Unraveling Representations in Scene-selective Brain Regions Using Scene-Parsing Deep Neural Networks
bioRxiv(2021)
摘要
Visual scene perception is mediated by a set of cortical regions that respond preferentially to images of scenes, including the occipital place area (OPA) and parahippocampal place area (PPA). However, the differential contribution of OPA and PPA to scene perception remains an open research question. In this study, we take a deep neural network (DNN)-based computational approach to investigate the differences in OPA and PPA function. In a first step, we search for a computational model that predicts fMRI responses to scenes in OPA and PPA well. We find that DNNs trained to predict scene components (e.g., wall, ceiling, floor) explain higher variance uniquely in OPA and PPA than a DNN trained to predict scene category (e.g., bathroom, kitchen, office). This result is robust across several DNN architectures. On this basis, we then determine whether particular scene components predicted by DNNs differentially account for unique variance in OPA and PPA. We find that variance in OPA responses uniquely explained by the navigation-related floor component is higher compared to the variance explained by the wall and ceiling components. In contrast, PPA responses are better explained by the combination of wall and floor, that is, scene components that together contain the structure and texture of the scene. This differential sensitivity to scene components suggests differential functions of OPA and PPA in scene processing. Moreover, our results further highlight the potential of the proposed computational approach as a general tool in the investigation of the neural basis of human scene perception.
更多查看译文
关键词
Occipital Place Area,Parahippocampal Place Area,Deep Neural Networks,Functional Magnetic Resonance Imaging,Navigational Affordance model,Representational Similarity Analysis,Scene parsing
AI 理解论文
溯源树
样例
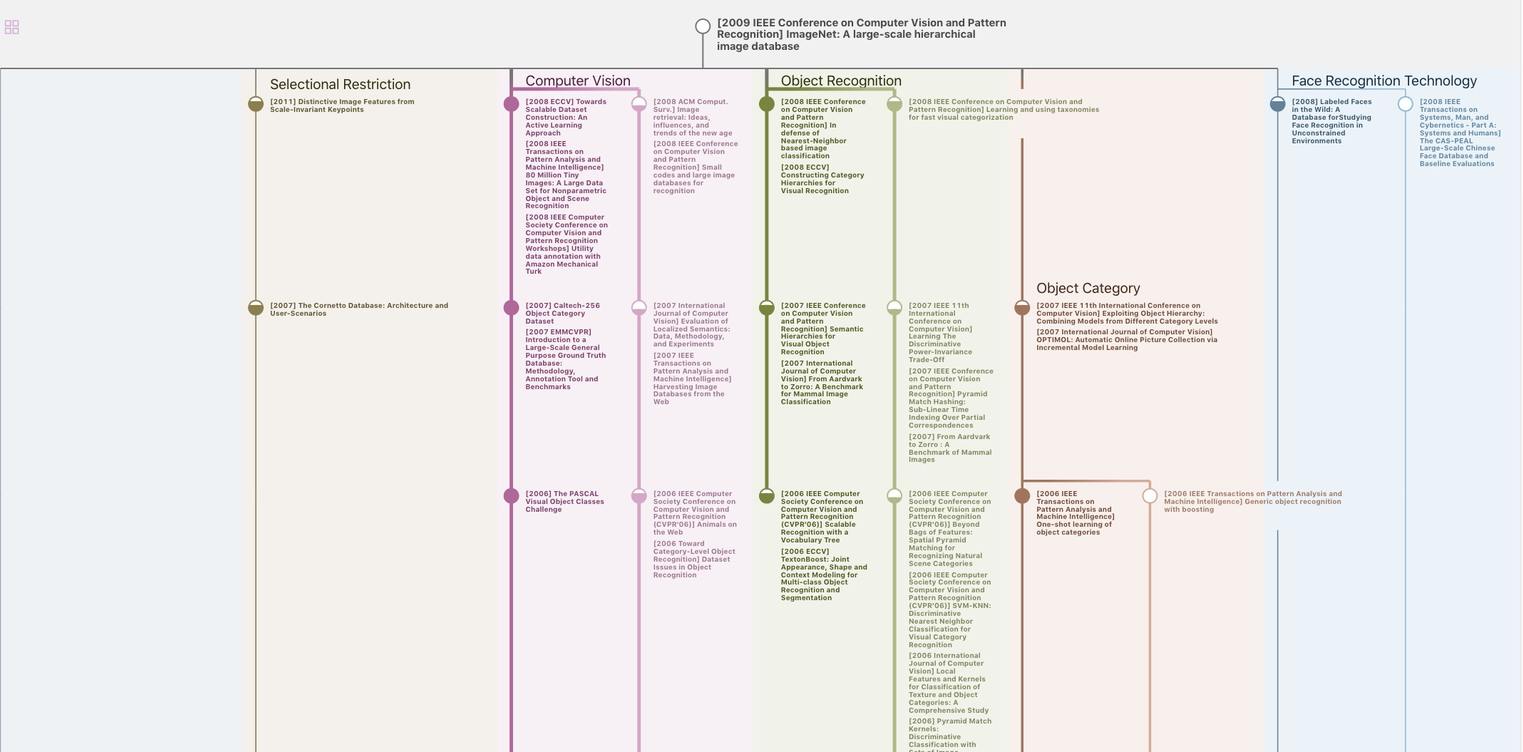
生成溯源树,研究论文发展脉络
Chat Paper
正在生成论文摘要