, exploration and exploitation are well-balanced around the edge of chaos, which leads to good learning performance.
更多查看译文
关键词
Chaotic neural network,Reservoir computing,Reward-modulated Hebbian learning,Edge of chaos,Exploration–exploitation dilemma
AI 理解论文
溯源树
样例
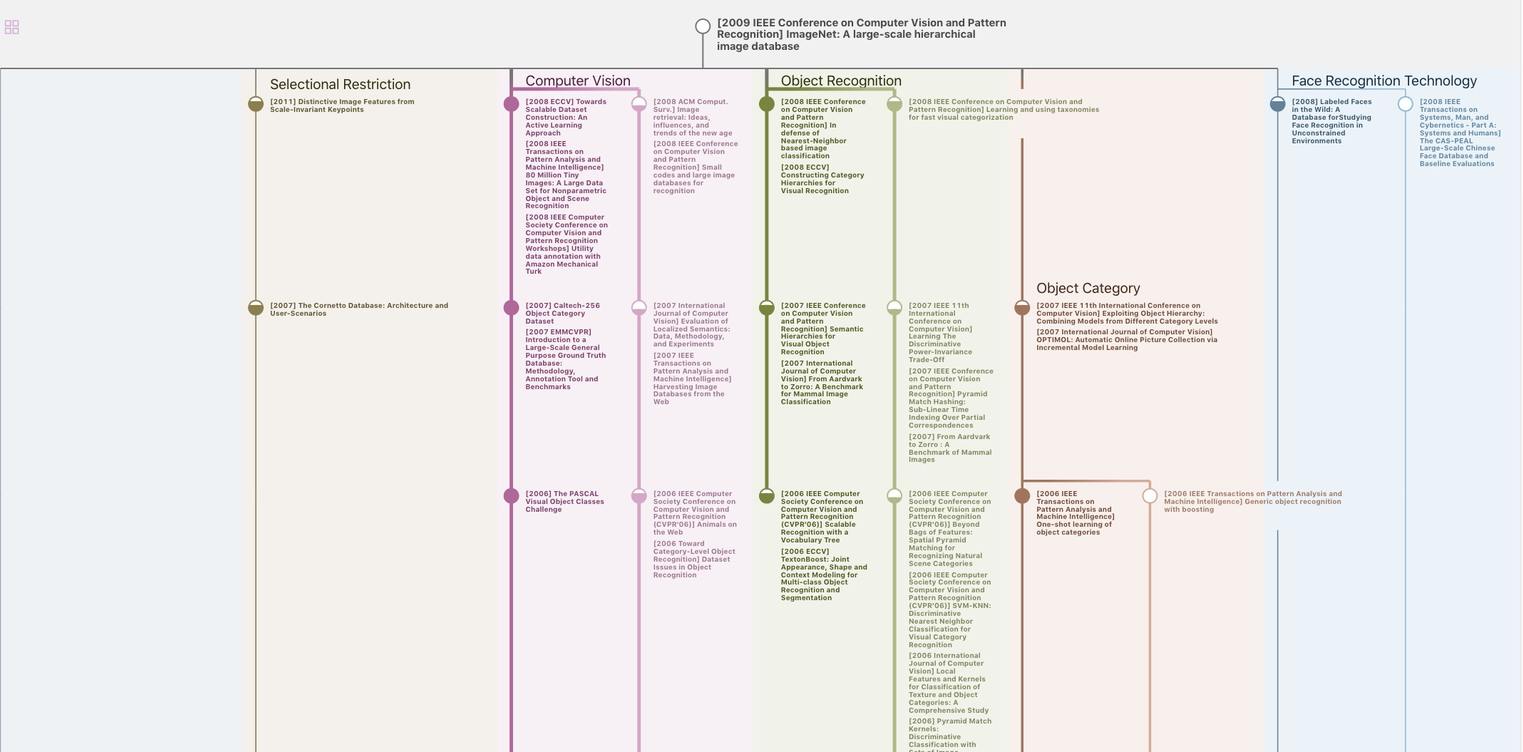
生成溯源树,研究论文发展脉络
Chat Paper
正在生成论文摘要