Conditional Entropy Coding for Efficient Video Compression
European Conference on Computer Vision(2020)
摘要
We propose a very simple and efficient video compression framework that only focuses on modeling the conditional entropy between frames. Unlike prior learning-based approaches, we reduce complexity by not performing any form of explicit transformations between frames and assume each frame is encoded with an independent state-of-the-art deep image compressor. We first show that a simple architecture modeling the entropy between the image latent codes is as competitive as other neural video compression works and video codecs while being much faster and easier to implement. We then propose a novel internal learning extension on top of this architecture that brings an additional \(\sim \)10% bitrate savings without trading off decoding speed. Importantly, we show that our approach outperforms H.265 and other deep learning baselines in MS-SSIM on higher bitrate UVG video, and against all video codecs on lower framerates, while being thousands of times faster in decoding than deep models utilizing an autoregressive entropy model.
更多查看译文
关键词
video
AI 理解论文
溯源树
样例
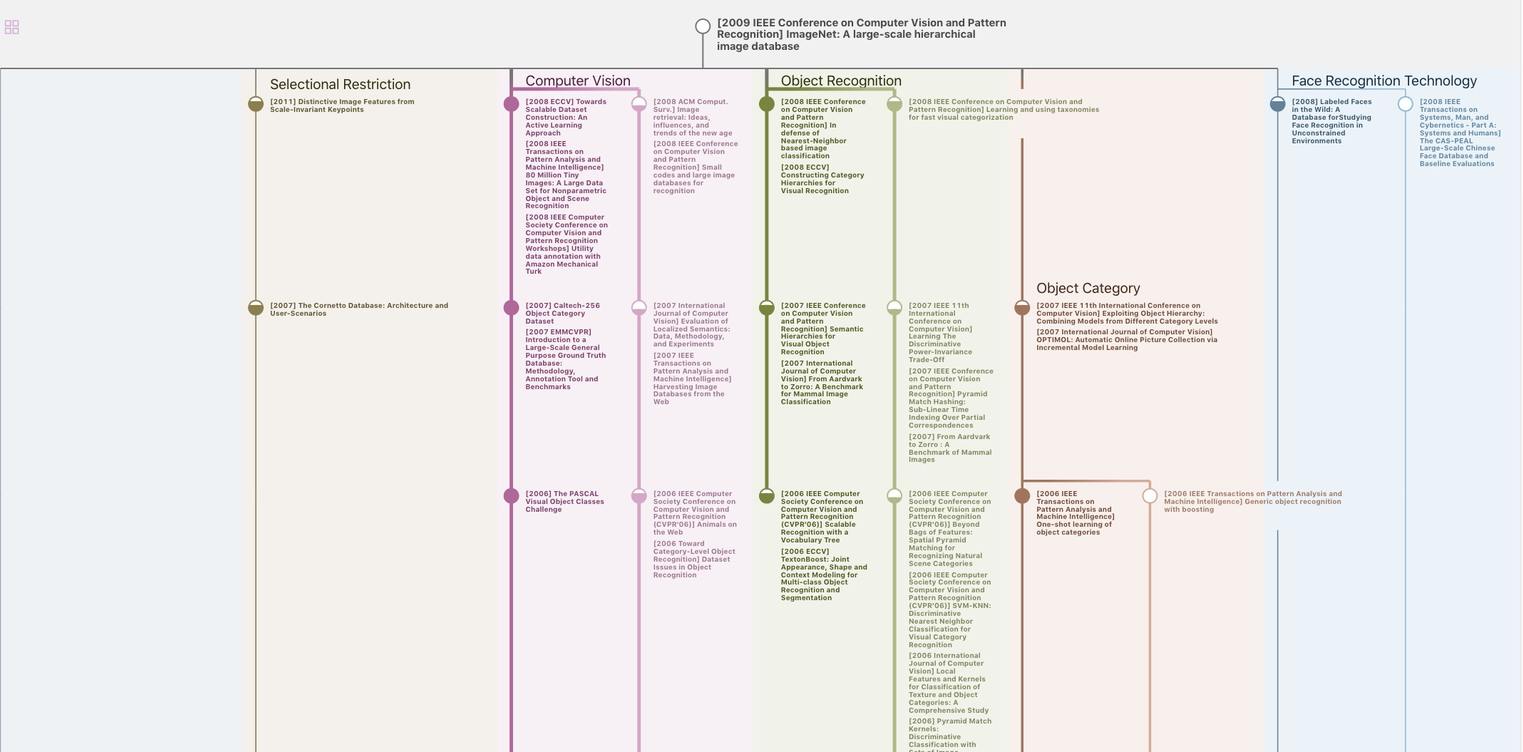
生成溯源树,研究论文发展脉络
Chat Paper
正在生成论文摘要