Rethinking Pseudo-LiDAR Representation
European Conference on Computer Vision(2020)
摘要
The recently proposed pseudo-LiDAR based 3D detectors greatly improve the benchmark of monocular/stereo 3D detection task. However, the underlying mechanism remains obscure to the research community. In this paper, we perform an in-depth investigation and observe that the efficacy of pseudo-LiDAR representation comes from the coordinate transformation, instead of data representation itself. Based on this observation, we design an image based CNN detector named PatchNet, which is more generalized and can be instantiated as pseudo-LiDAR based 3D detectors. Moreover, the pseudo-LiDAR data in our PatchNet is organized as the image representation, which means existing 2D CNN designs can be easily utilized for extracting deep features from input data and boosting 3D detection performance. We conduct extensive experiments on the challenging KITTI dataset, where the proposed PatchNet outperforms all existing pseudo-LiDAR based counterparts. Code has been made available at: https://github.com/xinzhuma/patchnet.
更多查看译文
关键词
representation,pseudo-lidar
AI 理解论文
溯源树
样例
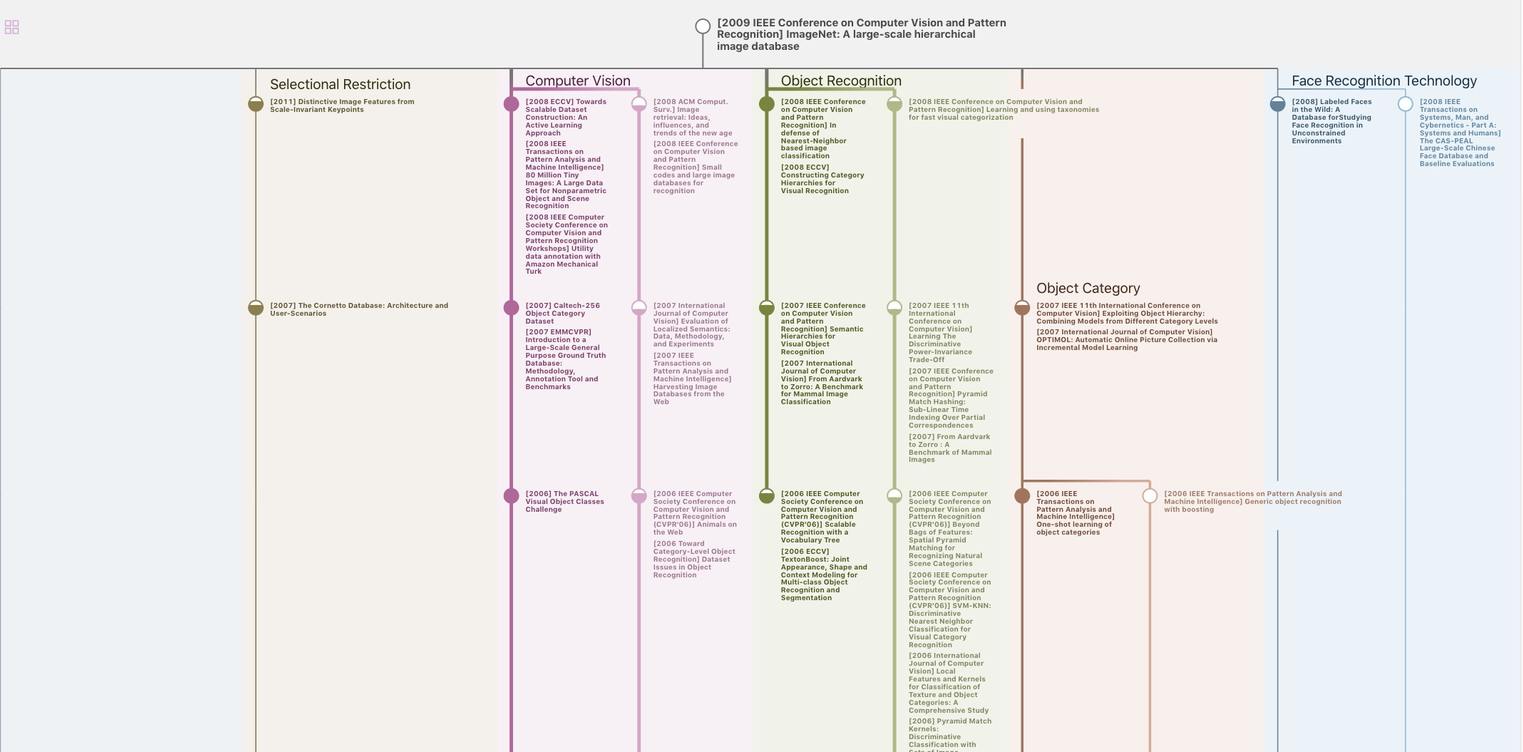
生成溯源树,研究论文发展脉络
Chat Paper
正在生成论文摘要