Look, Listen, and Attend: Co-Attention Network for Self-Supervised Audio-Visual Representation Learning
MM '20: The 28th ACM International Conference on Multimedia Seattle WA USA October, 2020(2020)
摘要
When watching videos, the occurrence of a visual event is often accompanied by an audio event, e.g., the voice of lip motion, the music of playing instruments. There is an underlying correlation between audio and visual events, which can be utilized as free supervised information to train a neural network by solving the pretext task of audio-visual synchronization. In this paper, we propose a novel self-supervised framework with co-attention mechanism to learn generic cross-modal representations from unlabelled videos in the wild, and further benefit downstream tasks. Specifically, we explore three different co-attention modules to focus on discriminative visual regions correlated to the sounds and introduce the interactions between them. Experiments show that our model achieves state-of-the-art performance on the pretext task while having fewer parameters compared with existing methods. To further evaluate the generalizability and transferability of our approach, we apply the pre-trained model on two downstream tasks, i.e., sound source localization and action recognition. Extensive experiments demonstrate that our model provides competitive results with other self-supervised methods, and also indicate that our approach can tackle the challenging scenes which contain multiple sound sources.
更多查看译文
关键词
Self-Supervised Learning, Representation Learning, Co-Attention Network, Audio-Visual Synchronization
AI 理解论文
溯源树
样例
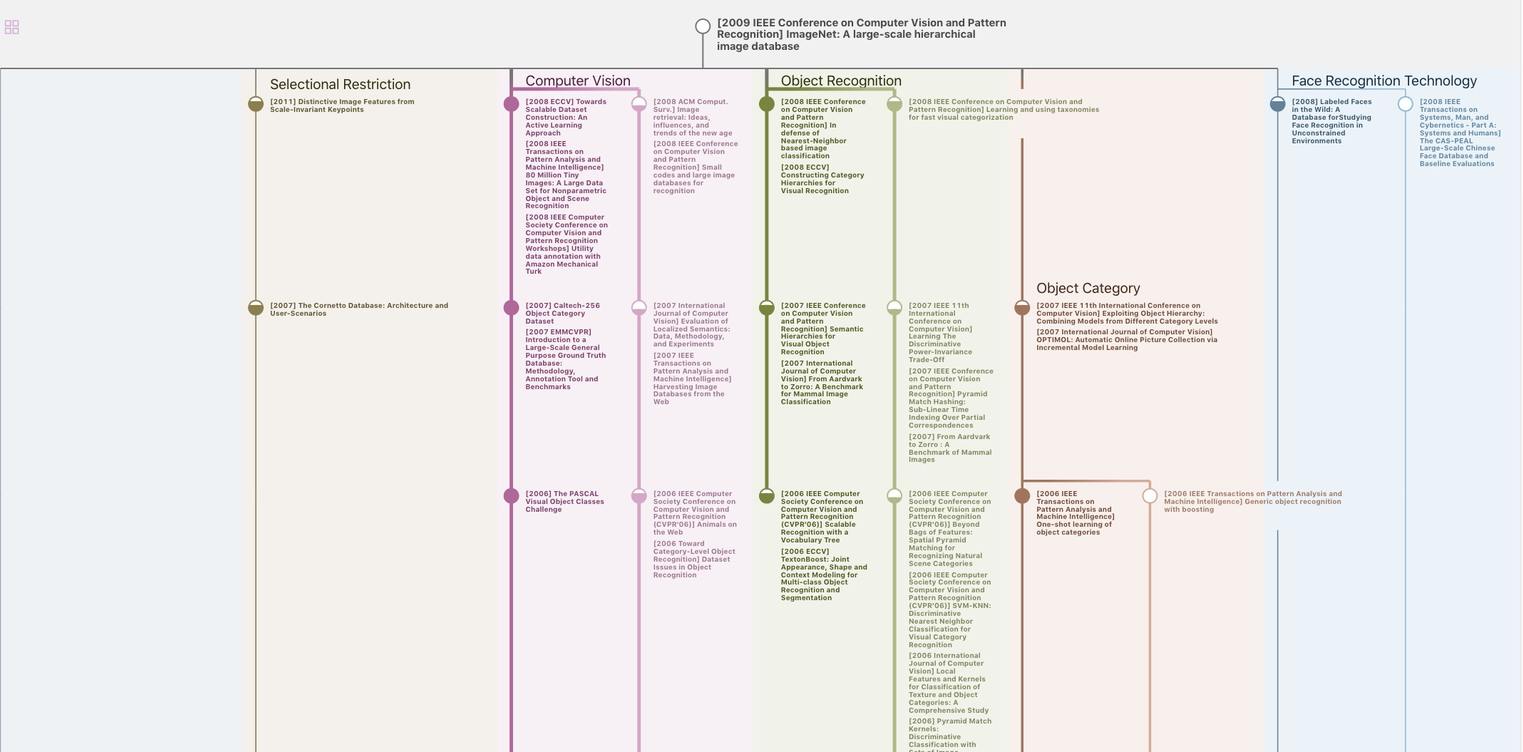
生成溯源树,研究论文发展脉络
Chat Paper
正在生成论文摘要