Neural Collaborative Preference Learning With Pairwise Comparisons
IEEE TRANSACTIONS ON MULTIMEDIA(2021)
摘要
Collaborative Ranking (CR), as an effective recommendation framework, has attracted increasing attention in recent years. Most CR methods simply adopt the inner product between user/item embeddings as the rating score function, with an assumption that the interacted items are preferred to non-interacted ones. However, such fixed score functions and assumptions might not be sufficient to capture the real preference ranking list from the complicated interactions in real-world data. To alleviate this issue, we develop a novel collaborative ranking framework that learns an arbitrary utility function for item ranking with user preference concerned. In the core of our framework, a neural network is employed to model the utility function for personalized ranking with the strength of its nonlinearity. On top of this, we further adopt a pairwise ranking loss for user-item pairs to preserve the preference order of items for users. Besides, such a utility function enables us to generate the final top-K preference list in a much easier way. Finally, extensive experiments on four real-world datasets show the validity of our proposed method.
更多查看译文
关键词
Recommender system, collaborative ranking, neural networks, preference ranking
AI 理解论文
溯源树
样例
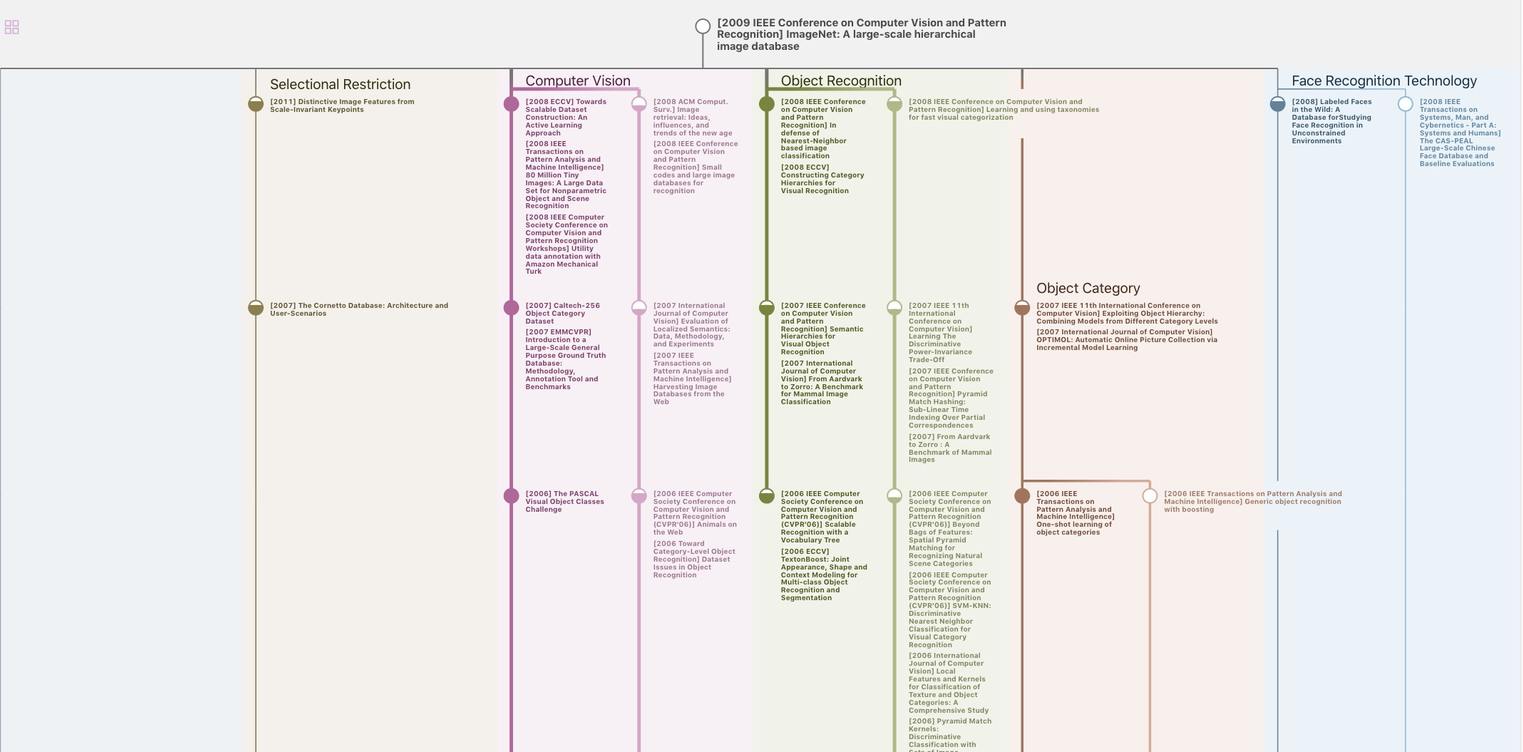
生成溯源树,研究论文发展脉络
Chat Paper
正在生成论文摘要