Capturing associations in graphs
PROCEEDINGS OF THE VLDB ENDOWMENT(2020)
摘要
This paper proposes a class of graph association rules, denoted by GARs, to specify regularities between entities in graphs. A GAR is a combination of a graph pattern and a dependency; it may take as predicates ML (machine learning) classifiers for link prediction. We show that GARs help us catch incomplete information in schemaless graphs, predict links in social graphs, identify potential customers in digital marketing, and extend graph functional dependencies (GFDs) to capture both missing links and inconsistencies. We formalize association deduction with GARs in terms of the chase, and prove its Church-Rosser property. We show that the satisfiability, implication and association deduction problems for GARs are coNP-complete, NP-complete and NP-complete, respectively, retaining the same complexity bounds as their GFD counterparts, despite the increased expressive power of GARs. The incremental deduction problem is DP-complete for GARs versus coNP-complete for GFDs. In addition, we provide parallel algorithms for association deduction and incremental deduction. Using real-life and synthetic graphs, we experimentally verify the effectiveness, scalability and efficiency of the parallel algorithms.
更多查看译文
AI 理解论文
溯源树
样例
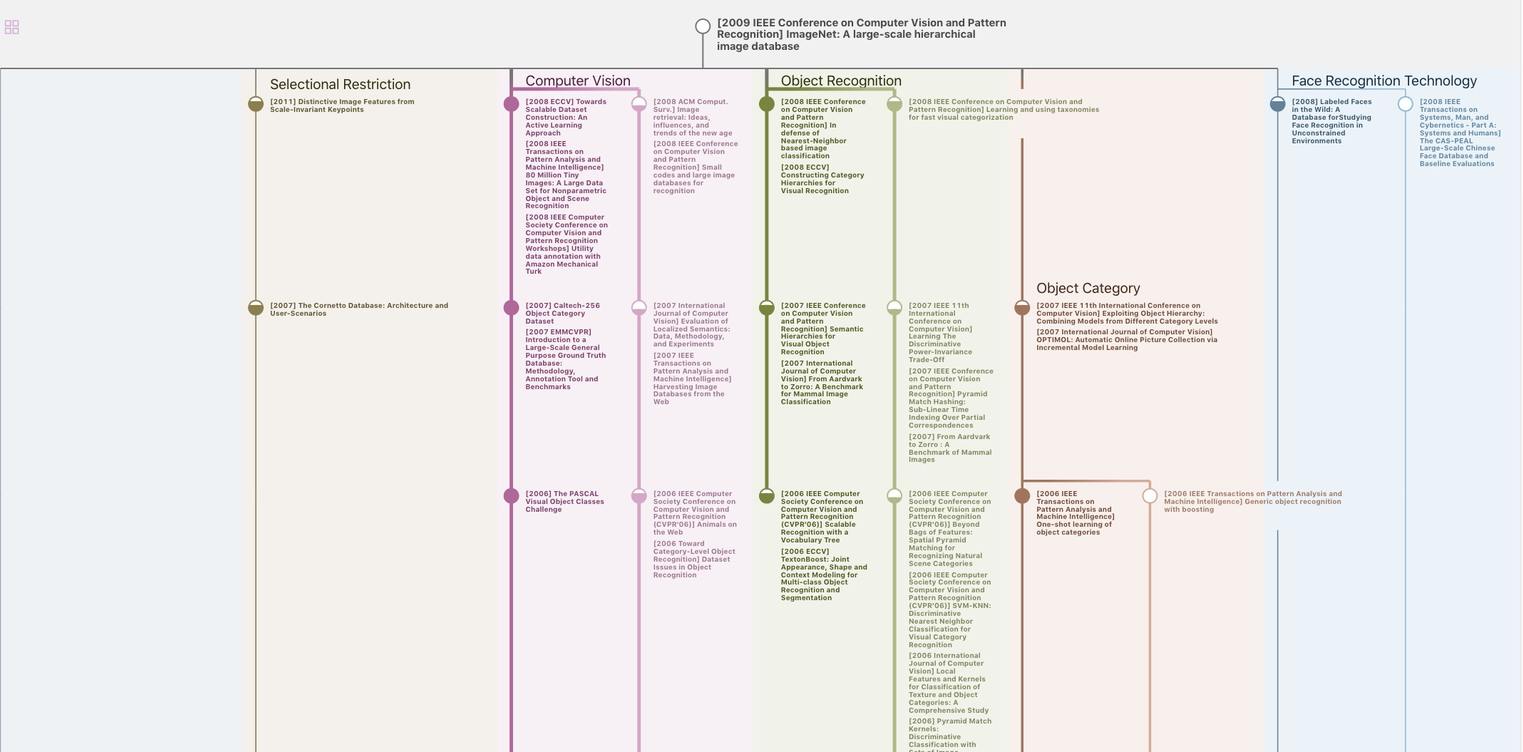
生成溯源树,研究论文发展脉络
Chat Paper
正在生成论文摘要