Stochastically Controlled Compositional Gradient for Composition Problems
IEEE Transactions on Neural Networks and Learning Systems(2023)
摘要
We consider composition problems of the form
$(1/n)\sum _{i= 1}^{n} F_{i} (1/m)\sum _{j = 1}^{m} G_{j}(x)$
, which are important for machine learning. Although gradient descent and stochastic gradient descent are straightforward solutions, the essential computation of
$G (x)= (1/m)\sum _{j = 1}^{m}{G_{j}(x)}$
in each single iteration is expensive, let alone for large
$m$
. In this article, we devise a stochastically controlled compositional gradient algorithm. Specifically, we introduce two variants of stochastically controlled technique to estimate the inner function
$G(x)$
and the gradient of the objective function, respectively. The computational cost is largely reduced. However, the natural needs of two stochastic subsets
${\mathcal D}_{1}$
and
${\mathcal D}_{2}$
form direct barriers to guarantee the convergence of the algorithm, especially the theoretical proof of the convergence. To this end, we present a general convergence analysis by proving
$|{\mathcal{ D}}_{1}|=\min \{1/\epsilon,m\}$
and
$|{\mathcal{ D}}_{2}|=\min \{1/\epsilon,n \}$
, through which the proposed method significantly improve composition algorithms under low target accuracy (i.e.,
$1/\epsilon \ll m$
or
$n$
) in both strongly convex and nonconvex settings. Comprehensive experiments demonstrate the superiority of the proposed method over existing methods.
更多查看译文
关键词
Composition problem,stochastic optimization,stochastically controlled gradient,variance reduction
AI 理解论文
溯源树
样例
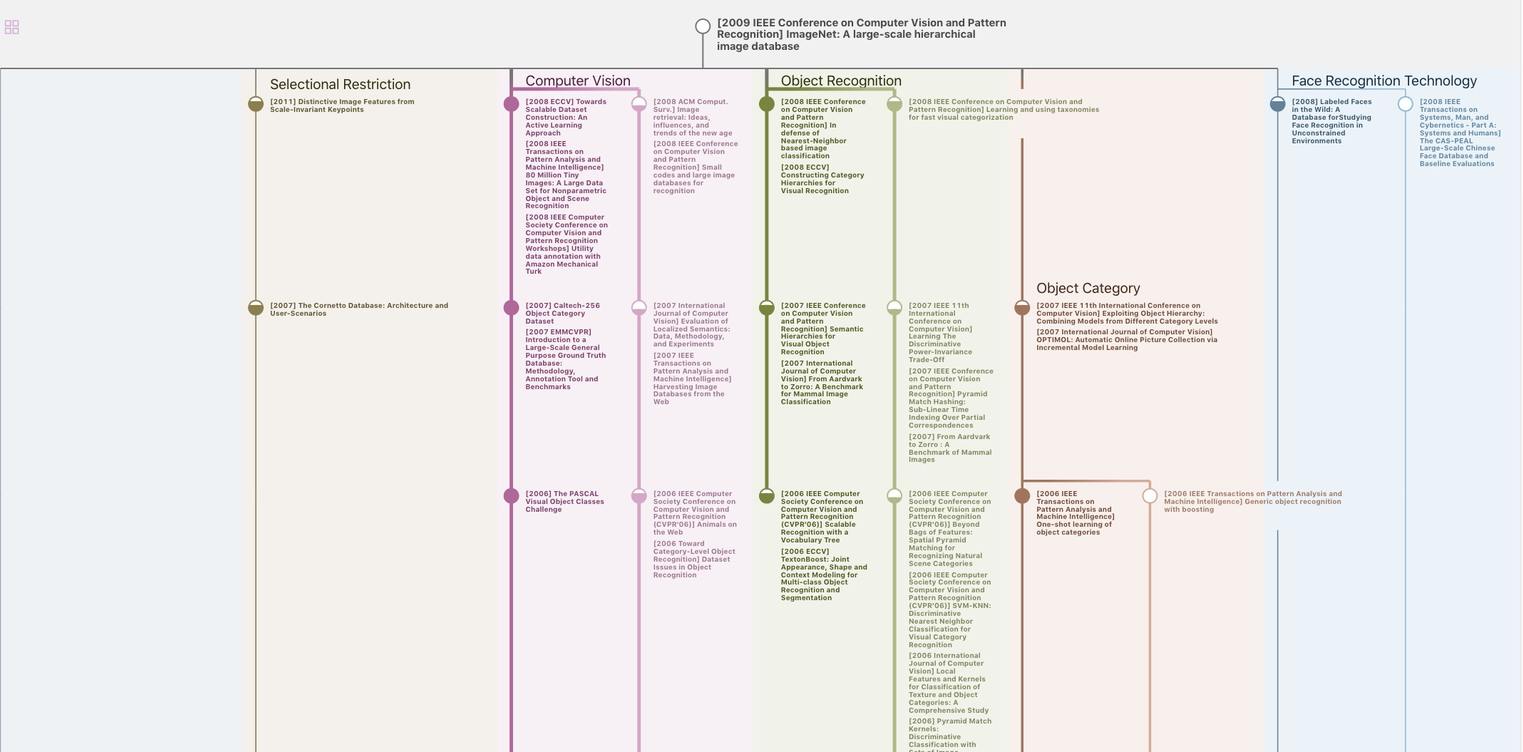
生成溯源树,研究论文发展脉络
Chat Paper
正在生成论文摘要