A knowledge-based approach for representing jobholder profile toward optimal human–machine collaboration in cyber physical production systems
CIRP Journal of Manufacturing Science and Technology(2020)
摘要
Increasing number of AI-enhanced approaches provide helpful “know-how” for reproducing and imitating skills and finally substituting human jobs with algorithms and intelligent machines. However, complementarity of human and machine, especially in hybrid man-machine settings is still not sufficiently explored. The main objective of this paper is to establish a twofold qualitative and quantitative methodology for optimal selection of a competent jobholder(s) to perform a certain task by semantic modeling and analysis of jobholder (human and machine) profiles corresponding to the task characteristics and learning requirements including knowledge, skills and competences (KSCs). The proposed knowledge-based approach comprises semantic modeling and quantitative methods focusing on measuring and correlating the level of human competences and machine autonomy, and identifying the extent of human–machine complementarity in performing an assigned task. The Vector of Competence and Autonomy (VCA) is built for identifying the extent of human–machine collaboration. The quantitative analysis involves several human factors in association to various combinations of technological components of Digital Assistance Systems with different automation degrees, under certain aspects of complexity of products and workplaces. Applying a set of rules and considering jobholder profiles, VCA values are interpreted and the current state of complementarity is inferred. Furthermore, feasible radical or incremental managerial transition pathways are identified as an initial step to reach the optimal (desired) level of human–machine collaboration in the example of TU Wien Pilot Factory Industry 4.0.
更多查看译文
关键词
Human,CPPS,Competence,Autonomy,Smart factory,Industry 4.0
AI 理解论文
溯源树
样例
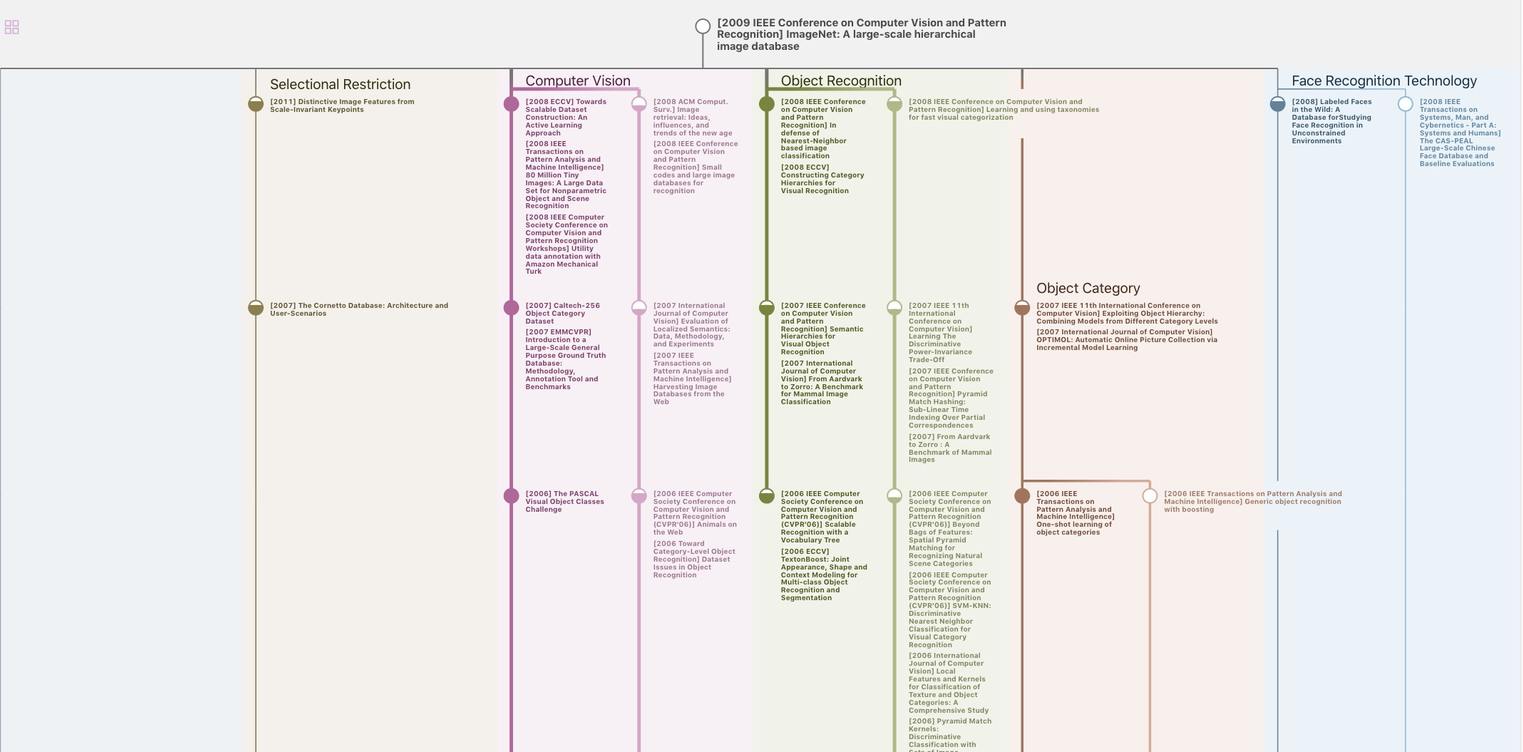
生成溯源树,研究论文发展脉络
Chat Paper
正在生成论文摘要