Differentiable Hebbian plasticity for continual learning
International conference on machine learning (ICML) adaptive and multitask learning: Algorithms & Systems (AMTL) workshop(2019)
摘要
Catastrophic forgetting poses a grand challenge for continual learning systems, which prevents neural networks from protecting old knowledge while learning new tasks sequentially. We propose a Differentiable Hebbian Plasticity (DHP) Softmax layer which adds a fast learning plastic component to the slow weights of the softmax output layer. The DHP Softmax behaves as a compressed episodic memory that reactivates existing memory traces, while creating new ones. We demonstrate the flexibility of our model by combining it with existing well-known consolidation methods to prevent catastrophic forgetting. We evaluate our approach on the Permuted MNIST and Split MNIST benchmarks, and introduce Imbalanced Permuted MNIST—a dataset that combines the challenges of class imbalance and concept drift. Our model requires no additional hyperparameters and outperforms comparable baselines by reducing forgetting.
更多查看译文
AI 理解论文
溯源树
样例
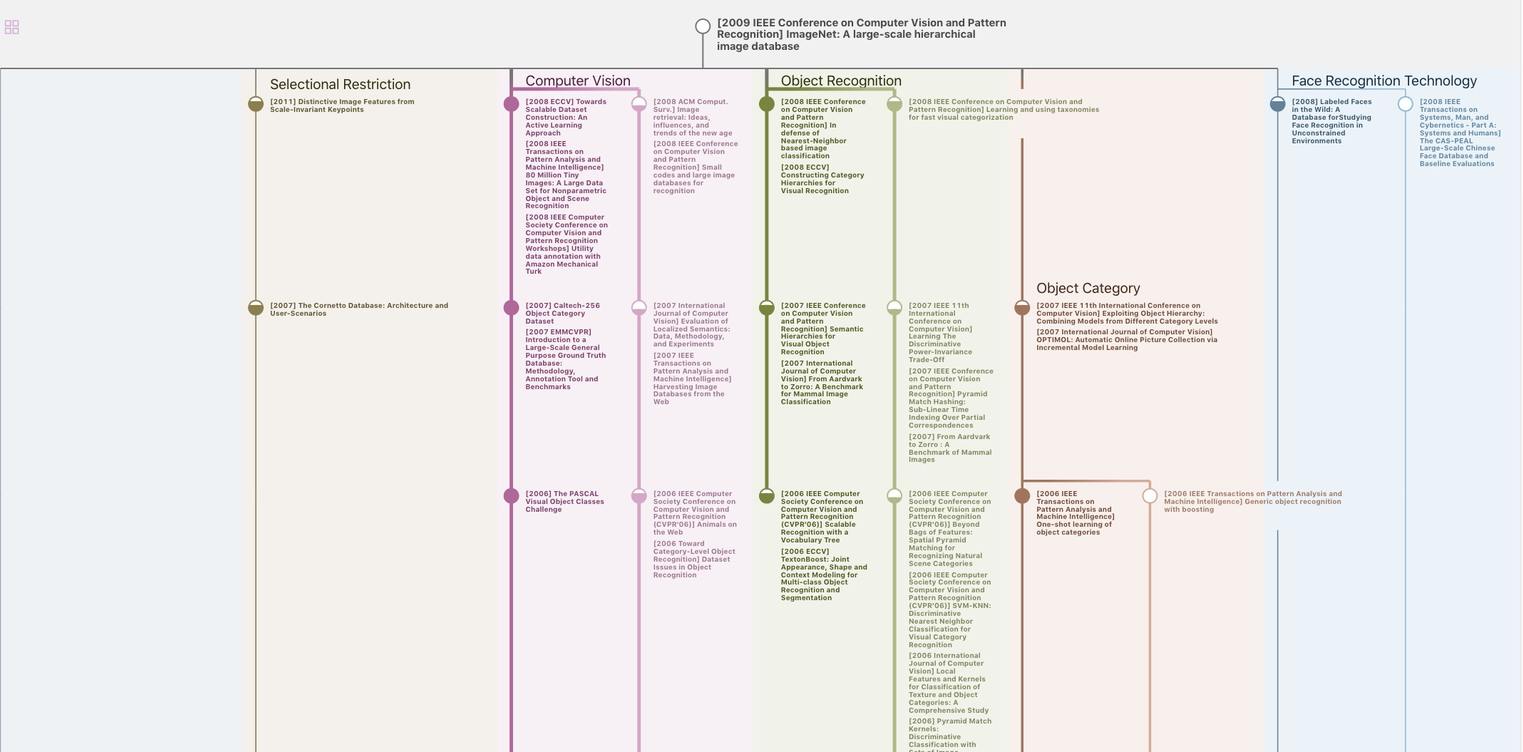
生成溯源树,研究论文发展脉络
Chat Paper
正在生成论文摘要