Practical Multisource Transfer Regression With Source–Target Similarity Captures
IEEE Transactions on Neural Networks and Learning Systems(2021)
摘要
A key challenge in many applications of multisource transfer learning is to explicitly capture the diverse source-target similarities. In this article, we are concerned with stretching the set of practical approaches based on Gaussian process (GP) models to solve multisource transfer regression problems. Precisely, we first investigate the feasibility and performance of a family of transfer covariance functions that represent the pairwise similarity of each source and the target domain. We theoretically show that using such a transfer covariance function for general GP modeling can only capture the same similarity coefficient for all the sources, and thus may result in unsatisfactory transfer performance. This outcome, together with the scalability issues of a single GP based approach, leads us to propose TC
MS
Stack, an integrated framework incorporating a separate transfer covariance function for each source and stacking. Contrary to typical stacking approaches, TC
MS
Stack learns the source-target similarity in each base GP model by considering the dependencies of the other sources along the process. We introduce two instances of the proposed TC
MS
Stack. Extensive experiments on one synthetic and two real-world data sets, with learning settings up to 11 sources for the latter, demonstrate the effectiveness of our approach.
更多查看译文
关键词
Gaussian process (GP),interdomain similarity,stacking,transfer covariance function
AI 理解论文
溯源树
样例
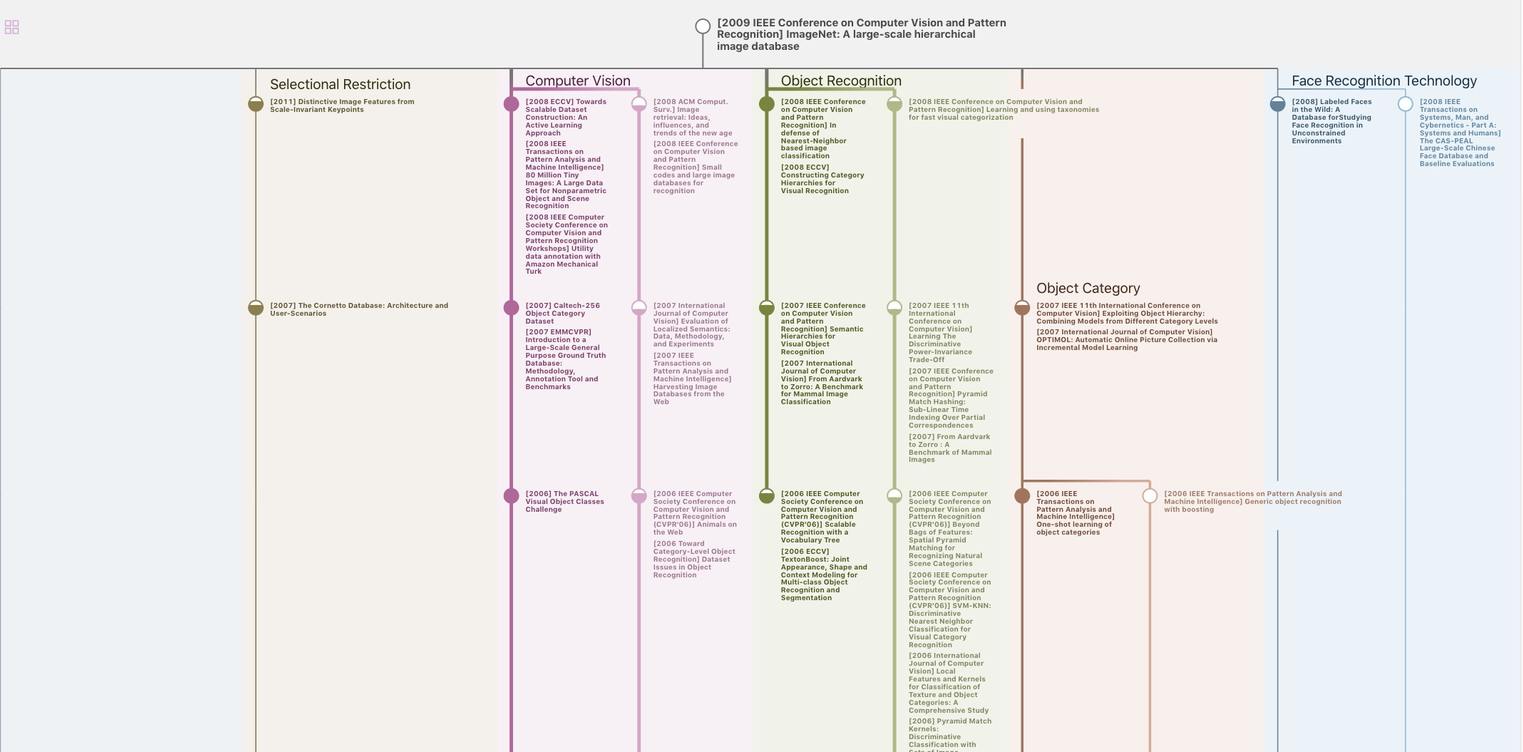
生成溯源树,研究论文发展脉络
Chat Paper
正在生成论文摘要