What-If Motion Prediction for Autonomous Driving
arxiv(2020)
摘要
Forecasting the long-term future motion of road actors is a core challenge to the deployment of safe autonomous vehicles (AVs). Viable solutions must account for both the static geometric context, such as road lanes, and dynamic social interactions arising from multiple actors. While recent deep architectures have achieved state-of-the-art performance on distance-based forecasting metrics, these approaches produce forecasts that are predicted without regard to the AV's intended motion plan. In contrast, we propose a recurrent graph-based attentional approach with interpretable geometric (actor-lane) and social (actor-actor) relationships that supports the injection of counterfactual geometric goals and social contexts. Our model can produce diverse predictions conditioned on hypothetical or "what-if" road lanes and multi-actor interactions. We show that such an approach could be used in the planning loop to reason about unobserved causes or unlikely futures that are directly relevant to the AV's intended route.
更多查看译文
关键词
motion prediction
AI 理解论文
溯源树
样例
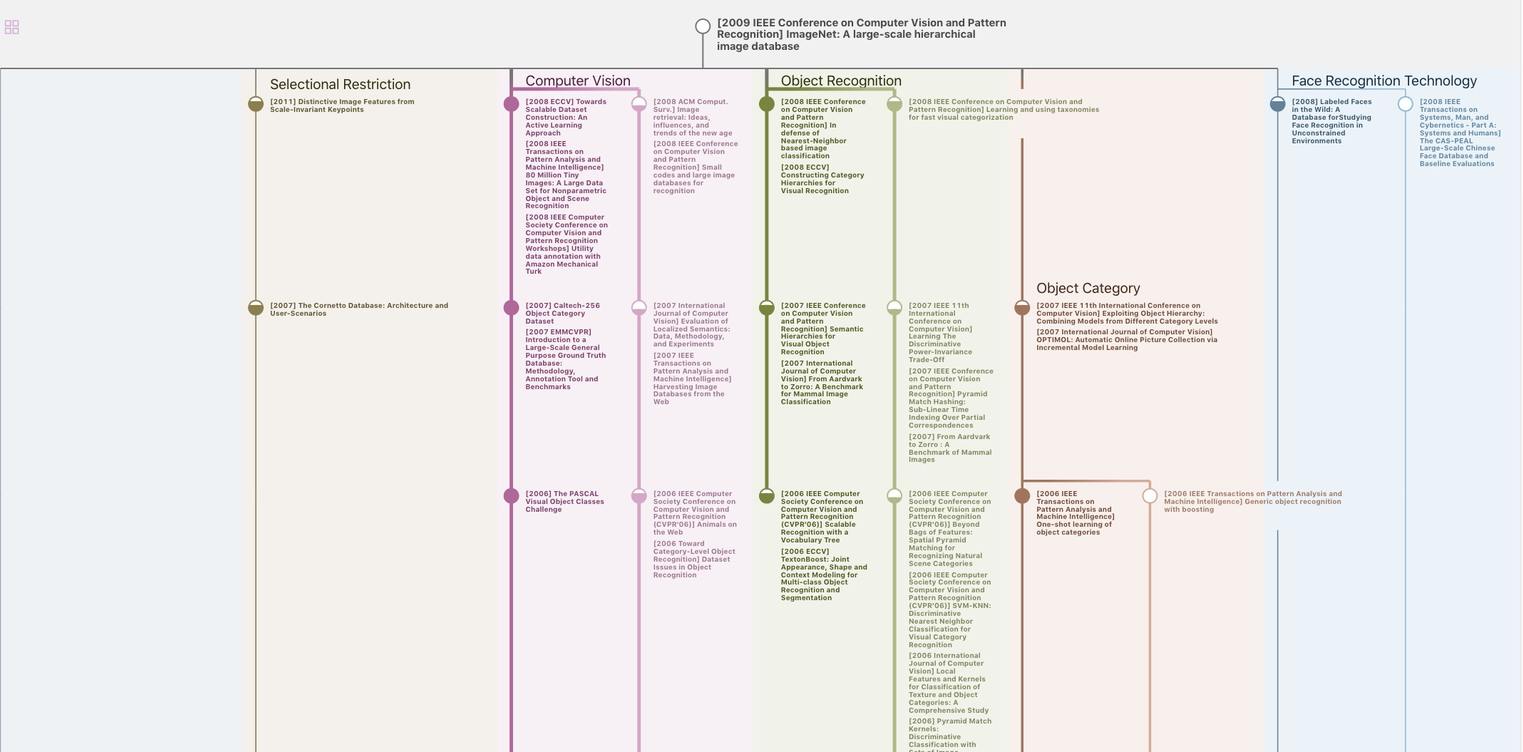
生成溯源树,研究论文发展脉络
Chat Paper
正在生成论文摘要