Personalized Deep Learning for Ventricular Arrhythmias Detection on Medical loT Systems
arxiv(2020)
摘要
Life-threatening ventricular arrhythmias (VA) are the leading cause of sudden cardiac death (SCD), which is the most significant cause of natural death in the US [6]. The implantable cardioverter defibrillator (ICD) is a small device implanted to patients under high risk of SCD as a preventive treatment. The ICD continuously monitors the intracardiac rhythm and delivers shock when detecting the life-threatening VA. Traditional methods detect VA by setting criteria on the detected rhythm. However, those methods suffer from a high inappropriate shock rate and require a regular follow-up to optimize criteria parameters for each ICD recipient. To ameliorate the challenges, we propose the personalized computing framework for deep learning based VA detection on medical IoT systems. The system consists of intracardiac and surface rhythm monitors, and the cloud platform for data uploading, diagnosis, and CNN model personalization. We equip the system with real-time inference on both intracardiac and surface rhythm monitors. To improve the detection accuracy, we enable the monitors to detect VA collaboratively by proposing the cooperative inference. We also introduce the CNN personalization for each patient based on the computing framework to tackle the unlabeled and limited rhythm data problem. When compared with the traditional detection algorithm, the proposed method achieves comparable accuracy on VA rhythm detection and 6.6% reduction in inappropriate shock rate, while the average inference latency is kept at 71ms.
更多查看译文
关键词
intracardiac rhythm,implantable cardioverter defibrillator,sudden cardiac death,ventricular arrhythmias,medical loT systems,personalized deep learning,CNN model personalization,surface rhythm monitors,medical IoT systems
AI 理解论文
溯源树
样例
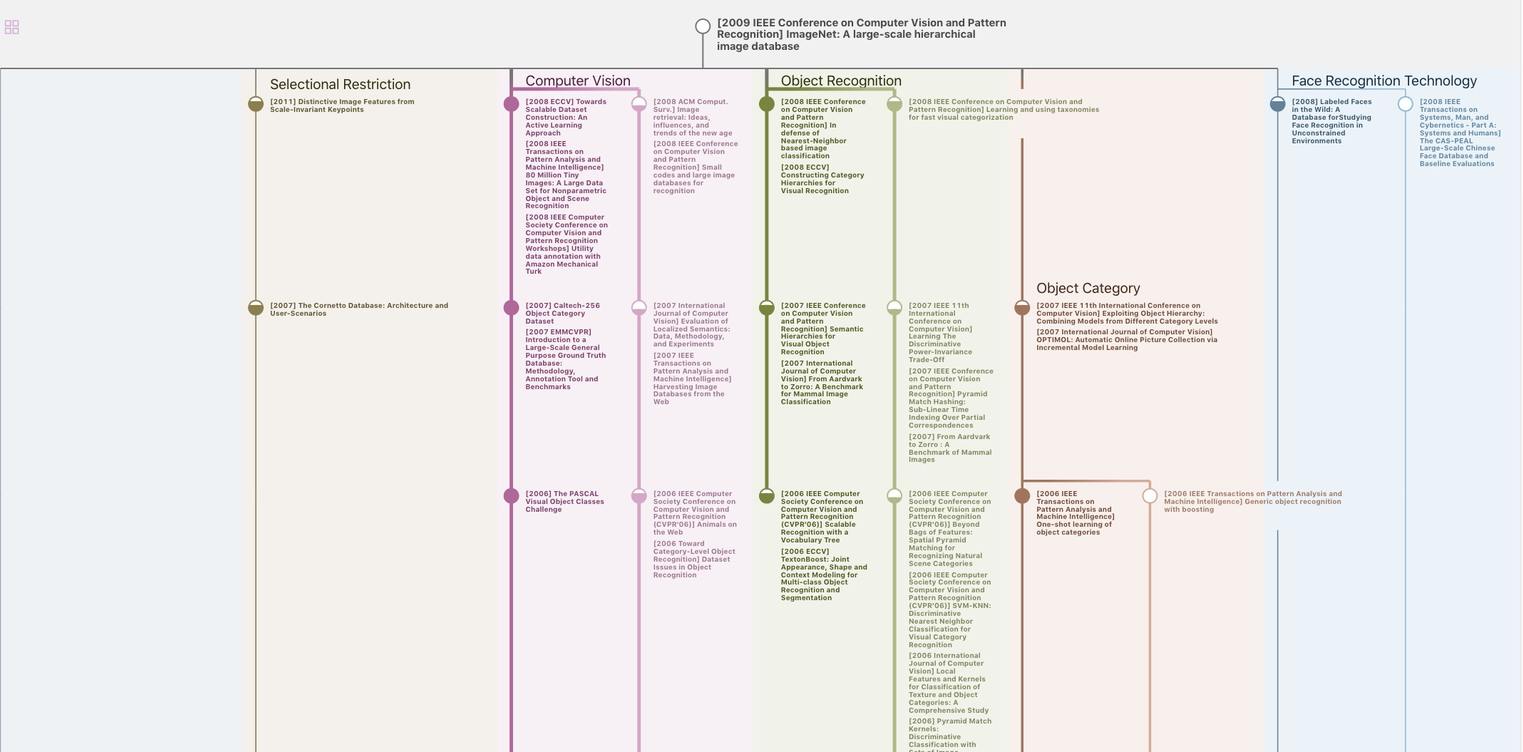
生成溯源树,研究论文发展脉络
Chat Paper
正在生成论文摘要