Improving deconvolution methods in biology through open innovation competitions: an application to the connectivity map
BIOINFORMATICS(2021)
摘要
Motivation: Do machine learning methods improve standard deconvolution techniques for gene expression data? This article uses a unique new dataset combined with an open innovation competition to evaluate a wide range of approaches developed by 294 competitors from 20 countries. The competition's objective was to address a deconvolution problem critical to analyzing genetic perturbations from the Connectivity Map. The issue consists of separating gene expression of individual genes from raw measurements obtained from gene pairs. We evaluated the outcomes using ground-truth data (direct measurements for single genes) obtained from the same samples. Results: We find that the top-ranked algorithm, based on random forest regression, beat the other methods in accuracy and reproducibility; more traditional gaussian-mixture methods performed well and tended to be faster, and the best deep learning approach yielded outcomes slightly inferior to the above methods. We anticipate researchers in the field will find the dataset and algorithms developed in this study to be a powerful research tool for benchmarking their deconvolution methods and a resource useful for multiple applications.
更多查看译文
关键词
connectivity map,open innovation competition,crowdsourcing,deconvolution algorithm,machine learning,gene expression
AI 理解论文
溯源树
样例
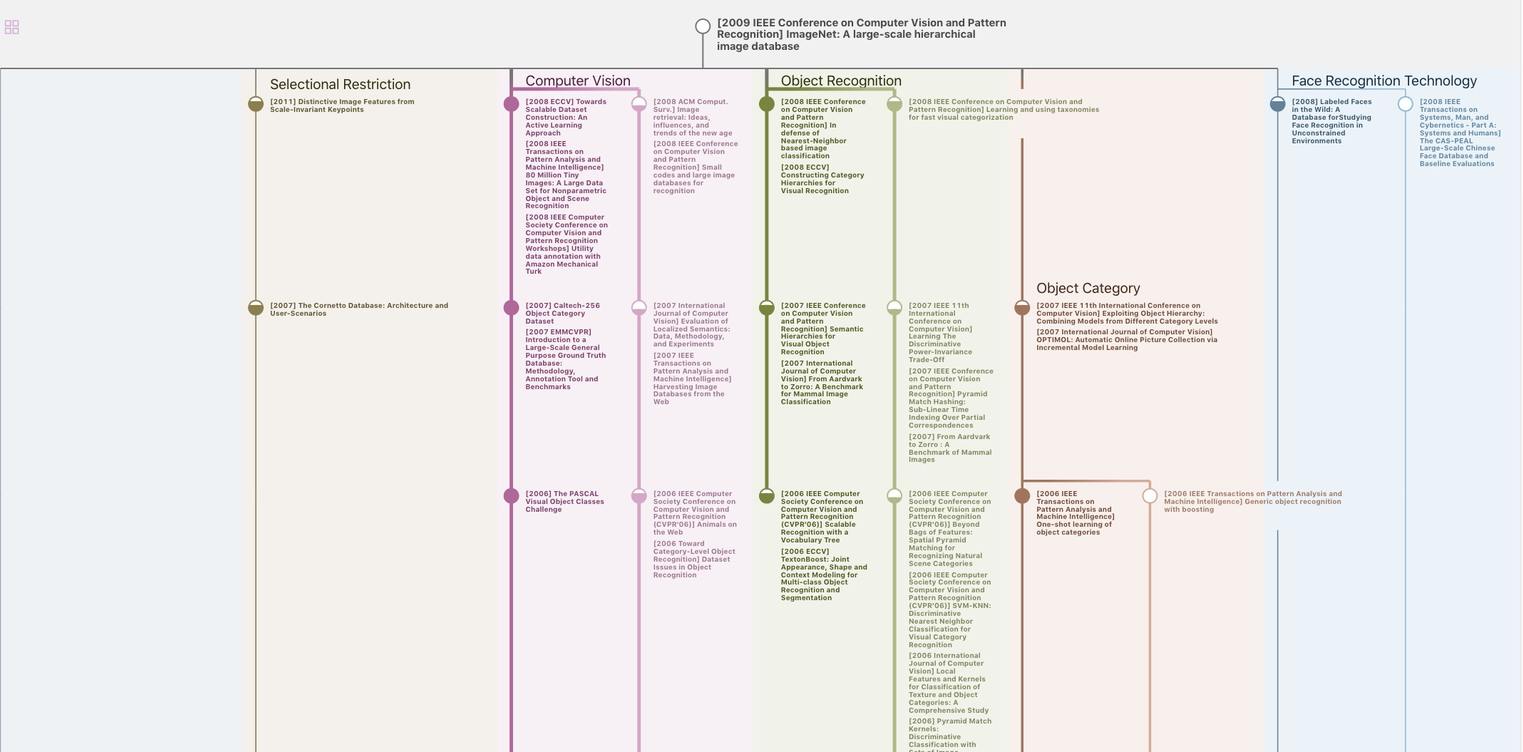
生成溯源树,研究论文发展脉络
Chat Paper
正在生成论文摘要