Bayesian Model Selection in Additive Partial Linear Models Via Locally Adaptive Splines
JOURNAL OF COMPUTATIONAL AND GRAPHICAL STATISTICS(2022)
摘要
We provide a flexible framework for selecting among a class of additive partial linear models that allows both linear and nonlinear additive components. In practice, it is challenging to determine which additive components should be excluded from the model while simultaneously determining whether nonzero additive components should be represented as linear or nonlinear components in the final model. In this article, we propose a Bayesian model selection method that is facilitated by a carefully specified class of models, including the choice of a prior distribution and the nonparametric model used for the nonlinear additive components. We employ a series of latent variables that determine the effect of each variable among the three possibilities (no effect, linear effect, and nonlinear effect) and that simultaneously determine the knots of each spline for a suitable penalization of smooth functions. The use of a pseudo-prior distribution along with a collapsing scheme enables us to deploy well-behaved Markov chain Monte Carlo samplers, both for model selection and for fitting the preferred model. Our method and algorithm are deployed on a suite of numerical studies and are applied to a nutritional epidemiology study. The numerical results show that the proposed methodology outperforms previously available methods in terms of effective sample sizes of the Markov chain samplers and the overall misclassification rates.
更多查看译文
关键词
Bayesian adaptive regression, Function estimation, Knot-selection, Mixtures of g-priors, Nonparametric regression
AI 理解论文
溯源树
样例
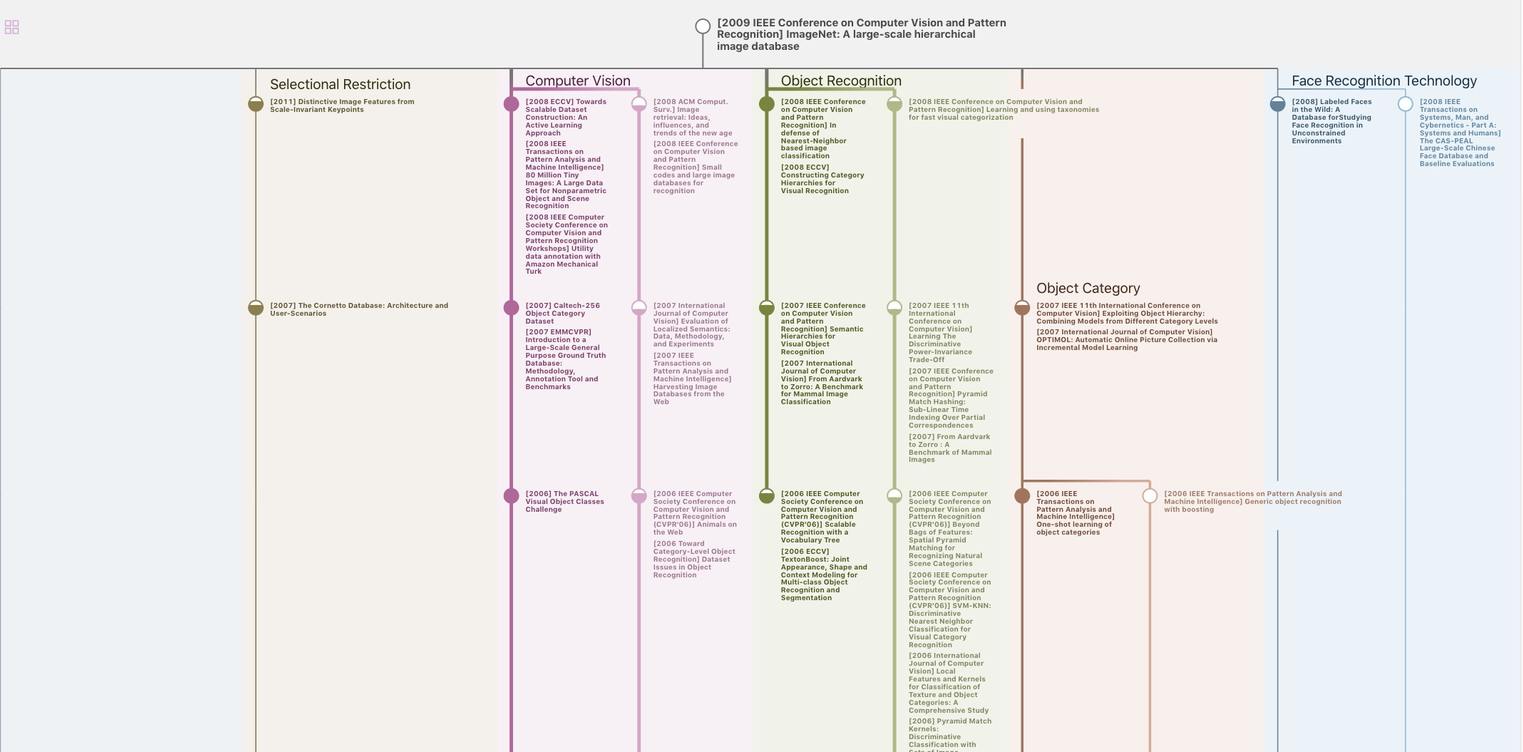
生成溯源树,研究论文发展脉络
Chat Paper
正在生成论文摘要