Perceive, Predict, and Plan: Safe Motion Planning Through Interpretable Semantic Representations
European Conference on Computer Vision(2020)
摘要
In this paper we propose a novel end-to-end learnable network that performs joint perception, prediction and motion planning for self-driving vehicles and produces interpretable intermediate representations. Unlike existing neural motion planners, our motion planning costs are consistent with our perception and prediction estimates. This is achieved by a novel differentiable semantic occupancy representation that is explicitly used as cost by the motion planning process. Our network is learned end-to-end from human demonstrations. The experiments in a large-scale manual-driving dataset and closed-loop simulation show that the proposed model significantly outperforms state-of-the-art planners in imitating the human behaviors while producing much safer trajectories.
更多查看译文
关键词
safe motion planning,predict
AI 理解论文
溯源树
样例
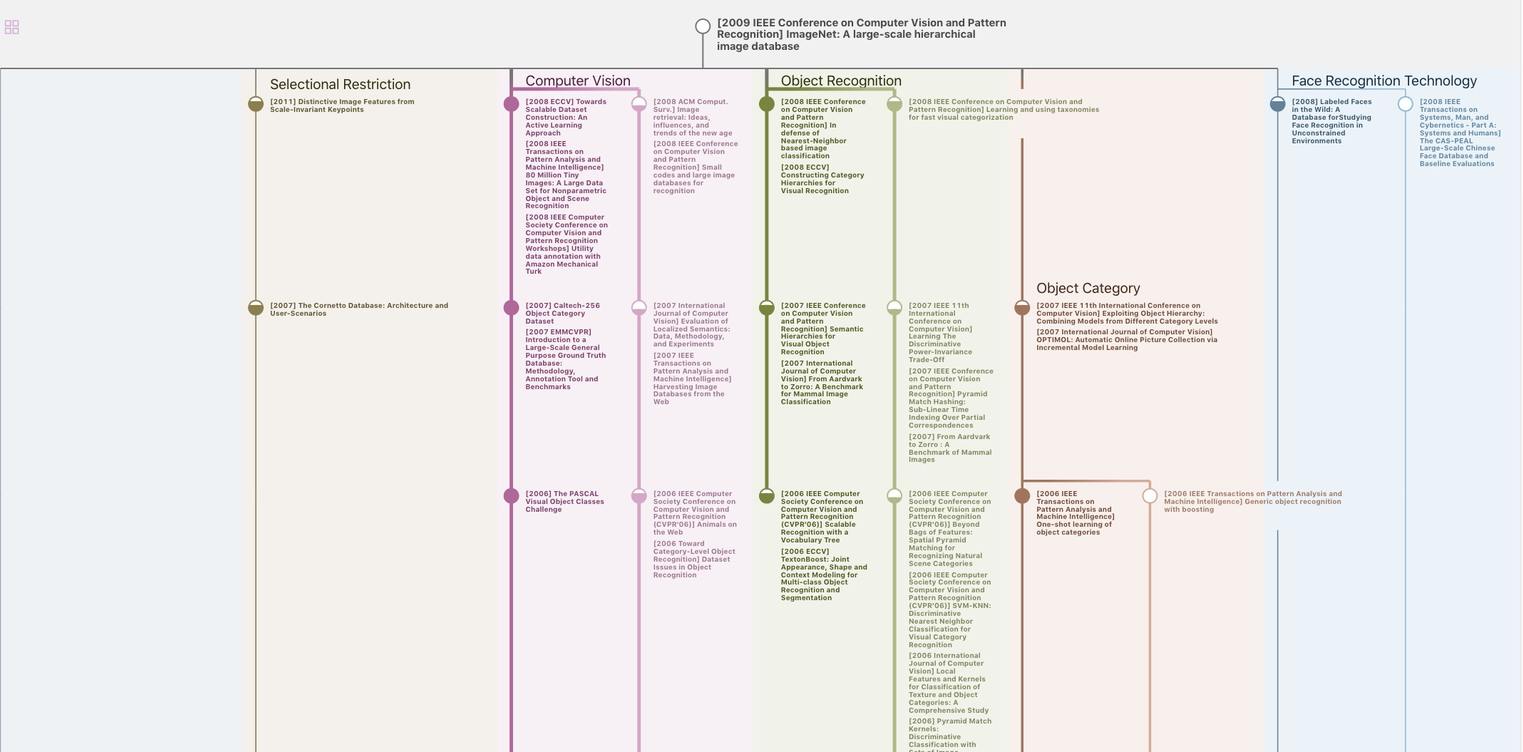
生成溯源树,研究论文发展脉络
Chat Paper
正在生成论文摘要