Wheat ear counting using K-means clustering segmentation and convolutional neural network.
Plant methods(2020)
摘要
BACKGROUND:Wheat yield is influenced by the number of ears per unit area, and manual counting has traditionally been used to estimate wheat yield. To realize rapid and accurate wheat ear counting, K-means clustering was used for the automatic segmentation of wheat ear images captured by hand-held devices. The segmented data set was constructed by creating four categories of image labels: non-wheat ear, one wheat ear, two wheat ears, and three wheat ears, which was then was sent into the convolution neural network (CNN) model for training and testing to reduce the complexity of the model.
RESULTS:The recognition accuracy of non-wheat, one wheat, two wheat ears, and three wheat ears were 99.8, 97.5, 98.07, and 98.5%, respectively. The model R 2 reached 0.96, the root mean square error (RMSE) was 10.84 ears, the macro F1-score and micro F1-score both achieved 98.47%, and the best performance was observed during late grain-filling stage (R 2 = 0.99, RMSE = 3.24 ears). The model could also be applied to the UAV platform (R 2 = 0.97, RMSE = 9.47 ears).
CONCLUSIONS:The classification of segmented images as opposed to target recognition not only reduces the workload of manual annotation but also improves significantly the efficiency and accuracy of wheat ear counting, thus meeting the requirements of wheat yield estimation in the field environment.
更多查看译文
AI 理解论文
溯源树
样例
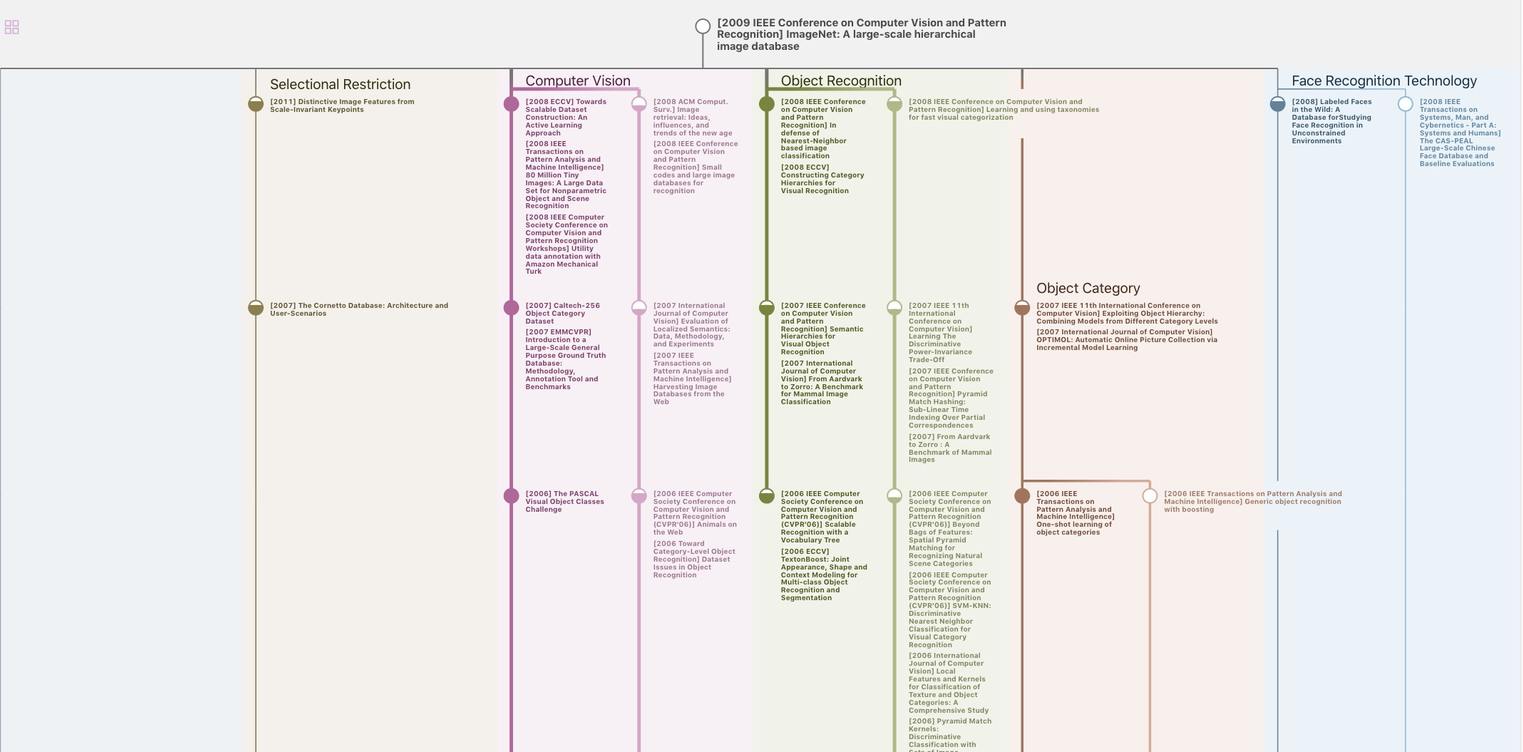
生成溯源树,研究论文发展脉络
Chat Paper
正在生成论文摘要