Reliable Detection of Causal Influences in Dynamical Systems
arxiv(2020)
摘要
Knowledge about existence, strength, and dominant direction of causal influences is of paramount importance for understanding complex systems. With limited amounts of realistic data, however, current methods for investigating causal links among different observables from dynamical systems suffer from ambiguous results. Missing is a statistically well defined approach that avoids false positive detections while being sensitive for weak interactions. Ideally, it should be able to infer directed causal influences also when synchronizations occur. The proposed method exploits local inflations of manifolds to obtain estimates of upper bounds on the information loss among state reconstructions from two observables. It comes with a test for the absence of causal influences. Simulated data demonstrate that it is robust to intrinsic noise, copes with synchronizations, and tolerates also measurement noise.
更多查看译文
关键词
causal influences,dynamical systems,detection
AI 理解论文
溯源树
样例
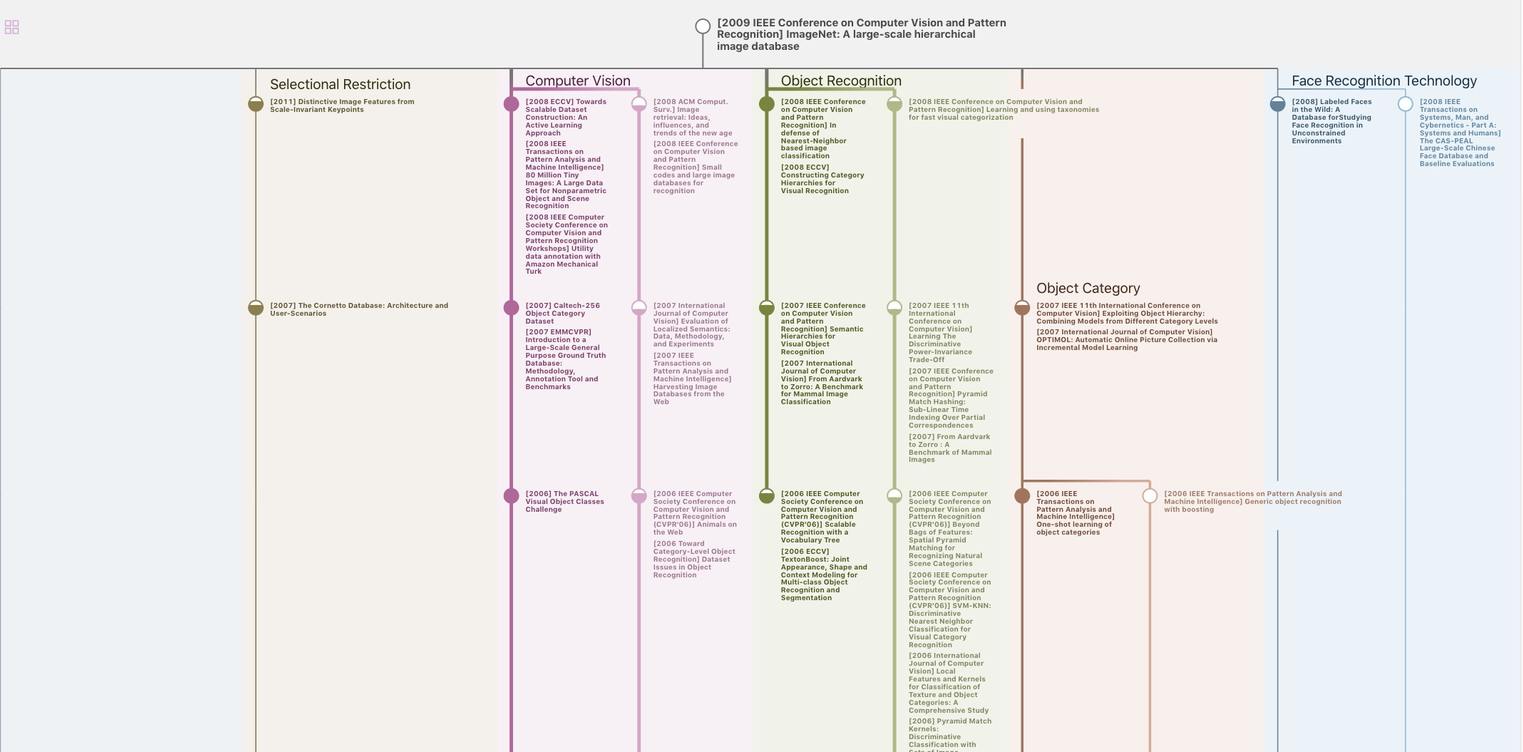
生成溯源树,研究论文发展脉络
Chat Paper
正在生成论文摘要