Rate Distortion Optimization Over Large Scale Video Corpus With Machine Learning
2020 IEEE INTERNATIONAL CONFERENCE ON IMAGE PROCESSING (ICIP)(2020)
摘要
We present an efficient codec-agnostic method for bitrate allocation over a large scale video corpus with the goal of minimizing the average bitrate subject to constraints on average and minimum quality. Our method clusters the videos in the corpus such that videos within one cluster have similar rate-distortion (R-D) characteristics. We train a support vector machine classifier to predict the R-D cluster of a video using simple video complexity features that are computationally easy to obtain. The model allows us to classify a large sample of the corpus in order to estimate the distribution of the number of videos in each of the clusters. We use this distribution to find the optimal encoder operating point for each R-D cluster. Experiments with AV1 encoder show that our method can achieve the same average quality over the corpus with 22% less average bitrate.
更多查看译文
关键词
rate distortion optimization, clustering, machine learning, adaptive streaming, YouTube
AI 理解论文
溯源树
样例
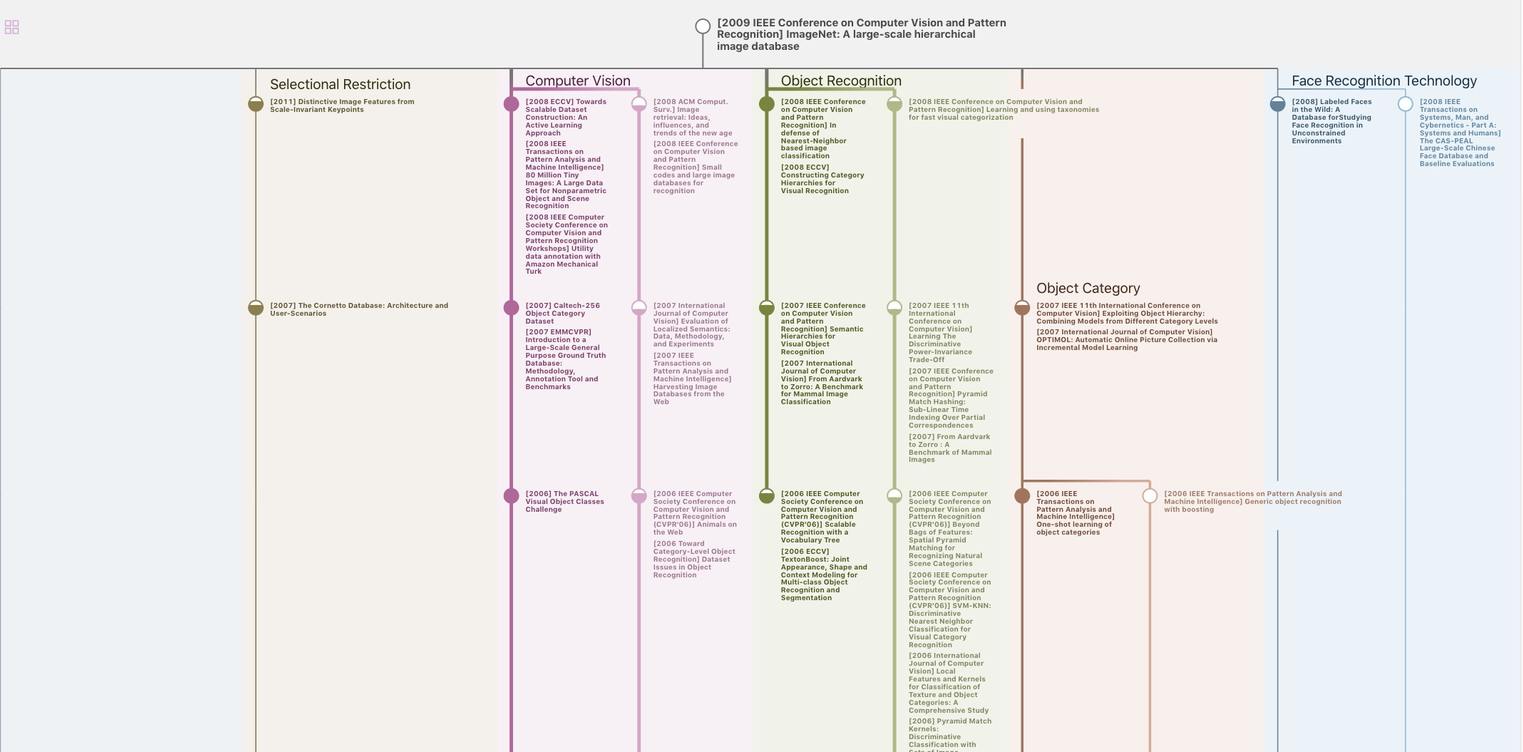
生成溯源树,研究论文发展脉络
Chat Paper
正在生成论文摘要