Prototype Mixture Models for Few-Shot Semantic Segmentation
European Conference on Computer Vision(2020)
摘要
Few-shot segmentation is challenging because objects within the support and query images could significantly differ in appearance and pose. Using a single prototype acquired directly from the support image to segment the query image causes semantic ambiguity. In this paper, we propose prototype mixture models (PMMs), which correlate diverse image regions with multiple prototypes to enforce the prototype-based semantic representation. Estimated by an Expectation-Maximization algorithm, PMMs incorporate rich channel-wised and spatial semantics from limited support images. Utilized as representations as well as classifiers, PMMs fully leverage the semantics to activate objects in the query image while depressing background regions in a duplex manner. Extensive experiments on Pascal VOC and MS-COCO datasets show that PMMs significantly improve upon state-of-the-arts. Particularly, PMMs improve 5-shot segmentation performance on MS-COCO by up to 5.82% with only a moderate cost for model size and inference speed (Code is available at github.com/Yang-Bob/PMMs.).
更多查看译文
关键词
Semantic segmentation,Few-shot segmentation,Few-shot learning,Mixture models
AI 理解论文
溯源树
样例
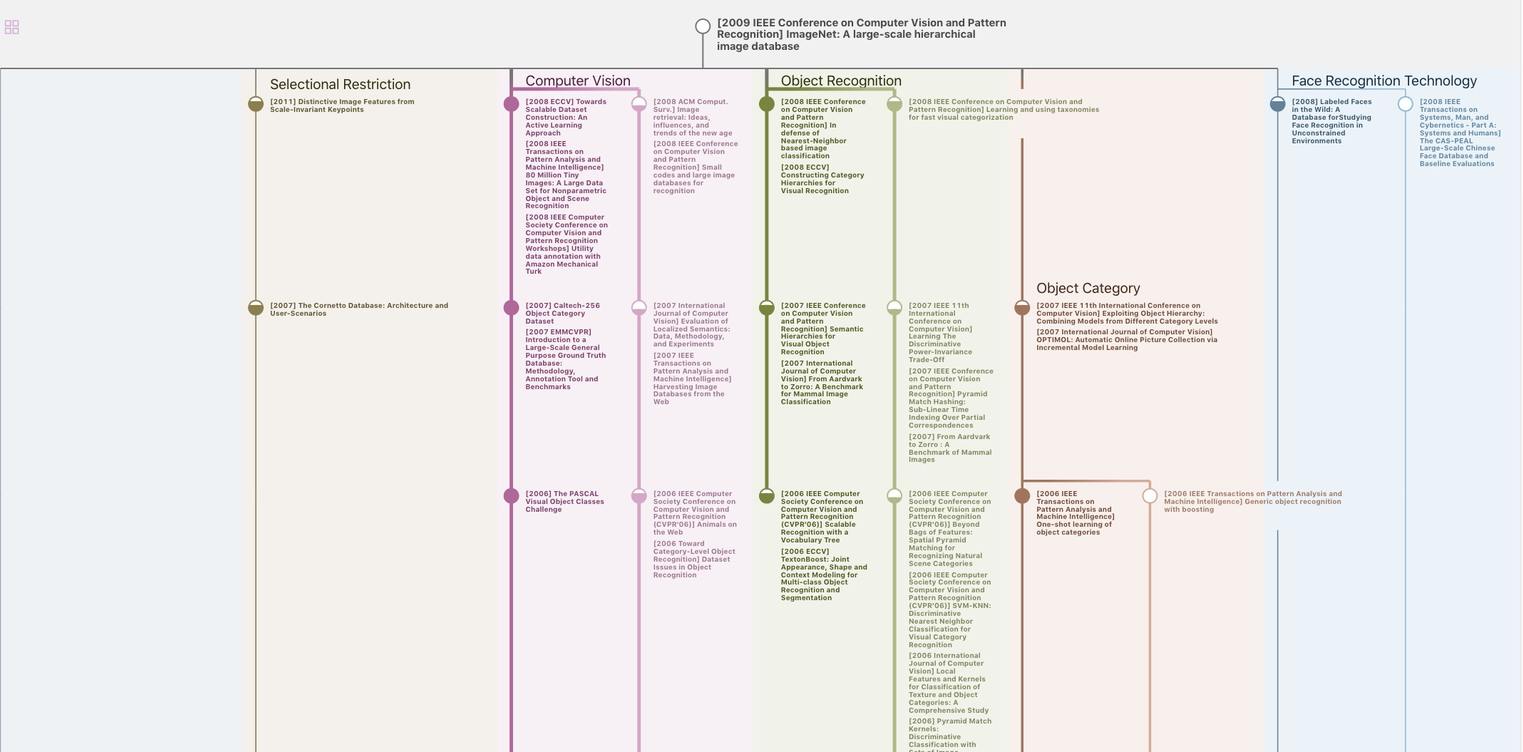
生成溯源树,研究论文发展脉络
Chat Paper
正在生成论文摘要