PIGNet: a physics-informed deep learning model toward generalized drug-target interaction predictions
CHEMICAL SCIENCE(2022)
摘要
Recently, deep neural network (DNN)-based drug-target interaction (DTI) models were highlighted for their high accuracy with affordable computational costs. Yet, the models' insufficient generalization remains a challenging problem in the practice of in silico drug discovery. We propose two key strategies to enhance generalization in the DTI model. The first is to predict the atom-atom pairwise interactions via physics-informed equations parameterized with neural networks and provides the total binding affinity of a protein-ligand complex as their sum. We further improved the model generalization by augmenting a broader range of binding poses and ligands to training data. We validated our model, PIGNet, in the comparative assessment of scoring functions (CASF) 2016, demonstrating the outperforming docking and screening powers than previous methods. Our physics-informing strategy also enables the interpretation of predicted affinities by visualizing the contribution of ligand substructures, providing insights for further ligand optimization.
更多查看译文
关键词
deep learning model,interaction,physics-informed,drug-target
AI 理解论文
溯源树
样例
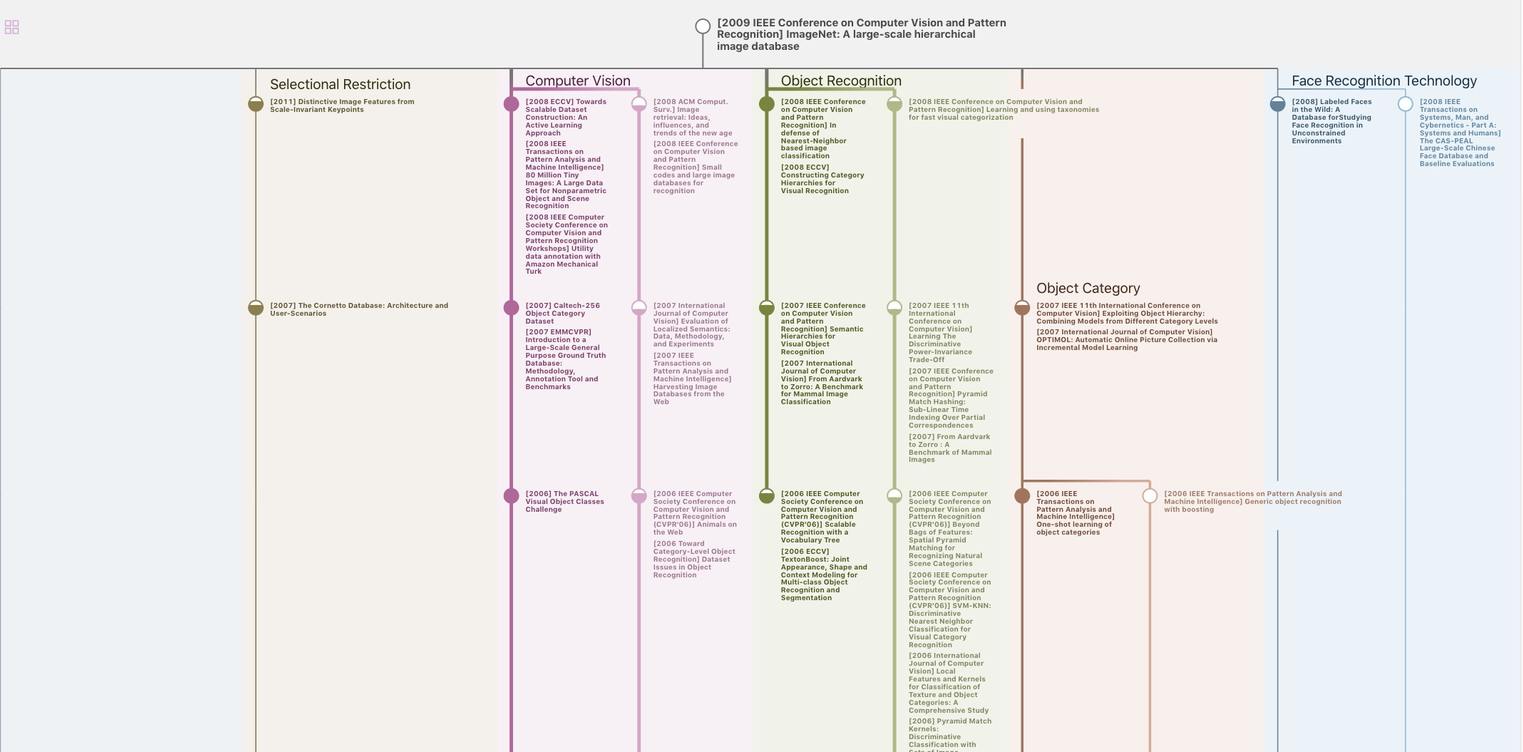
生成溯源树,研究论文发展脉络
Chat Paper
正在生成论文摘要