MutaGAN: A sequence-to-sequence GAN framework to predict mutations of evolving protein populations.
Virus evolution(2023)
摘要
The ability to predict the evolution of a pathogen would significantly improve the ability to control, prevent, and treat disease. Machine learning, however, is yet to be used to predict the evolutionary progeny of a virus. To address this gap, we developed a novel machine learning framework, named MutaGAN, using generative adversarial networks with sequence-to-sequence, recurrent neural networks generator to accurately predict genetic mutations and evolution of future biological populations. MutaGAN was trained using a generalized time-reversible phylogenetic model of protein evolution with maximum likelihood tree estimation. MutaGAN was applied to influenza virus sequences because influenza evolves quickly and there is a large amount of publicly available data from the National Center for Biotechnology Information's Influenza Virus Resource. MutaGAN generated 'child' sequences from a given 'parent' protein sequence with a median Levenshtein distance of 4.00 amino acids. Additionally, the generator was able to generate sequences that contained at least one known mutation identified within the global influenza virus population for 72.8 per cent of parent sequences. These results demonstrate the power of the MutaGAN framework to aid in pathogen forecasting with implications for broad utility in evolutionary prediction for any protein population.
更多查看译文
关键词
Influenza virus,deep learning,evolution,generative adversarial networks,sequence generation
AI 理解论文
溯源树
样例
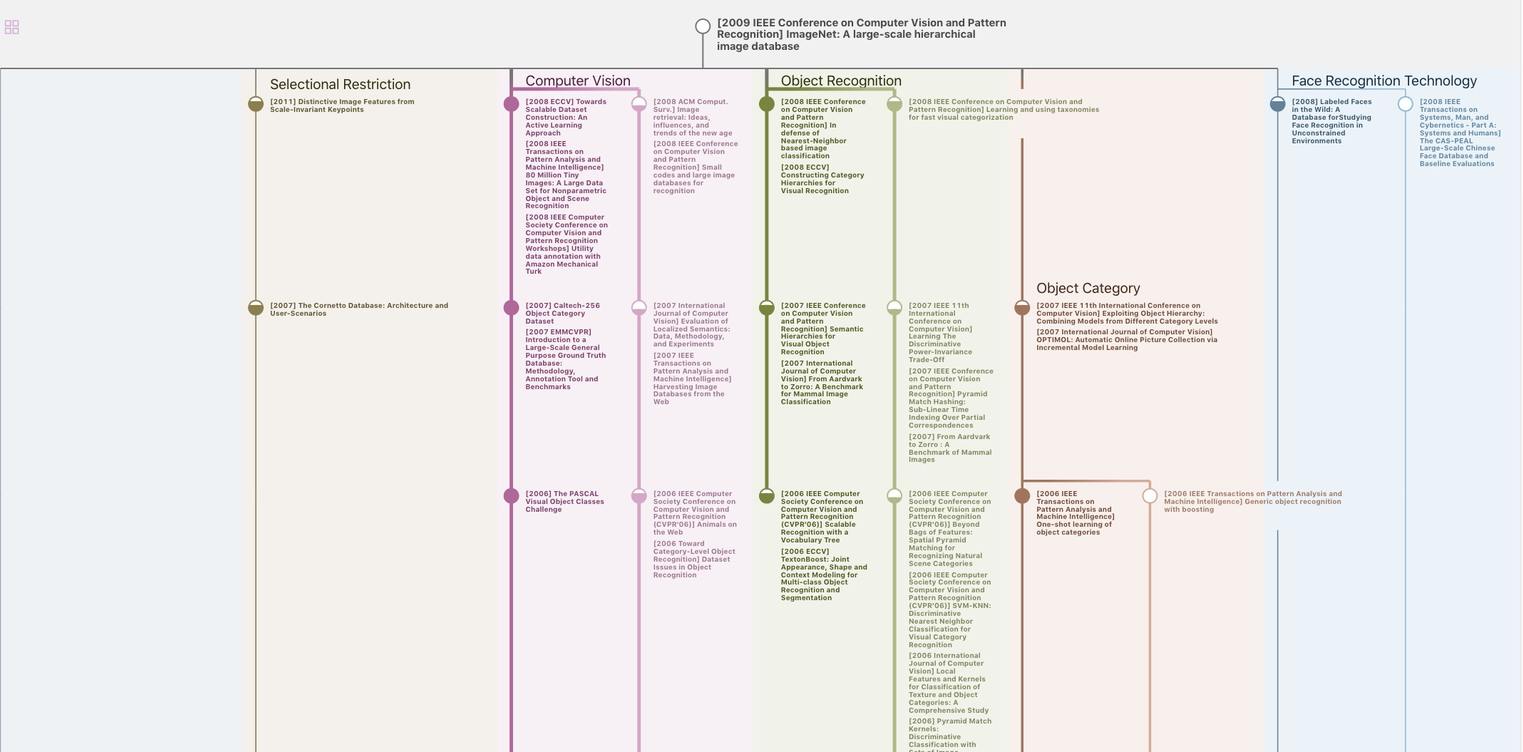
生成溯源树,研究论文发展脉络
Chat Paper
正在生成论文摘要