A Multiscale Self-Adaptive Attention Network for Remote Sensing Scene Classification.
REMOTE SENSING(2020)
摘要
High-resolution optical remote sensing image classification is an important research direction in the field of computer vision. It is difficult to extract the rich semantic information from remote sensing images with many objects. In this paper, a multiscale self-adaptive attention network (MSAA-Net) is proposed for the optical remote sensing image classification, which includes multiscale feature extraction, adaptive information fusion, and classification. In the first part, two parallel convolution blocks with different receptive fields are adopted to capture multiscale features. Then, the squeeze process is used to obtain global information and the excitation process is used to learn the weights in different channels, which can adaptively select useful information from multiscale features. Furthermore, the high-level features are classified by many residual blocks with an attention mechanism and a fully connected layer. Experiments were conducted using the UC Merced, NWPU, and the Google SIRI-WHU datasets. Compared to the state-of-the-art methods, the MSAA-Net has great effect and robustness, with average accuracies of 94.52%, 95.01%, and 95.21% on the three widely used remote sensing datasets.
更多查看译文
关键词
multiscale feature,feature fusion,scene classification,dual attention
AI 理解论文
溯源树
样例
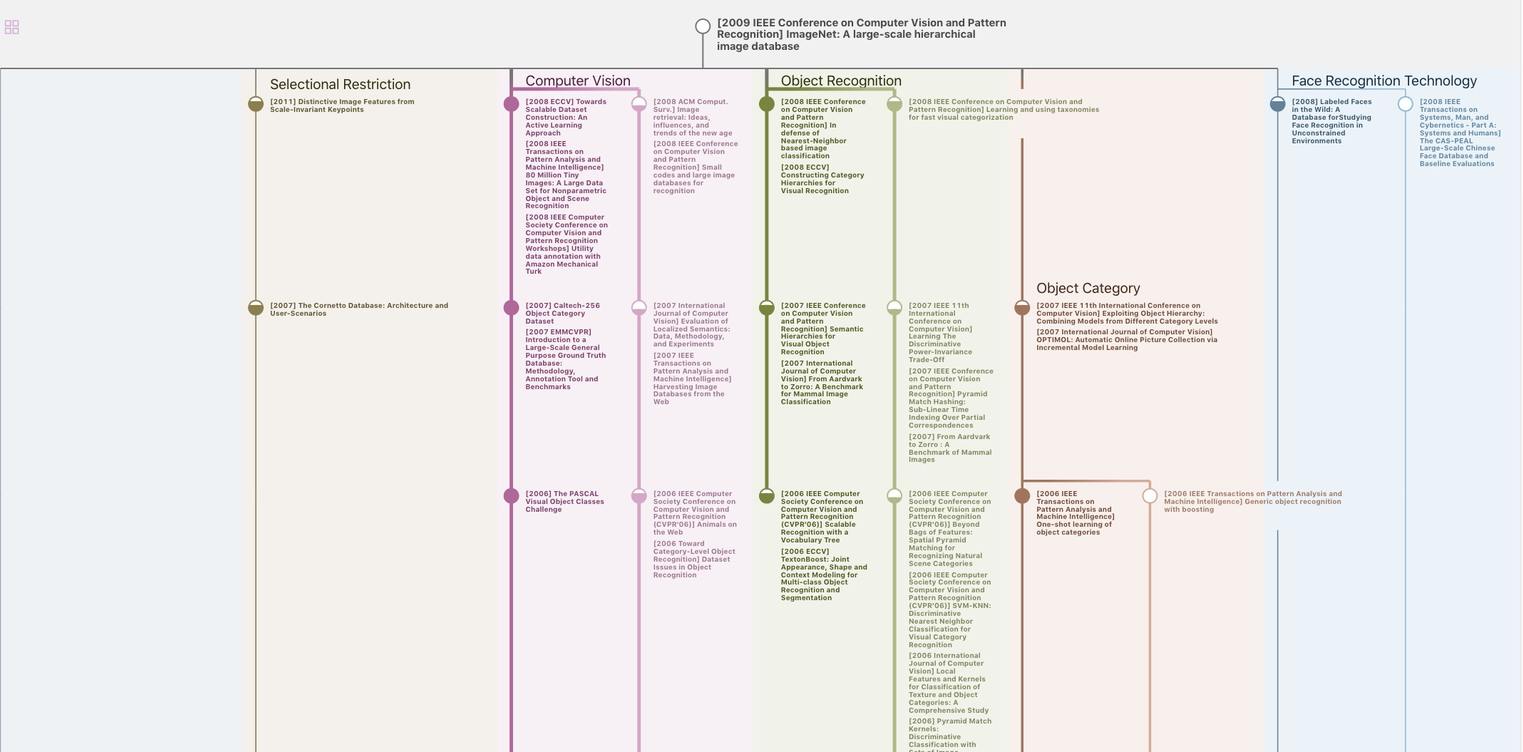
生成溯源树,研究论文发展脉络
Chat Paper
正在生成论文摘要