Modeling and Reasoning of Contexts in Smart Spaces
2020 IEEE International Conference on Pervasive Computing and Communications Workshops (PerCom Workshops)(2020)
摘要
Context can be defined to be a meaningful and descriptive state of a physical space, in which relevant activities of the humans and movements of objects in the same space are consecutively performed. Context aware computing thus requires the instantiation and use of a suitable context model and algorithms capable of discerning a most relevant context at the present time. In our previous research, we developed a method based on stochastic analysis, for inferring contexts from sensor datasets collected from a given smart space. By utilizing three statistical techniques including conditional probability table (CPT), K-means clustering, and Principal Component Analysis (PCA), our inference method is able to predict and generate contexts which can potentially happen. Generated contexts are then used as references when the present context needs to be found. In this paper, we build on our prior work and propose a method that analyzes the current state space and determines the present context guided by the collection of generated potential contexts. We first reconsolidate our context models and context graph in which contexts are structured, and then introduce reasoning methods which apply Euclidean distance and Cosine Similarity. PCA is also used to statistically analyze the state space, which helps to achieve better performance. Using experimental evaluation, we validated the accuracy of our proposed approach in identifying the present context.
更多查看译文
关键词
context awareness computing,smart spaces/cities,sensors,IoT,context graph,principal components analysis,context modeling and reasoning
AI 理解论文
溯源树
样例
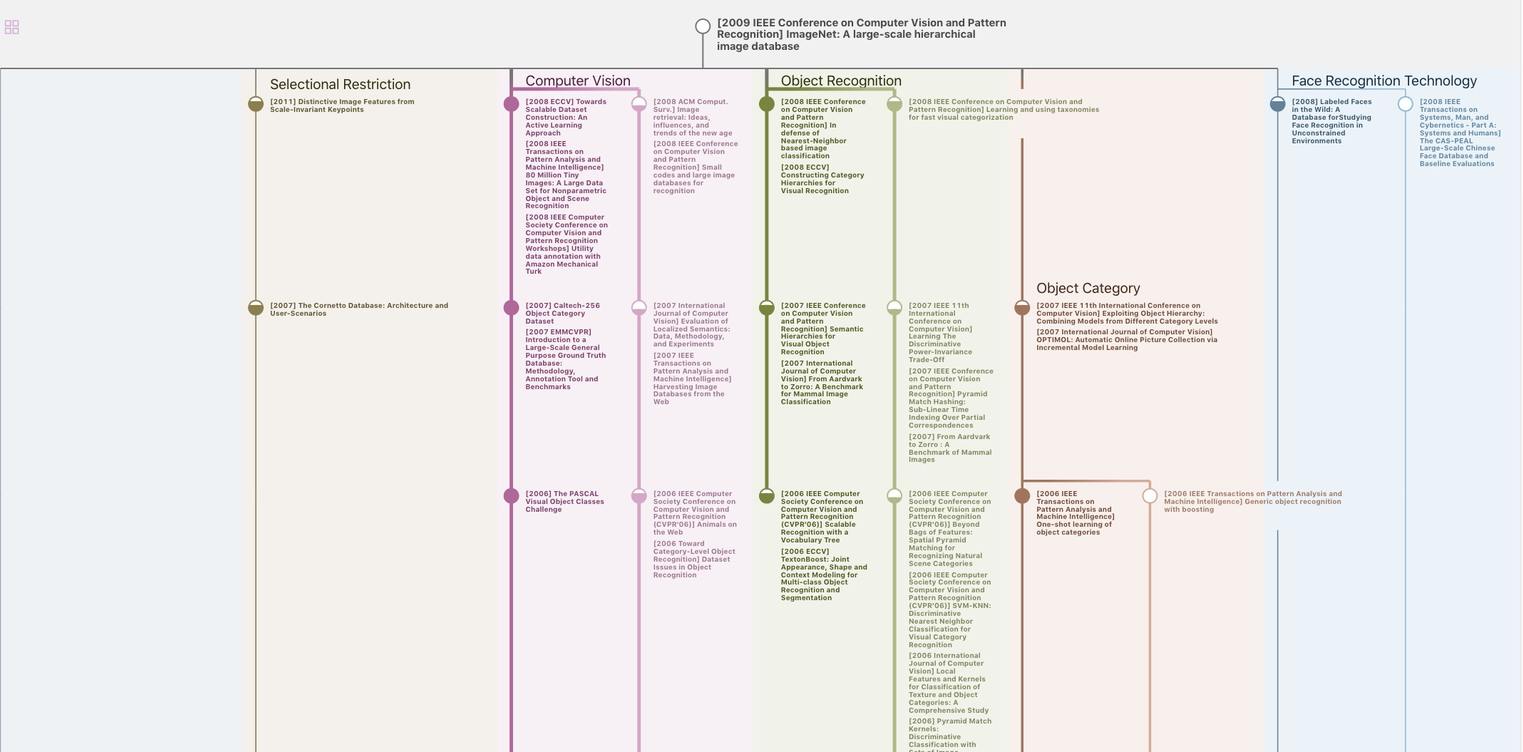
生成溯源树,研究论文发展脉络
Chat Paper
正在生成论文摘要