FedSKETCH: Communication-Efficient and Private Federated Learning via Sketching
Unknown Journal(2020)
摘要
Communication complexity and privacy are the two key challenges in Federated Learning where the goal is to perform a distributed learning through a large volume of devices. In this work, we introduce FedSKETCH and FedSKETCHGATE algorithms to address both challenges in Federated learning jointly, where these algorithms are intended to be used for homogeneous and heterogeneous data distribution settings respectively. The key idea is to compress the accumulation of local gradients using count sketch, therefore, the server does not have access to the gradients themselves which provides privacy. Furthermore, due to the lower dimension of sketching used, our method exhibits communication-efficiency property as well. We provide, for the aforementioned schemes, sharp convergence guarantees. Finally, we back up our theory with various set of experiments.
更多查看译文
关键词
private federated learning,federated learning,communication-efficient
AI 理解论文
溯源树
样例
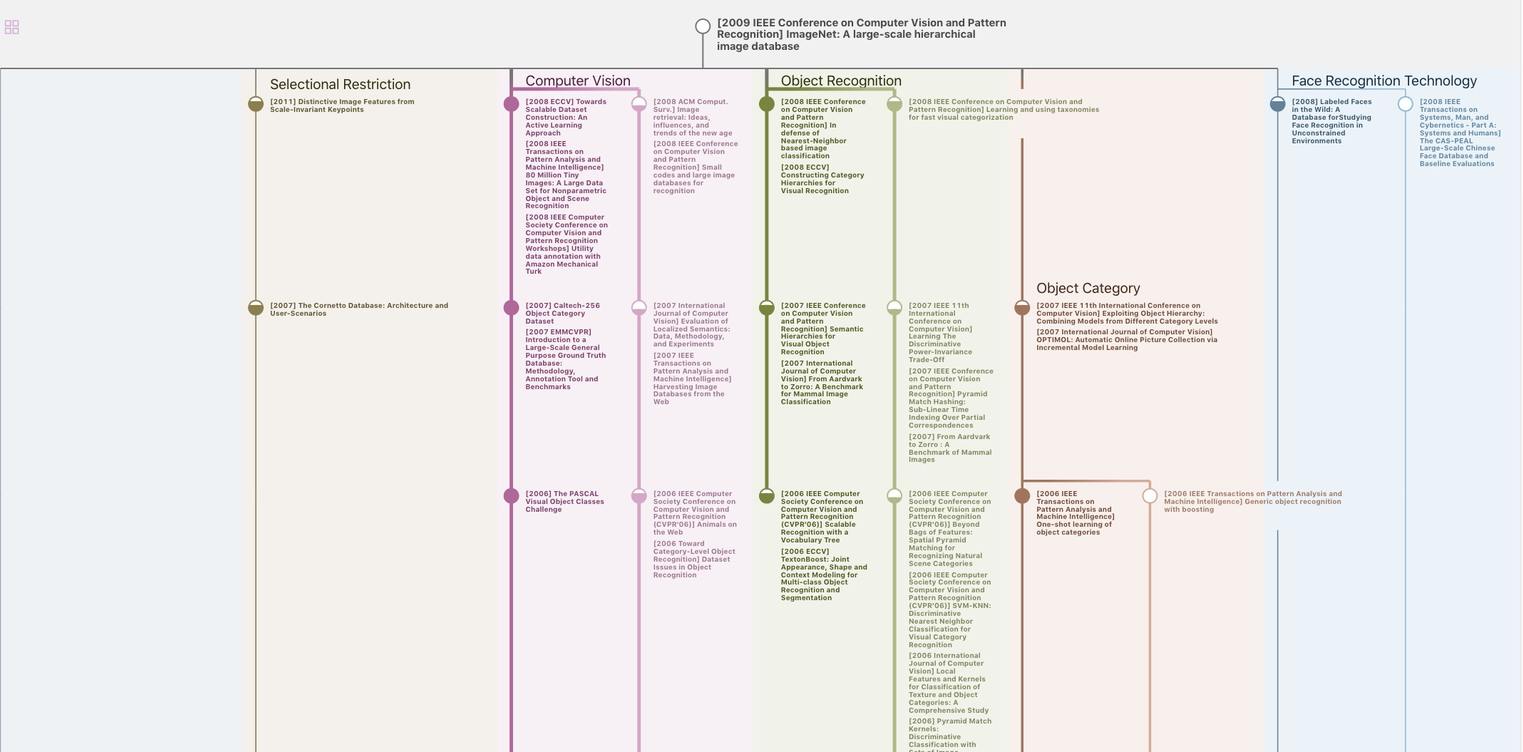
生成溯源树,研究论文发展脉络
Chat Paper
正在生成论文摘要