Simplifying Architecture Search for Graph Neural Network
CIKM(2020)
摘要
Recent years have witnessed the popularity of Graph Neural Networks (GNN) in various scenarios. To obtain optimal data-specific GNN architectures, researchers turn to neural architecture search (NAS) methods, which have made impressive progress in discovering effective architectures in convolutional neural networks. Two preliminary works, GraphNAS and Auto-GNN, have made first attempt to apply NAS methods to GNN. Despite the promising results, there are several drawbacks in expressive capability and search efficiency of GraphNAS and Auto-GNN due to the designed search space. To overcome these drawbacks, we propose the SNAG framework (Simplified Neural Architecture search for Graph neural networks), consisting of a novel search space and a reinforcement learning based search algorithm. Extensive experiments on real-world datasets demonstrate the effectiveness of the SNAG framework compared to human-designed GNNs and NAS methods, including GraphNAS and Auto-GNN.
更多查看译文
关键词
architecture search,neural network,graph
AI 理解论文
溯源树
样例
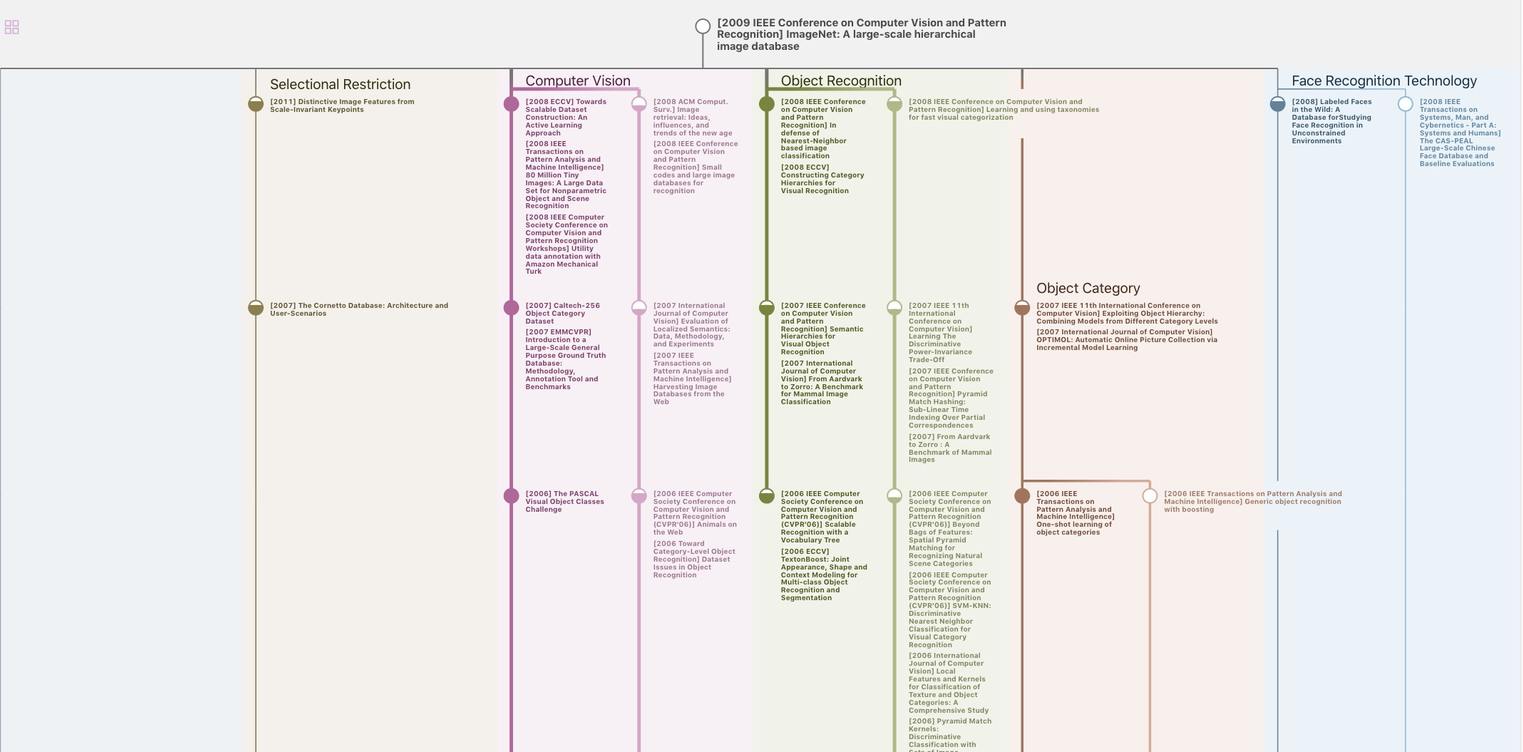
生成溯源树,研究论文发展脉络
Chat Paper
正在生成论文摘要