DIR-DBTnet: Deep iterative reconstruction network for 3D digital breast tomosynthesis imaging
arxiv(2020)
摘要
Purpose: The goal of this study is to develop a novel deep learning (DL) based reconstruction framework to improve the digital breast tomosynthesis (DBT) imaging performance. Methods: In this work, the DIR-DBTnet is developed for DBT image reconstruction by unrolling the standard iterative reconstruction algorithm within the deep learning framework. In particular, such network learns the regularizer and the iteration parameters automatically through network training with a large amount of simulated DBT data. Afterwards, both numerical and experimental data are used to evaluate its performance. Quantitative metrics such as the artifact spread function (ASF), breast density, and the signal difference to noise ratio (SDNR) are used for image quality assessment. Results: For both numerical and experimental data, the proposed DIR-DBTnet generates reduced in-plane shadow artifacts and out-of-plane artifacts compared with the filtered back projection (FBP) and total variation (TV) methods. Quantitatively, the full width half maximum (FWHM) of the measured ASF curve from the numerical data is 33.4% and 19.7% smaller than those obtained with the FBP and TV methods, respectively; the breast density of the network reconstructed DBT images is more accurate and consistent with the ground truth. Conclusions: In conclusion, a deep iterative reconstruction network, DIR-DBTnet, has been proposed. Both qualitative and quantitative analyses of the numerical and experimental results show superior DBT imaging performance than the FBP and iterative algorithms.
更多查看译文
AI 理解论文
溯源树
样例
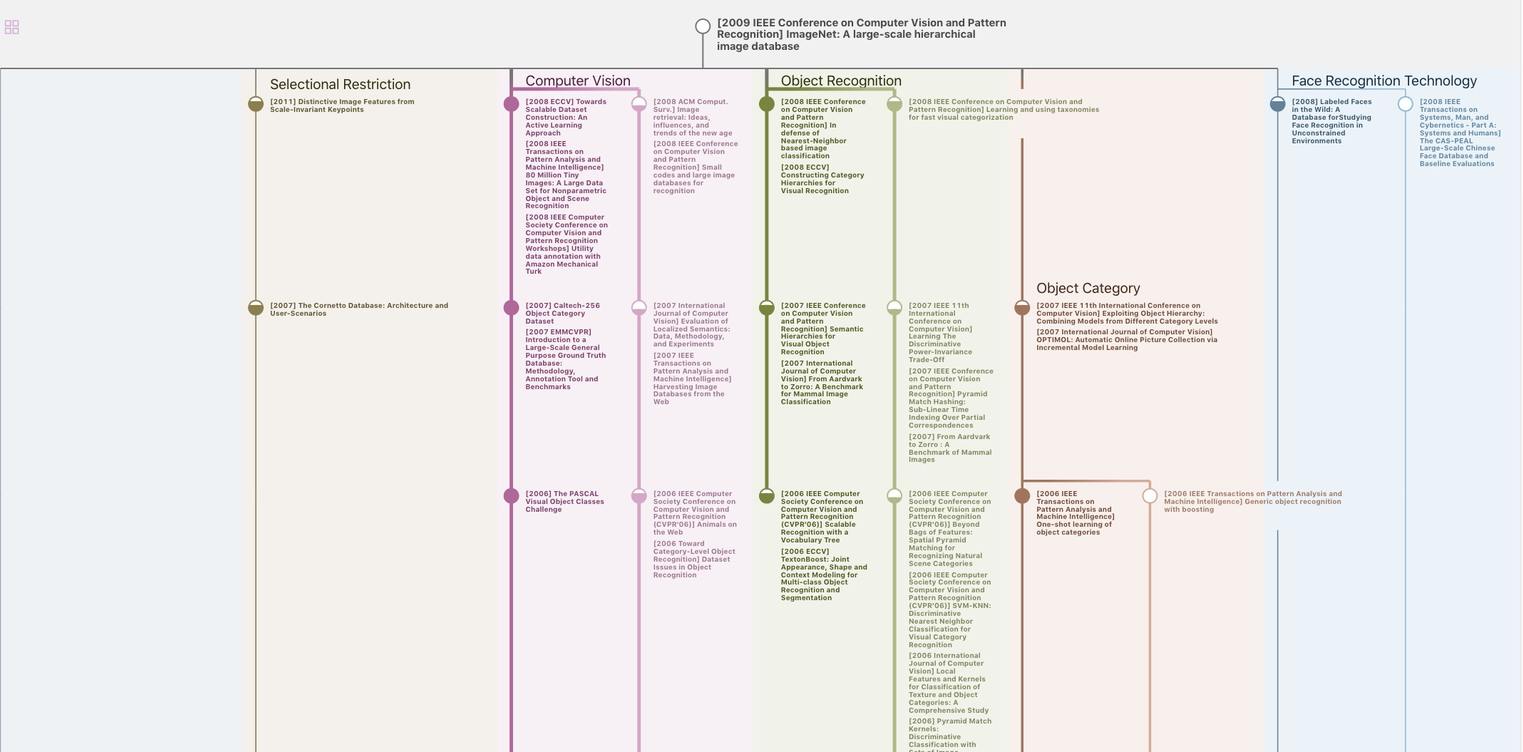
生成溯源树,研究论文发展脉络
Chat Paper
正在生成论文摘要