Domain Invariant Representations: A Look on Compression and Weights
user-5ef9583f4c775ed682ecca0f(2019)
摘要
Learning Invariant Representations to adapt deep classifiers of a source domain to a new target domain has recently attracted much attention. In this paper, we show that the search for invariance favors the compression of representations. We point out this may have a bad impact on adaptability of representations expressed as a minimal combined domain error. By considering the risk of compression, we show that weighting representations can align representation distributions without impacting their adaptability. This supports the claim that representation invariance is too strict a constraint. First, we introduce a new bound on the target risk that reveals a trade-off between compression and invariance of learned representations. More precisely, our results show that the adaptability of a representation can be better controlled when the compression risk is taken into account. In contrast, preserving adaptability may overestimate the risk of compression that makes the bound impracticable. We support these statements with a theoretical analysis illustrated on a standard domain adaptation benchmark. Second, we show that learning weighted representations plays a key role in relaxing the constraint of invariance and then preserving the risk of compression. Taking advantage of this trade-off may open up promising directions for the design of new adaptation methods.
更多查看译文
AI 理解论文
溯源树
样例
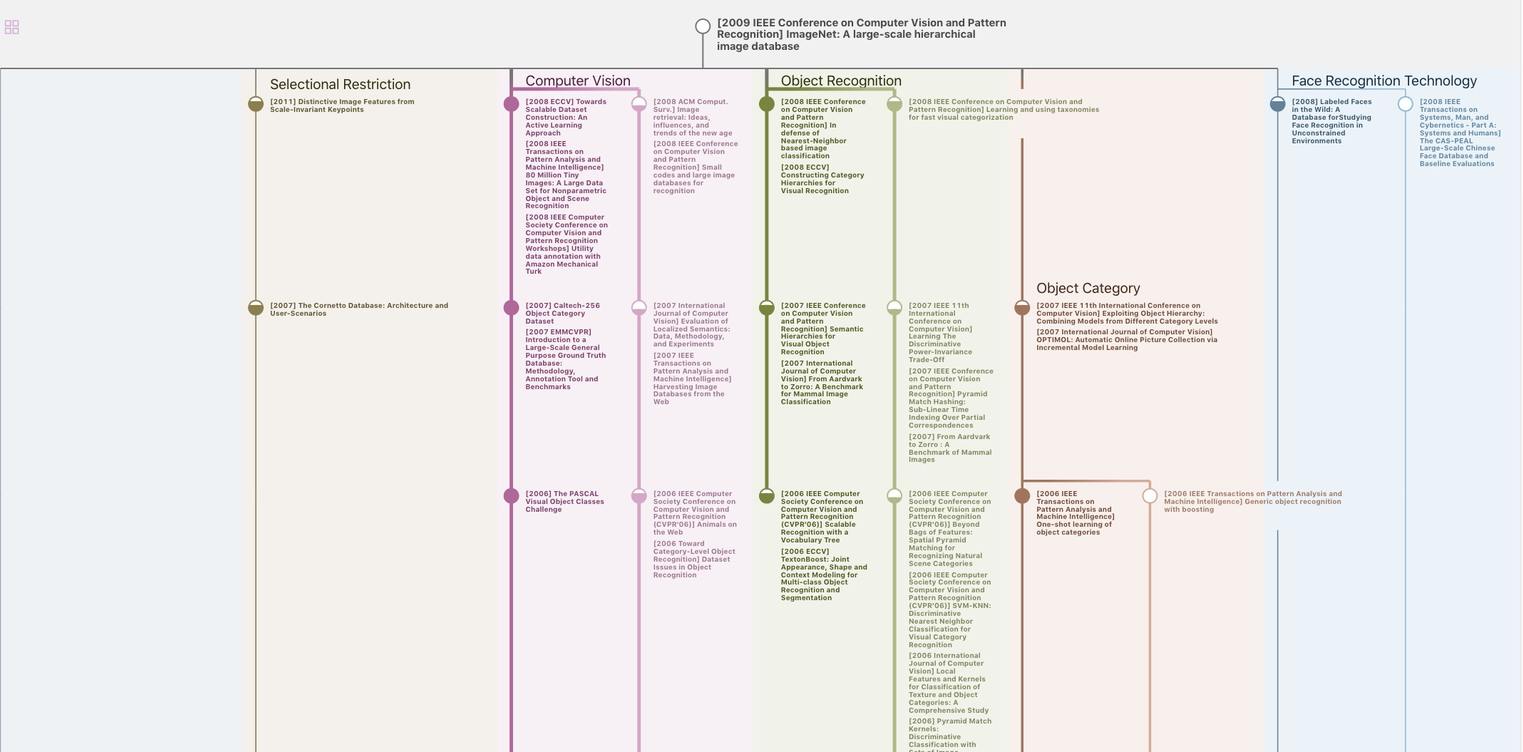
生成溯源树,研究论文发展脉络
Chat Paper
正在生成论文摘要