Joint Channel Coding And Modulation Via Deep Learning
SPAWC(2020)
摘要
Channel coding and modulation are two fundamental building blocks of physical layer wireless communications. We propose a neural network based end-to-end communication system, where both the channel coding and the modulation blocks are modeled as neural networks. Our proposed architecture combines Turbo Autoencoder together with feed-forward neural networks for modulation, and hence called TurboAE-MOD. Turbo Autoencoder was introduced in [1] and consists of a neural network based channel encoder (convolutional neural networks with an interleaver) and a neural network based decoder (iterations of convolutional neural networks with interleavers and de-interleavers in between). By allowing joint training of the channel coding and modulation in an end-to-end manner, we demonstrate that TurboAE-MOD performs comparable to modern codes stacked with canonical modulations for moderate block lengths. We also demonstrate that TurboAE-MOD learns interesting modulation patterns that are amenable to meaningful interpretations.
更多查看译文
关键词
Channel Coding, Modulation, Autoencoder, Turbo Principle, Deep Learning
AI 理解论文
溯源树
样例
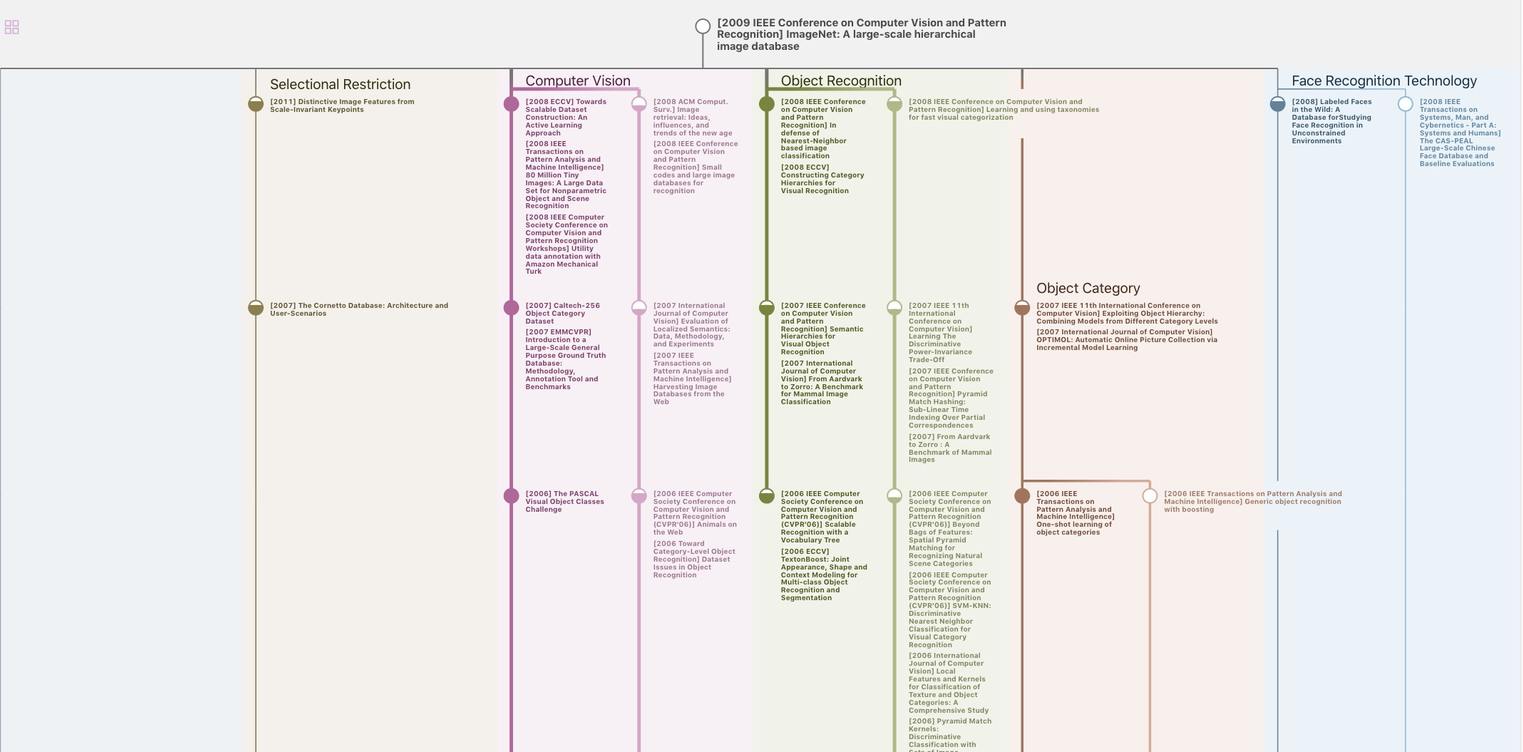
生成溯源树,研究论文发展脉络
Chat Paper
正在生成论文摘要