Fast Proximal Gradient Methods for Nonsmooth Convex Optimization for Tomographic Image Reconstruction
SENSING AND IMAGING(2020)
摘要
The Fast Proximal Gradient Method (FPGM) and the Monotone FPGM (MFPGM) for minimization of nonsmooth convex functions are introduced and applied to tomographic image reconstruction. Convergence properties of the sequence of objective function values are derived, including a O( 1/k^2) non-asymptotic bound. The presented theory broadens current knowledge and explains the convergence behavior of certain methods that are known to present good practical performance. Numerical experimentation involving computerized tomography image reconstruction shows the methods to be competitive in practical scenarios. Experimental comparison with Algebraic Reconstruction Techniques are performed uncovering certain behaviors of accelerated Proximal Gradient algorithms that apparently have not yet been noticed when these are applied to tomographic image reconstruction.
更多查看译文
关键词
Computerized tomography imaging, Convex optimization, Proximal gradient methods, Iterative algorithms
AI 理解论文
溯源树
样例
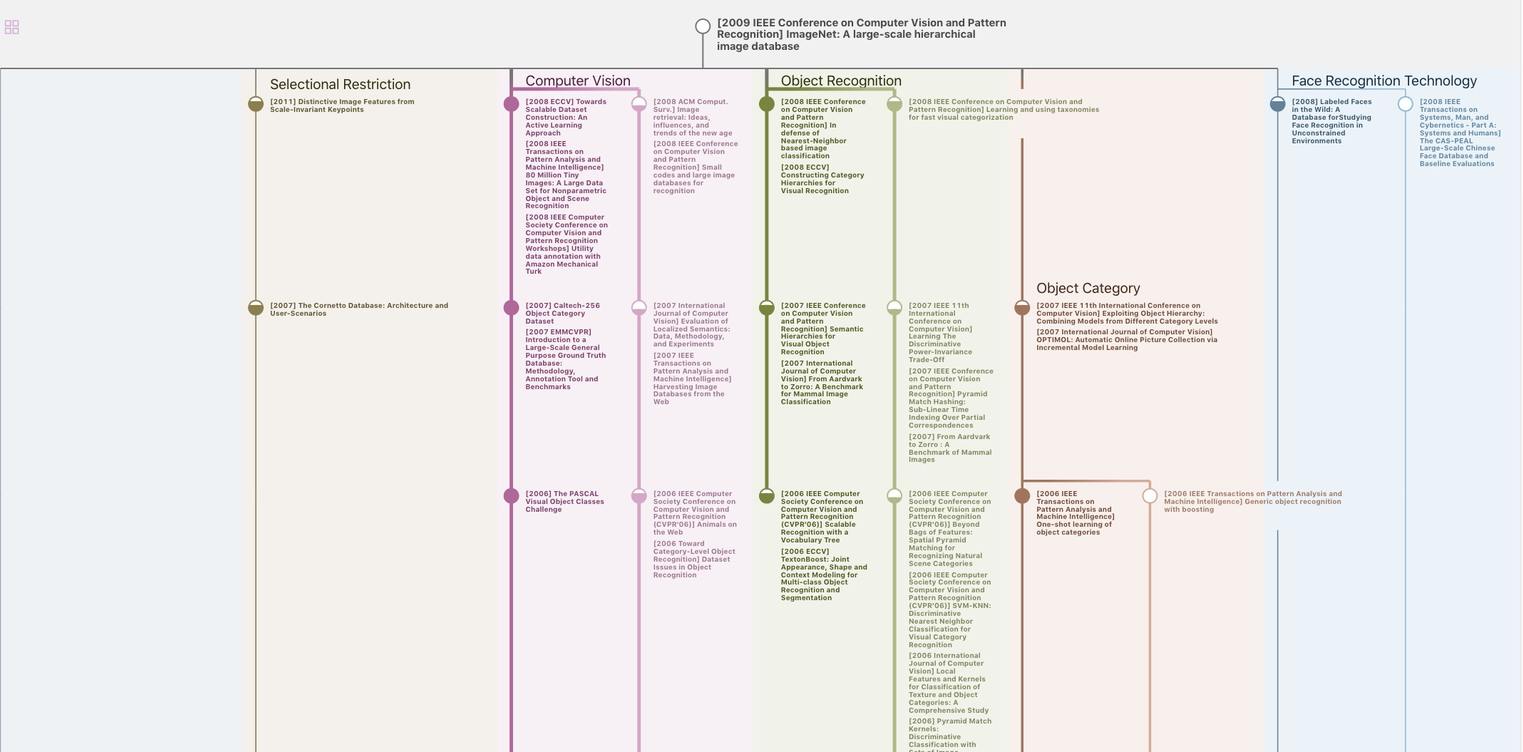
生成溯源树,研究论文发展脉络
Chat Paper
正在生成论文摘要