Using machine learning to predict clinical outcomes after shoulder arthroplasty with a minimal feature set
Journal of Shoulder and Elbow Surgery(2021)
摘要
Background: A machine learning analysis was conducted on 5774 shoulder arthroplasty patients to create predictive models for multiple clinical outcome measures after anatomic total shoulder arthroplasty (aTSA) and reverse total shoulder arthroplasty (rTSA). The goal of this study was to compare the accuracy associated with a full feature set predictive model (ie, full model, comprising 291 parameters) and a minimal feature set model (ie, abbreviated model, comprising 19 input parameters) to predict clinical outcomes to assess the efficacy of using a minimal feature set of inputs as a shoulder arthroplasty clinical decision-support tool.Methods: Clinical data from 2153 primary aTSA patients and 3621 primary rTSA patients were analyzed using the XGBoost machine learning technique to create and test predictive models for multiple outcome measures at different postoperative time points via the full and abbreviated models. Mean absolute errors (MAEs) quantified the difference between actual and predicted outcomes, and each model also predicted whether a patient would experience clinical improvement greater than the patient satisfaction anchor-based thresholds of the minimal clinically important difference and substantial clinical benefit for each outcome measure at 2-3 years after surgery.Results: Across all postoperative time points analyzed, the full and abbreviated models had similar MAEs for the American Shoulder and Elbow Surgeons score (+/- 11.7 with full model vs. +/- 12.0 with abbreviated model), Constant score (+8.9 vs. +/- 9.8), Global Shoulder Function score (+/- 1.4 vs. +/- 1.5), visual analog scale pain score (+/- 1.3 vs. +/- 1.4), active abduction (+/- 20.4 degrees - vs. +/- 21.8 degrees), forward elevation (+/- 17.6 degrees vs. +/- 19.2 degrees), and external rotation (+/- 12.2 degrees vs. +/- 12.6 degrees). Marginal improvements in MAEs were observed for each outcome measure prediction when the abbreviated model was supplemented with data on implant size and/or type and measurements of native glenoid anatomy. The full and abbreviated models each effectively risk stratified patients using only preoperative data by accurately identifying patients with improvement greater than the minimal clinically important difference and substantial clinical benefit thresholds.Discussion: Our study showed that the full and abbreviated machine learning models achieved similar accuracy in predicting clinical outcomes after aTSA and rTSA at multiple postoperative time points. These promising results demonstrate an efficient utilization of machine learning algorithms to predict clinical outcomes. Our findings using a minimal feature set of only 19 preoperative inputs suggest that this tool may be easily used during a surgical consultation to improve decision making related to shoulder arthroplasty. (C) 2020 Journal of Shoulder and Elbow Surgery Board of Trustees. All rights reserved.
更多查看译文
关键词
Basic Science Study,Computer Modeling
AI 理解论文
溯源树
样例
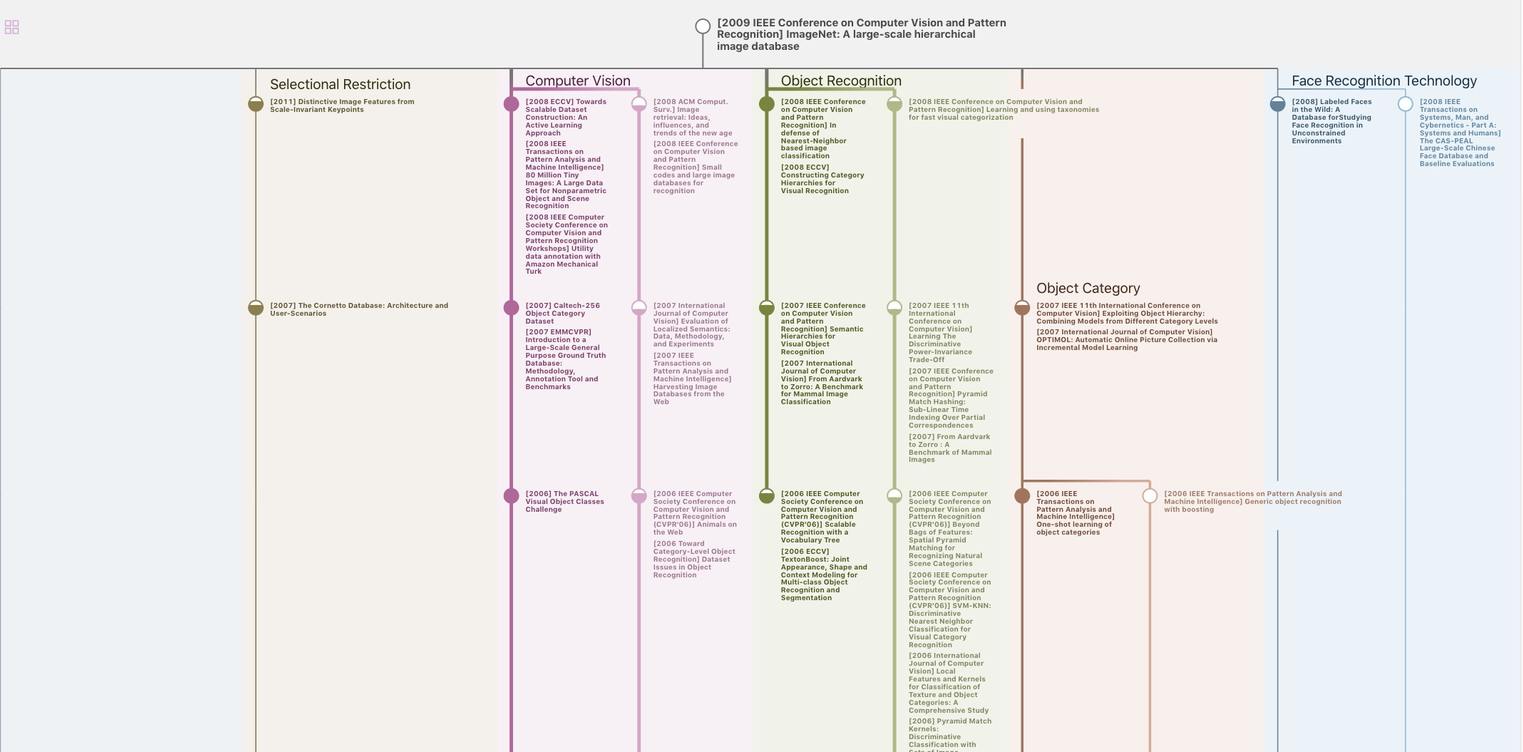
生成溯源树,研究论文发展脉络
Chat Paper
正在生成论文摘要