SoftPoolNet: Shape Descriptor for Point Cloud Completion and Classification
European Conference on Computer Vision(2020)
摘要
Point clouds are often the default choice for many applications as they exhibit more flexibility and efficiency than volumetric data. Nevertheless, their unorganized nature – points are stored in an unordered way – makes them less suited to be processed by deep learning pipelines. In this paper, we propose a method for 3D object completion and classification based on point clouds. We introduce a new way of organizing the extracted features based on their activations, which we name soft pooling. For the decoder stage, we propose regional convolutions, a novel operator aimed at maximizing the global activation entropy. Furthermore, inspired by the local refining procedure in Point Completion Network (PCN), we also propose a patch-deforming operation to simulate deconvolutional operations for point clouds. This paper proves that our regional activation can be incorporated in many point cloud architectures like AtlasNet and PCN, leading to better performance for geometric completion. We evaluate our approach on different 3D tasks such as object completion and classification, achieving state-of-the-art accuracy.
更多查看译文
关键词
point cloud completion,shape descriptor,classification
AI 理解论文
溯源树
样例
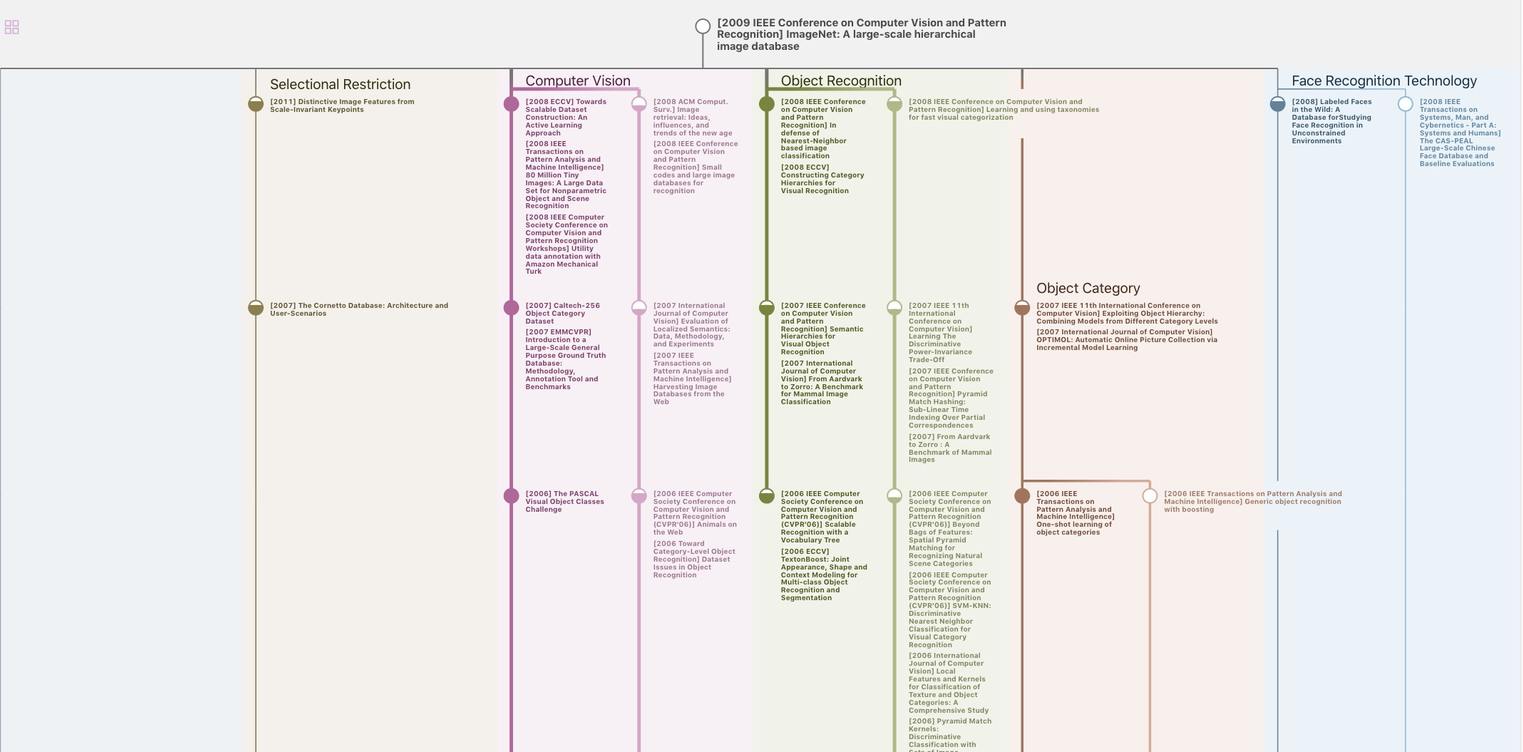
生成溯源树,研究论文发展脉络
Chat Paper
正在生成论文摘要