Towards a Deep Learning Model for Vulnerability Detection on Web Application Variants
2020 IEEE International Conference on Software Testing, Verification and Validation Workshops (ICSTW)(2020)
摘要
Reported vulnerabilities have grown significantly over the recent years, with SQL injection (SQLi) being one of the most prominent, especially in web applications. For these, such increase can be explained by the integration of multiple software parts (e.g., various plugins and modules), often developed by different organizations, composing thus web application variants. Machine Learning has the potential to be a great ally on finding vulnerabilities, aiding experts by reducing the search space or even by classifying programs on their own. However, previous work usually does not consider SQLi or utilizes techniques hard to scale. Moreover, there is a clear gap in vulnerability detection with machine learning for PHP, the most popular server-side language for web applications. This paper presents a Deep Learning model able to classify PHP slices as vulnerable (or not) to SQLi. As slices can belong to any variant, we propose the use of an intermediate language to represent the slices and interpret them as text, resorting to well-studied Natural Language Processing (NLP) techniques. Preliminary results of the use of the model show that it can discover SQLi, helping programmers and precluding attacks that would eventually cost a lot to repair.
更多查看译文
关键词
web application vulnerabilities,vulnerability detection,natural language processing,deep learning,software security
AI 理解论文
溯源树
样例
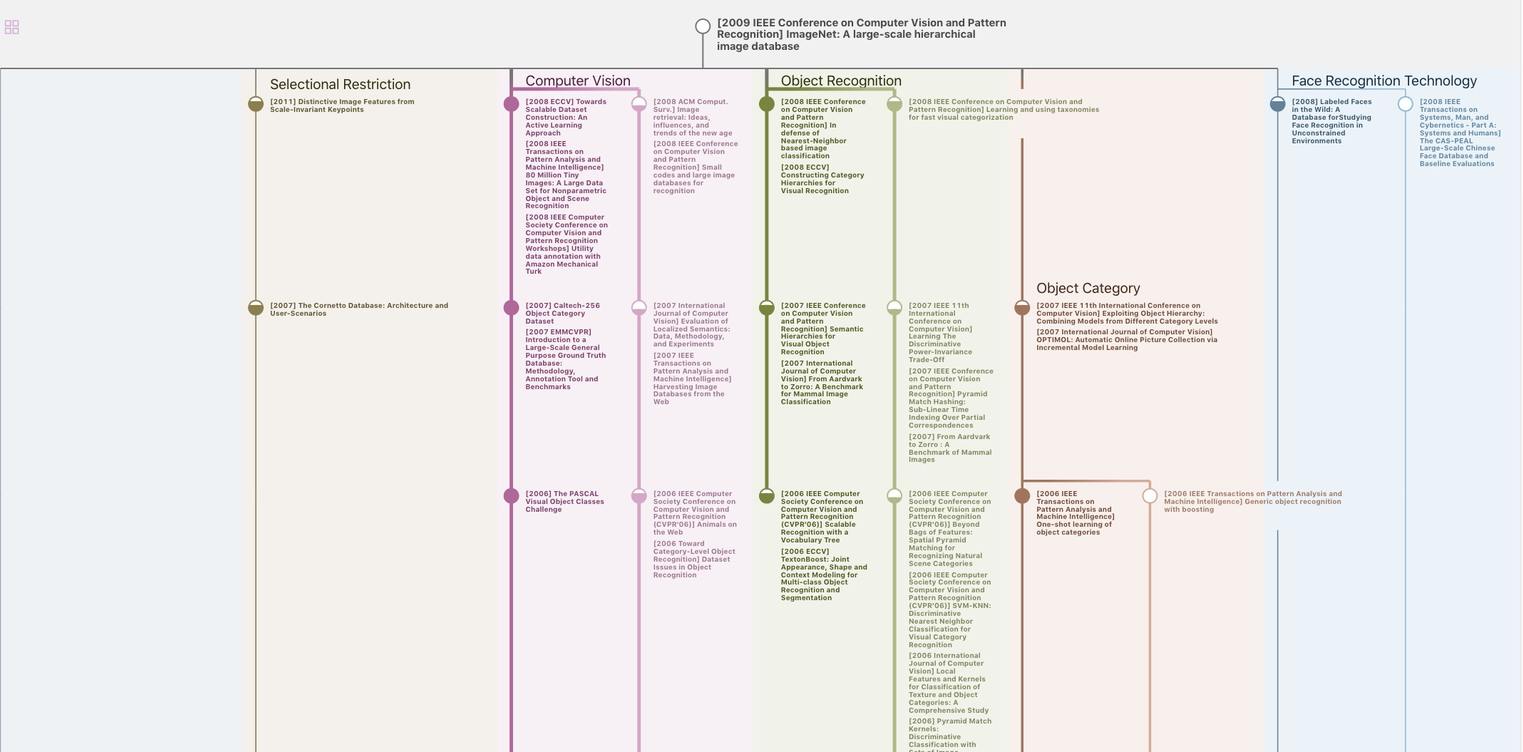
生成溯源树,研究论文发展脉络
Chat Paper
正在生成论文摘要