Learning Condition Invariant Features for Retrieval-Based Localization from 1M Images
arxiv(2020)
摘要
Image features for retrieval-based localization must be invariant to dynamic objects (e.g. cars) as well as seasonal and daytime changes. Such invariances are, up to some extent, learnable with existing methods using triplet-like losses, given a large number of diverse training images. However, due to the high algorithmic training complexity, there exists insufficient comparison between different loss functions on large datasets. In this paper, we train and evaluate several localization methods on three different benchmark datasets, including Oxford RobotCar with over one million images. This large scale evaluation yields valuable insights into the generalizability and performance of retrieval-based localization. Based on our findings, we develop a novel method for learning more accurate and better generalizing localization features. It consists of two main contributions: (i) a feature volume-based loss function, and (ii) hard positive and pairwise negative mining. On the challenging Oxford RobotCar night condition, our method outperforms the well-known triplet loss by 24.4% in localization accuracy within 5m.
更多查看译文
AI 理解论文
溯源树
样例
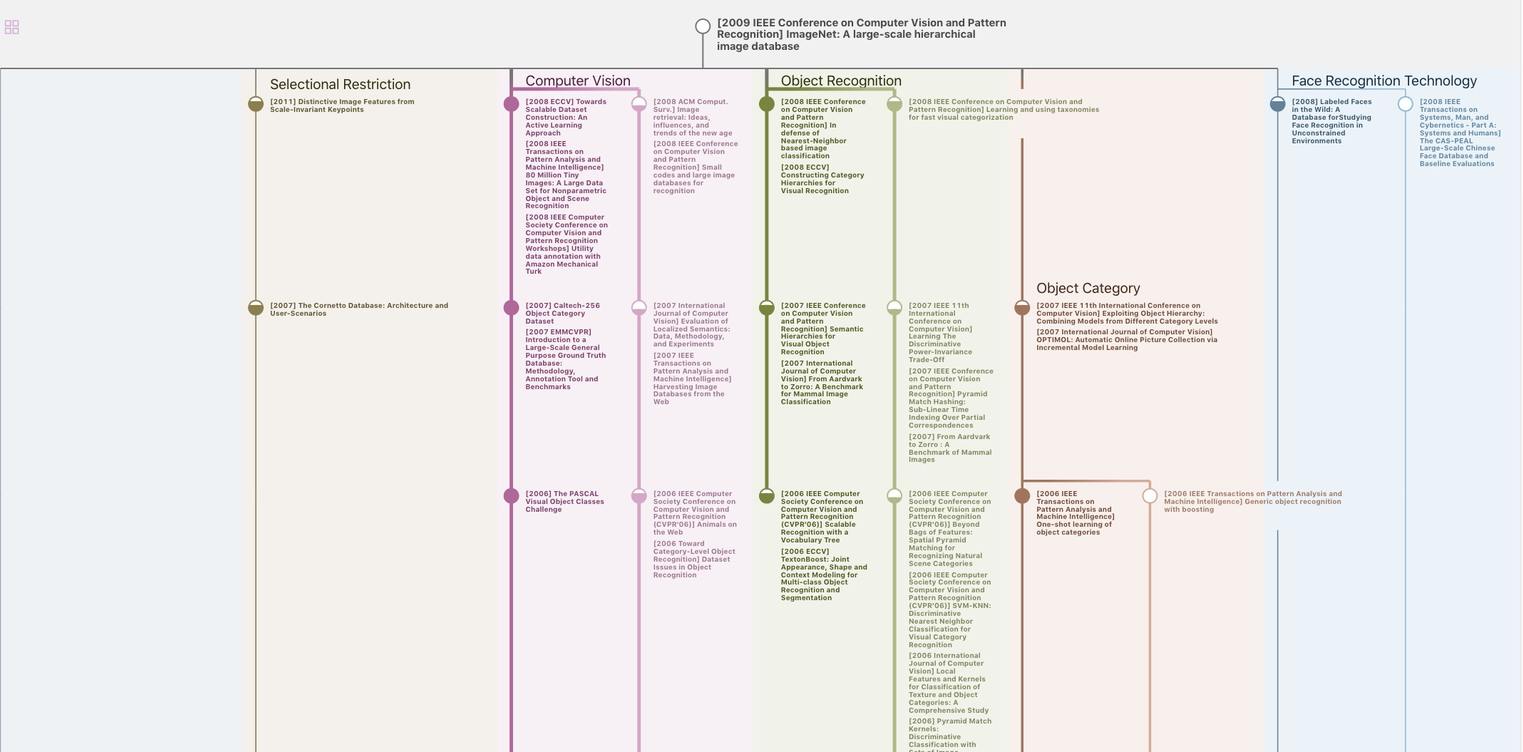
生成溯源树,研究论文发展脉络
Chat Paper
正在生成论文摘要