A digital biomarker of diabetes from smartphone-based vascular signals
NATURE MEDICINE(2020)
摘要
The global burden of diabetes is rapidly increasing, from 451 million people in 2019 to 693 million by 2045 1 . The insidious onset of type 2 diabetes delays diagnosis and increases morbidity 2 . Given the multifactorial vascular effects of diabetes, we hypothesized that smartphone-based photoplethysmography could provide a widely accessible digital biomarker for diabetes. Here we developed a deep neural network (DNN) to detect prevalent diabetes using smartphone-based photoplethysmography from an initial cohort of 53,870 individuals (the ‘primary cohort’), which we then validated in a separate cohort of 7,806 individuals (the ‘contemporary cohort’) and a cohort of 181 prospectively enrolled individuals from three clinics (the ‘clinic cohort’). The DNN achieved an area under the curve for prevalent diabetes of 0.766 in the primary cohort (95% confidence interval: 0.750–0.782; sensitivity 75%, specificity 65%) and 0.740 in the contemporary cohort (95% confidence interval: 0.723–0.758; sensitivity 81%, specificity 54%). When the output of the DNN, called the DNN score, was included in a regression analysis alongside age, gender, race/ethnicity and body mass index, the area under the curve was 0.830 and the DNN score remained independently predictive of diabetes. The performance of the DNN in the clinic cohort was similar to that in other validation datasets. There was a significant and positive association between the continuous DNN score and hemoglobin A1c ( P ≤ 0.001) among those with hemoglobin A1c data. These findings demonstrate that smartphone-based photoplethysmography provides a readily attainable, non-invasive digital biomarker of prevalent diabetes.
更多查看译文
关键词
Diabetes,Predictive markers,Biomedicine,general,Cancer Research,Metabolic Diseases,Infectious Diseases,Molecular Medicine,Neurosciences
AI 理解论文
溯源树
样例
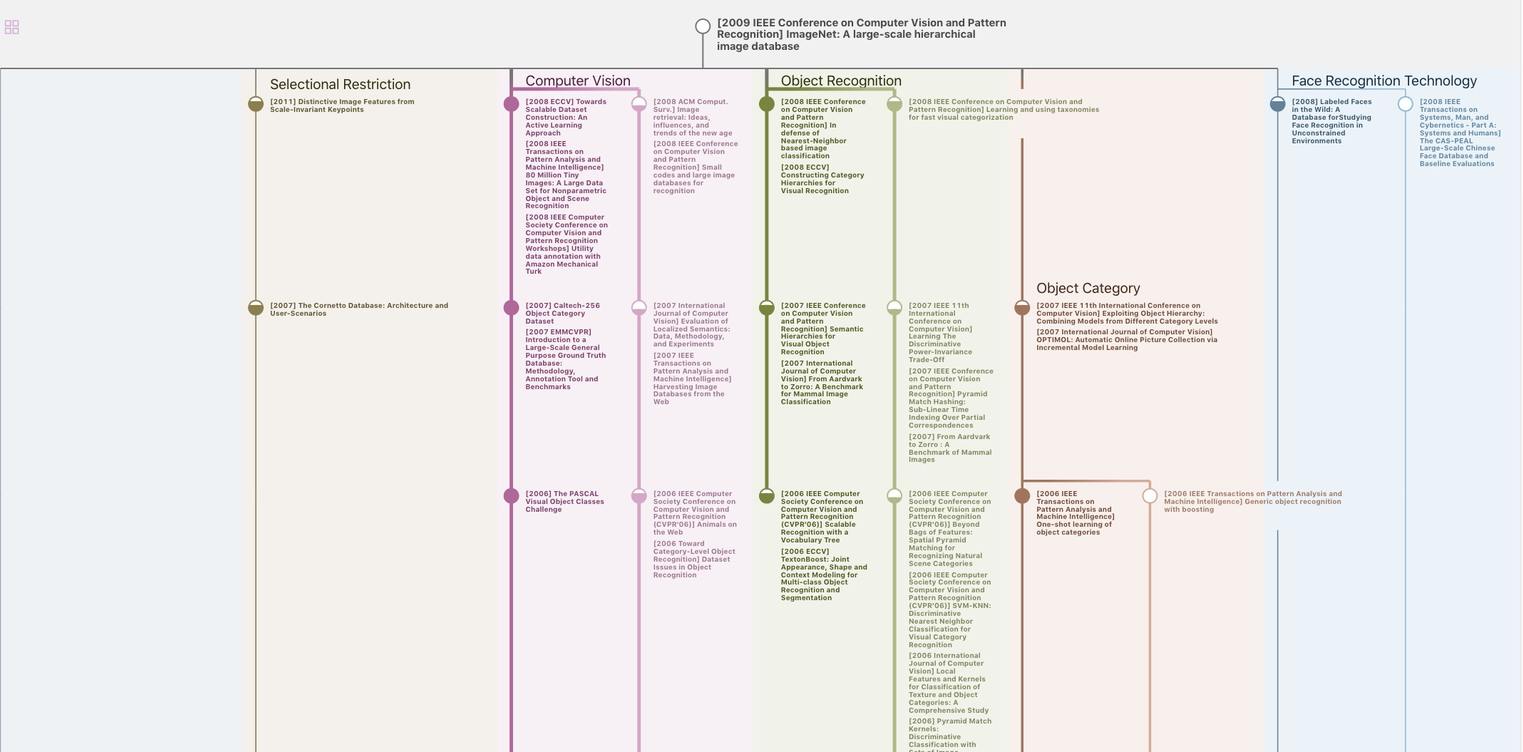
生成溯源树,研究论文发展脉络
Chat Paper
正在生成论文摘要