Demand Forecasting using Long Short-Term Memory Neural Networks
arxiv(2020)
摘要
In this paper we investigate to what extent long short-term memory neural networks (LSTMs) are suitable for demand forecasting in the e-grocery retail sector. For this purpose, univariate as well as multivariate LSTM-based models were developed and tested for 100 fast-moving consumer goods in the context of a master's thesis. On average, the developed models showed better results for food products than the comparative models from both statistical and machine learning families. Solely in the area of beverages random forest and linear regression achieved slightly better results. This outcome suggests that LSTMs can be used for demand forecasting at product level. The performance of the models presented here goes beyond the current state of research, as can be seen from the evaluations based on a data set that unfortunately has not been publicly available to date.
更多查看译文
关键词
neural networks,demand,memory,short-term
AI 理解论文
溯源树
样例
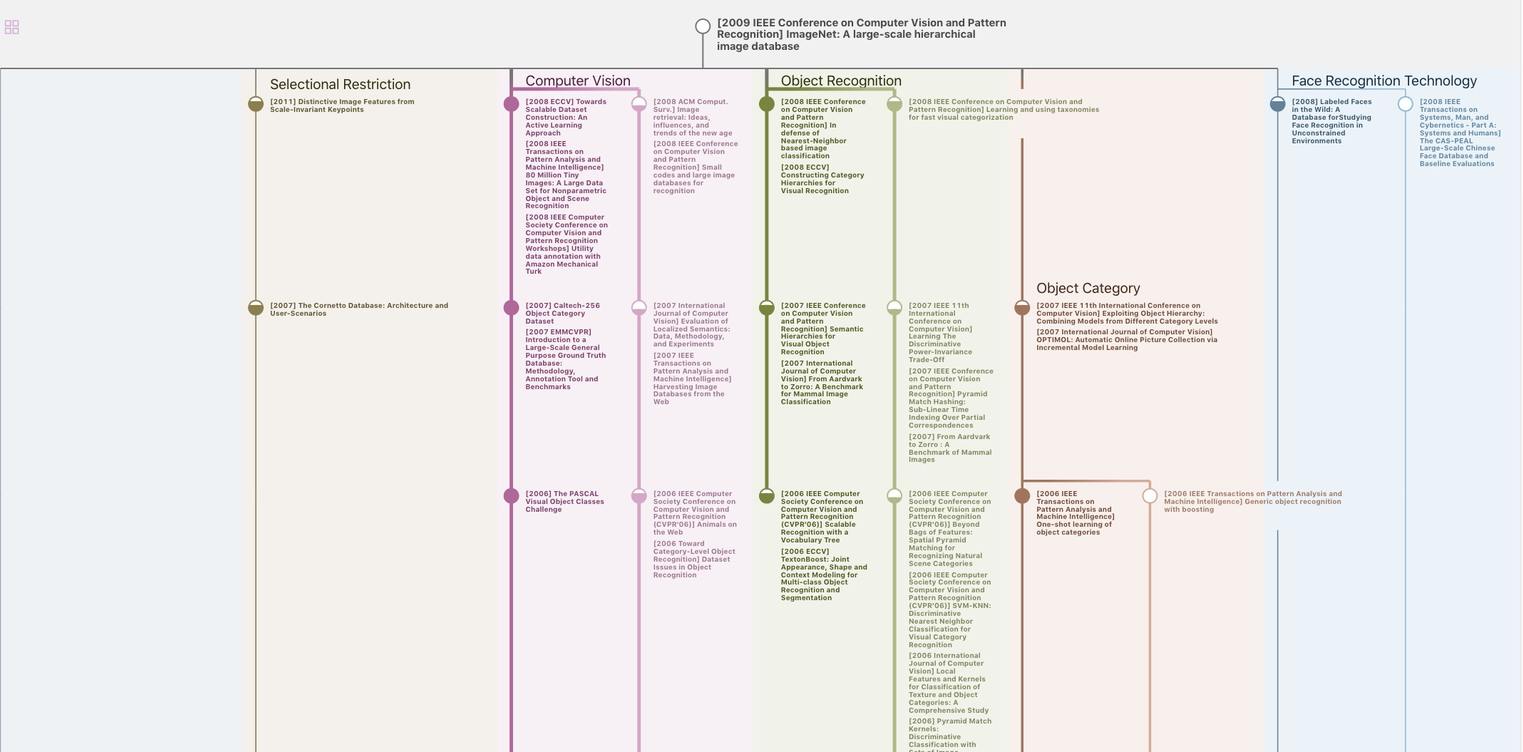
生成溯源树,研究论文发展脉络
Chat Paper
正在生成论文摘要