An Autoencoder-Based Reduced-Order Model For Eigenvalue Problems With Application To Neutron Diffusion
INTERNATIONAL JOURNAL FOR NUMERICAL METHODS IN ENGINEERING(2021)
摘要
Using an autoencoder for dimensionality reduction, this article presents a novel projection-based reduced-order model for eigenvalue problems. Reduced-order modeling relies on finding suitable basis functions which define a low-dimensional space in which a high-dimensional system is approximated. Proper orthogonal decomposition (POD) and singular value decomposition (SVD) are often used for this purpose and yield an optimal linear subspace. Autoencoders provide a nonlinear alternative to POD/SVD, that may capture, more efficiently, features or patterns in the high-fidelity model results. Reduced-order models based on an autoencoder and a novel hybrid SVD-autoencoder are developed. These methods are compared with the standard POD-Galerkin approach and are applied to two test cases taken from the field of nuclear reactor physics.
更多查看译文
关键词
autoencoder, machine learning, reduced-order modeling, model reduction, neutron diffusion equation, reactor physics
AI 理解论文
溯源树
样例
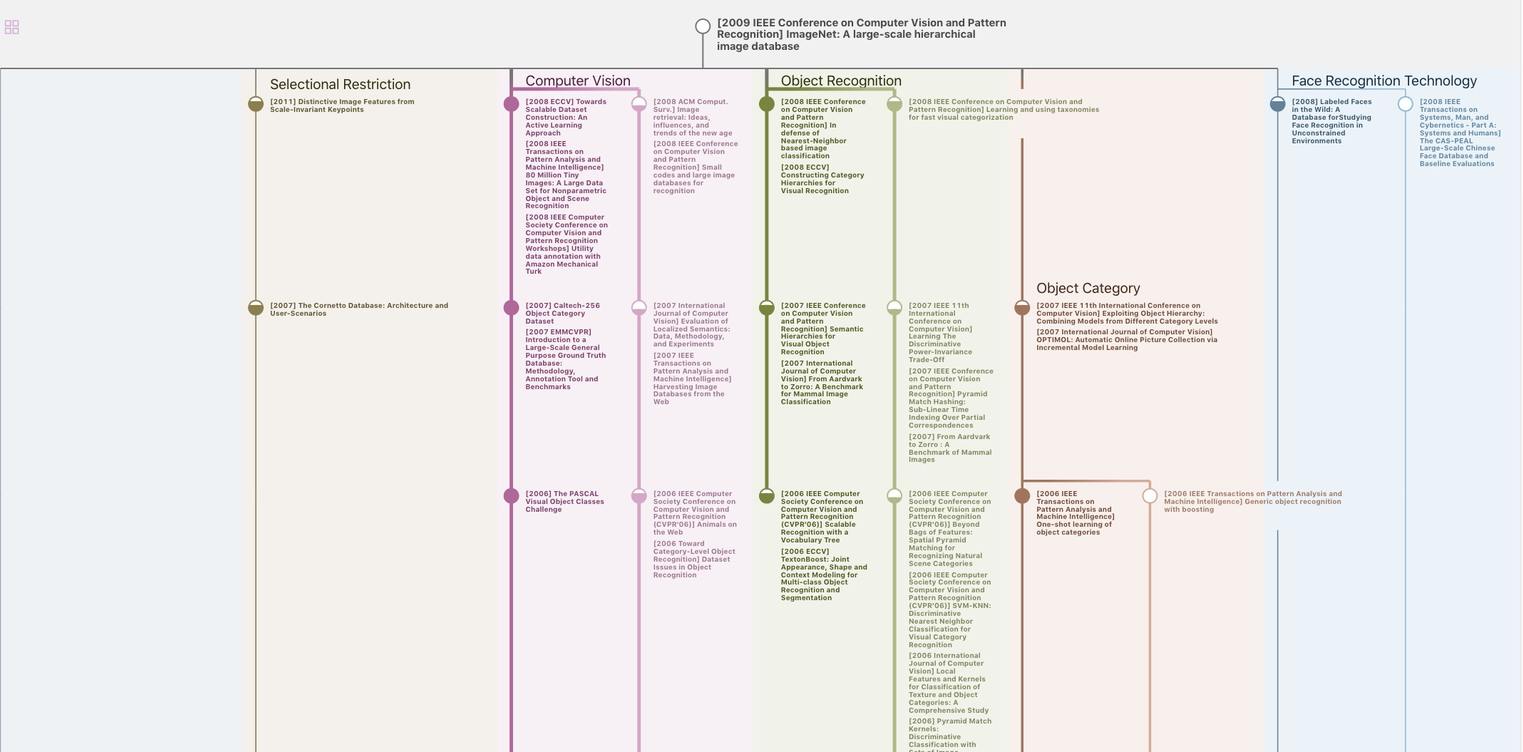
生成溯源树,研究论文发展脉络
Chat Paper
正在生成论文摘要