A preliminary study of deep learning-based reconstruction specialized for denoising in high-frequency domain: usefulness in high-resolution three-dimensional magnetic resonance cisternography of the cerebellopontine angle
NEURORADIOLOGY(2020)
摘要
Purpose Deep learning-based reconstruction (DLR) has been developed to reduce image noise and increase the signal-to-noise ratio (SNR). We aimed to evaluate the efficacy of DLR for high spatial resolution (HR)-MR cisternography. Methods This retrospective study included 35 patients who underwent HR-MR cisternography. The images were reconstructed with or without DLR. The SNRs of the CSF and pons, contrast of the CSF and pons, and sharpness of the normal-side trigeminal nerve using full width at half maximum (FWHM) were compared between the two image types. Noise quality, sharpness, artifacts, and overall image quality of these two types of images were qualitatively scored. Results The SNRs of the CSF and pons were significantly higher with DLR than without DLR (CSF 21.81 ± 7.60 vs. 15.33 ± 4.03, p < 0.001; pons 5.96 ± 1.38 vs. 3.99 ± 0.48, p < 0.001). There were no significant differences in the contrast of the CSF and pons ( p = 0.225) and sharpness of the normal-side trigeminal nerve using FWHM ( p = 0.185) without and with DLR, respectively. Noise quality and the overall image quality were significantly higher with DLR than without DLR (noise quality 3.95 ± 0.19 vs. 2.53 ± 0.44, p < 0.001; overall image quality 3.97 ± 0.17 vs. 2.97 ± 0.12, p < 0.001). There were no significant differences in sharpness ( p = 0.371) and artifacts ( p = 1) without and with DLR. Conclusion DLR can improve the image quality of HR-MR cisternography by reducing image noise without sacrificing contrast or sharpness.
更多查看译文
关键词
Deep learning,Image reconstruction,Magnetic resonance imaging,Noise,Signal-to-noise ratio
AI 理解论文
溯源树
样例
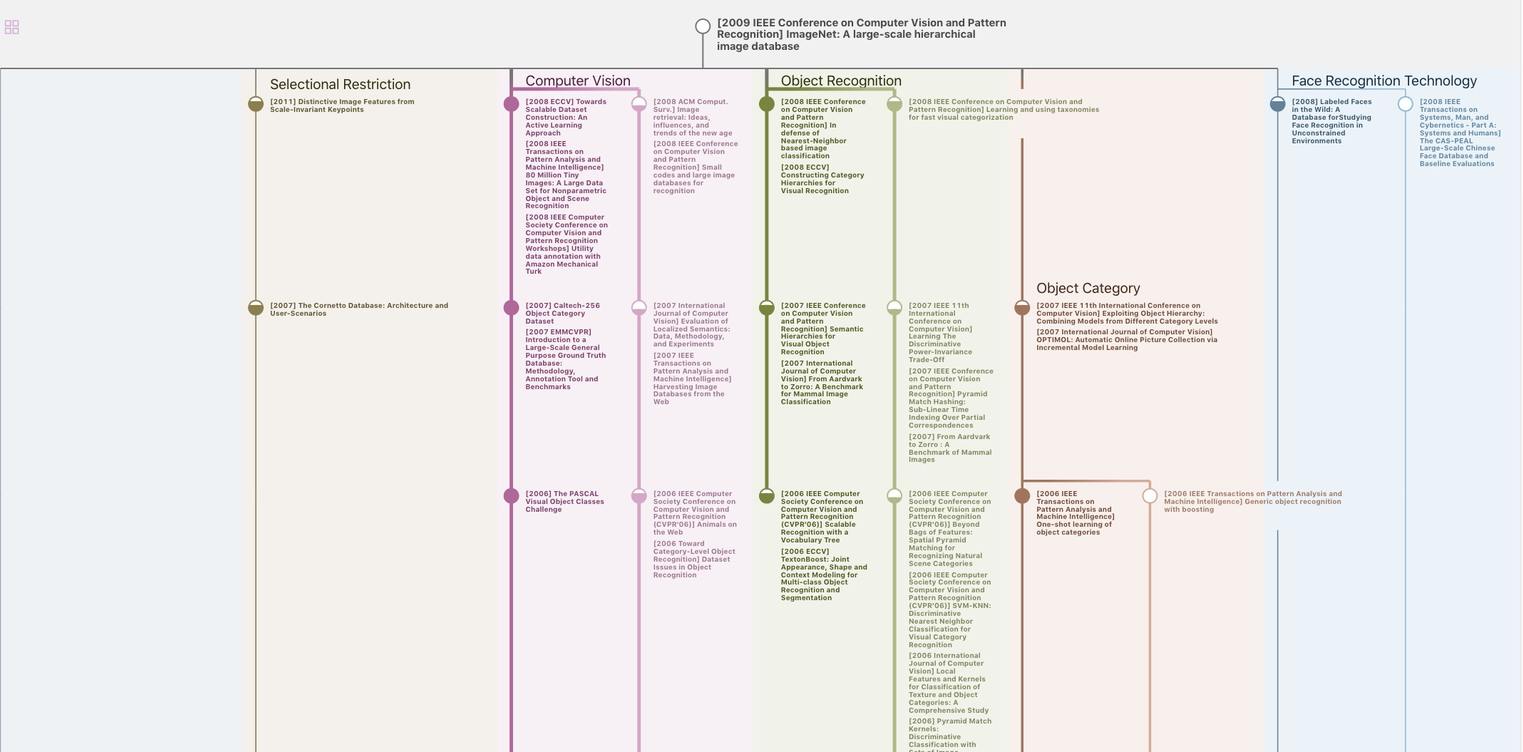
生成溯源树,研究论文发展脉络
Chat Paper
正在生成论文摘要