Adaptive support-driven Bayesian reweighted algorithm for sparse signal recovery
SIGNAL IMAGE AND VIDEO PROCESSING(2021)
摘要
This paper presents an adaptive support-driven Bayesian reweighted algorithm based on shrinkage thresholding, for large-scale sparse signal recovery. The proposed algorithm is based on the widely used sparse Bayesian learning (SBL) methods, which relies on parameterized prior . SBL leads to a nonconvex optimization problem that encourages model with few nonzero coefficients. In SBL, sparse signal recovery problems can be solved by a reweighted ℓ _1 -regularization algorithm. However, this algorithm is expensive in computation and memory, especially for large-scale problem. To alleviate this problem, we proposed a restart strategy leading to the adaptive support estimate, which reduces the computational burden and memory simultaneously. Numerical experiments demonstrate the effectiveness and computational advantages of the proposed algorithm.
更多查看译文
关键词
Sparse Bayesian learning,Adaptive support estimate,Sparse signal recovery
AI 理解论文
溯源树
样例
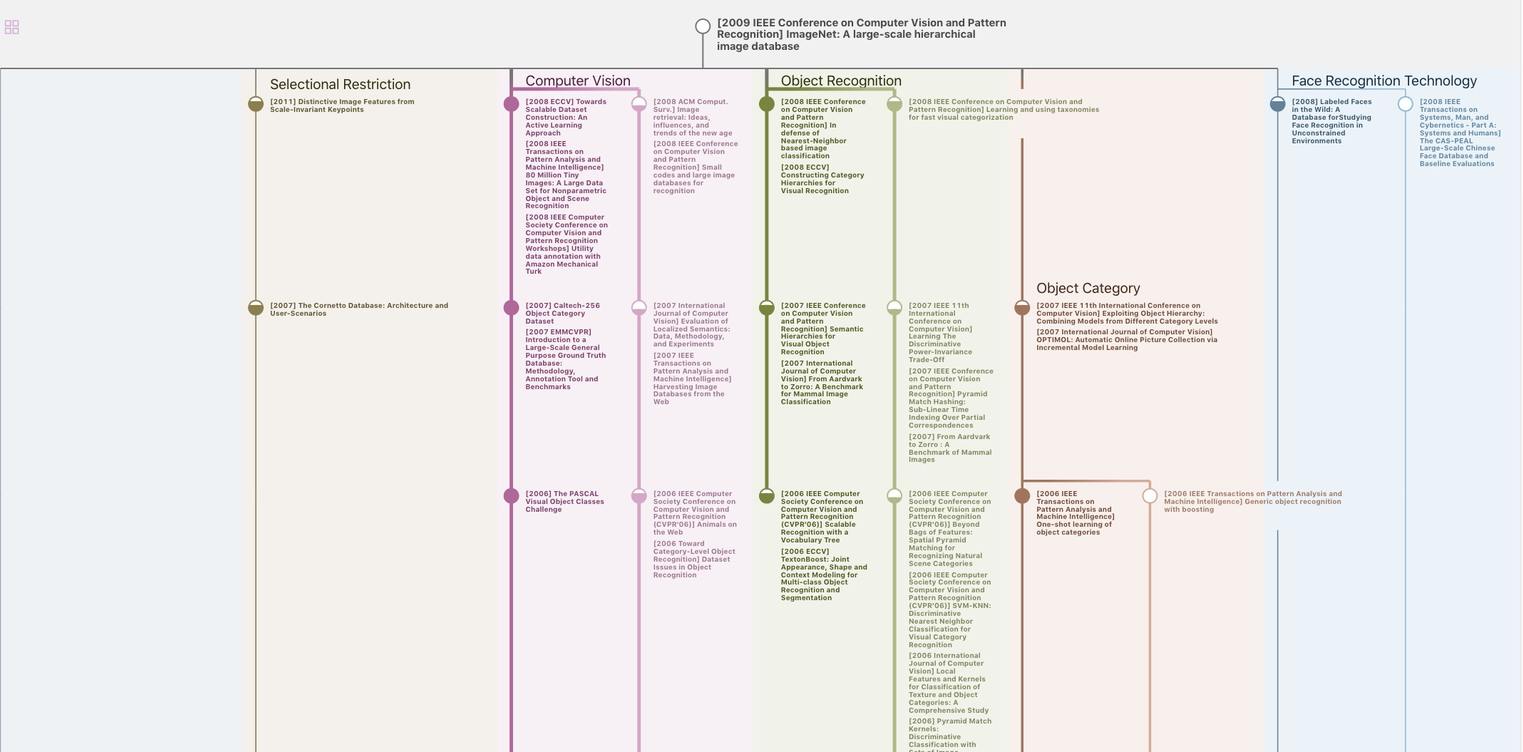
生成溯源树,研究论文发展脉络
Chat Paper
正在生成论文摘要