Frank-Wolfe algorithm for learning SVM-type multi-category classifiers.
SDM(2021)
摘要
Multi-category support vector machine (MC-SVM) is one of the most popular machine learning algorithms. There are lots of variants of MC-SVM, although different optimization algorithms were developed for different learning machines. In this study, we developed a new optimization algorithm that can be applied to many of MC-SVM variants. The algorithm is based on the Frank-Wolfe framework that requires two subproblems, direction finding and line search, in each iteration. The contribution of this study is the discovery that both subproblems have a closed form solution if the Frank-Wolfe framework is applied to the dual problem. Additionally, the closed form solutions on both for the direction finding and for the line search exist even for the Moreau envelopes of the loss functions. We use several large datasets to demonstrate that the proposed optimization algorithm converges rapidly and thereby improves the pattern recognition performance.
更多查看译文
关键词
SVM, convex optimization, Frank-Wolfe, dual problem, multi-category classification
AI 理解论文
溯源树
样例
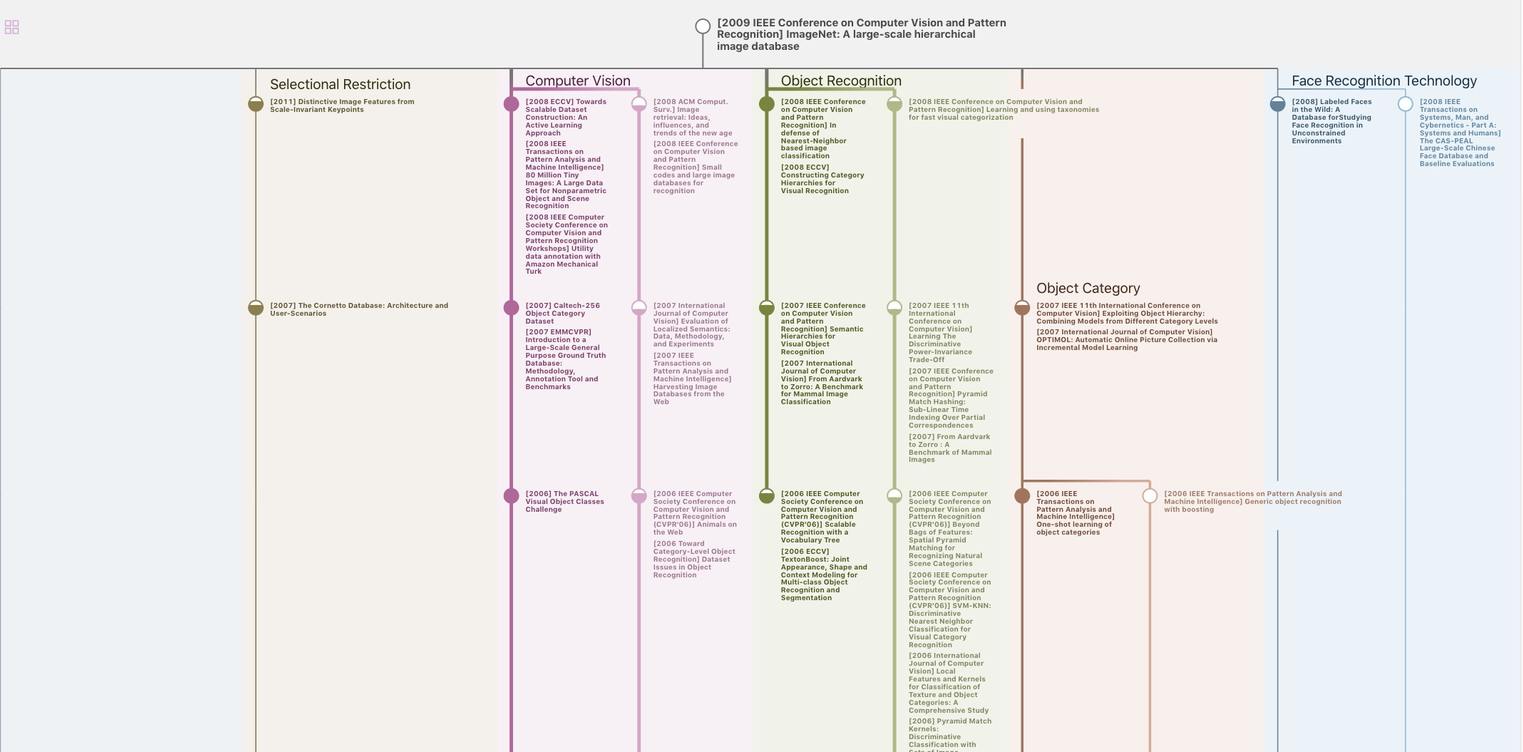
生成溯源树,研究论文发展脉络
Chat Paper
正在生成论文摘要