FedAir: Towards Multi-hop Federated Learning Over-the-Air
2020 IEEE 21st International Workshop on Signal Processing Advances in Wireless Communications (SPAWC)(2020)
摘要
Federated learning (FL) has emerged as a key technology for enabling next-generation AI at scale. The classical FL systems use single-hop cellular links to deliver the local models from mobile workers to edge routers that then reach the remote cloud servers via high-speed Internet core for global model averaging. Due to the cost-efficiency, wireless multi-hop networks have been widely exploited to build communication backbones. Therefore, enabling FL over wireless multi-hop networks can make it accessible in a low-cost manner to everyone (e.g., under-developed areas and disaster sites). Wireless multi-hop FL, however, suffers from profound communication constraints including noisy and interference-rich wireless links, which results in slow and nomadic FL model updates. To address this, we suggest novel machine learning-enabled wireless multi-hop FL framework, namely FedAir, that can greatly mitigate the adverse impact of wireless communications on FL performance metrics such as model convergence time. This will allow us to fast prototype, deploy, and evaluate FL algorithms over ML-enabled, programmable wireless router (ML-router). The experiments on the deployed testbed validate and show that wireless multi-hop FL framework can greatly accelerate the runtime convergence speed of the de-facto FL algorithm, FedAvg.
更多查看译文
关键词
Federated Learning,Multi-hop wireless edge,Wireless Networking
AI 理解论文
溯源树
样例
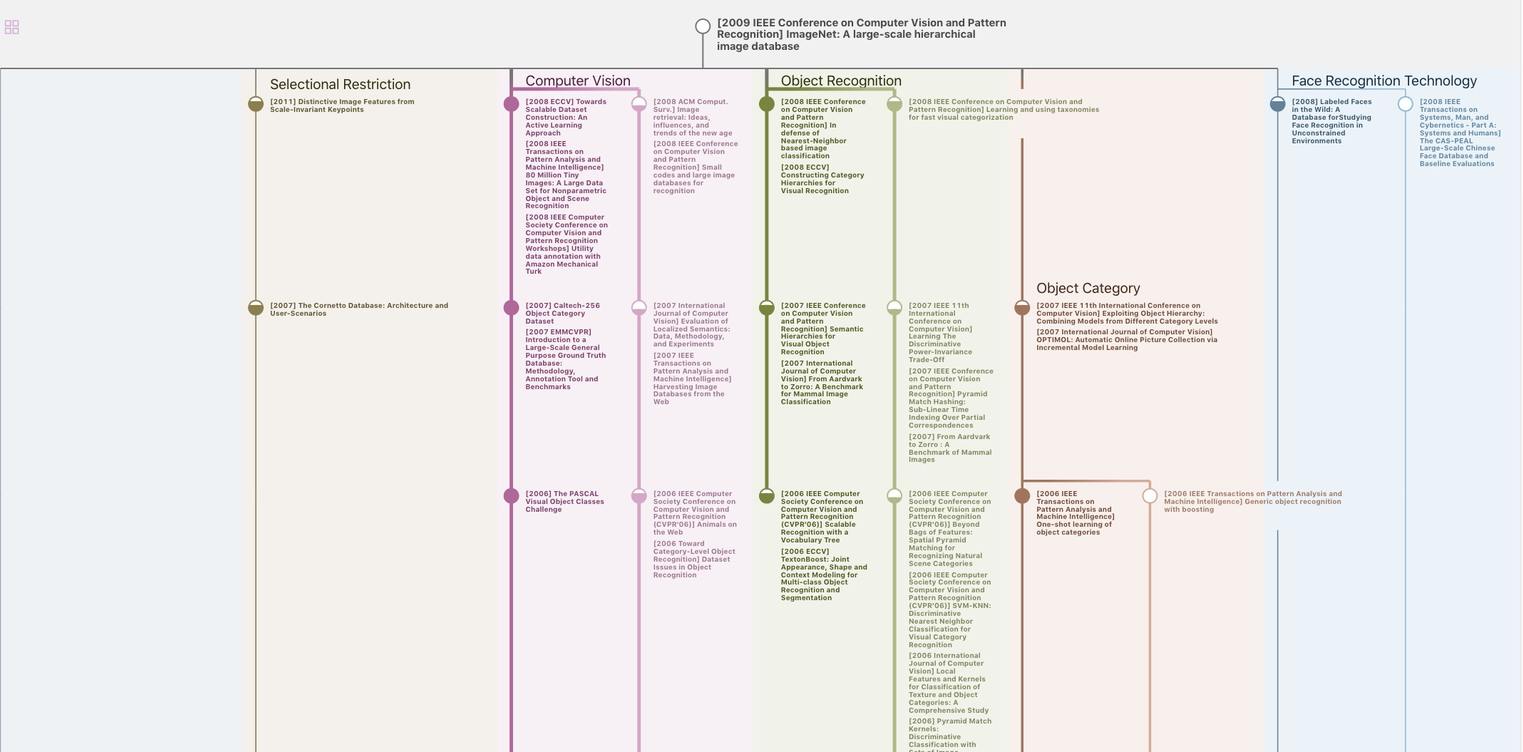
生成溯源树,研究论文发展脉络
Chat Paper
正在生成论文摘要