How Trustworthy are the Existing Performance Evaluations for Basic Vision Tasks?
CoRR(2020)
摘要
Performance evaluation is indispensable to the advancement of machine vision, yet its consistency and rigour have not received proportionate attention. This paper examines performance evaluation criteria for basic vision tasks namely, object detection, instance-level segmentation and multi-object tracking. Specifically, we advocate the use of criteria that are (i) consistent with mathematical requirements such as the metric properties, (ii) contextually meaningful in sanity tests, and (iii) robust to hyper-parameters for reliability. We show that many widely used performance criteria do not fulfill these requirements. Moreover, we explore alternative criteria for detection, segmentation, and tracking, using metrics for sets of shapes, and assess them against these requirements.
更多查看译文
AI 理解论文
溯源树
样例
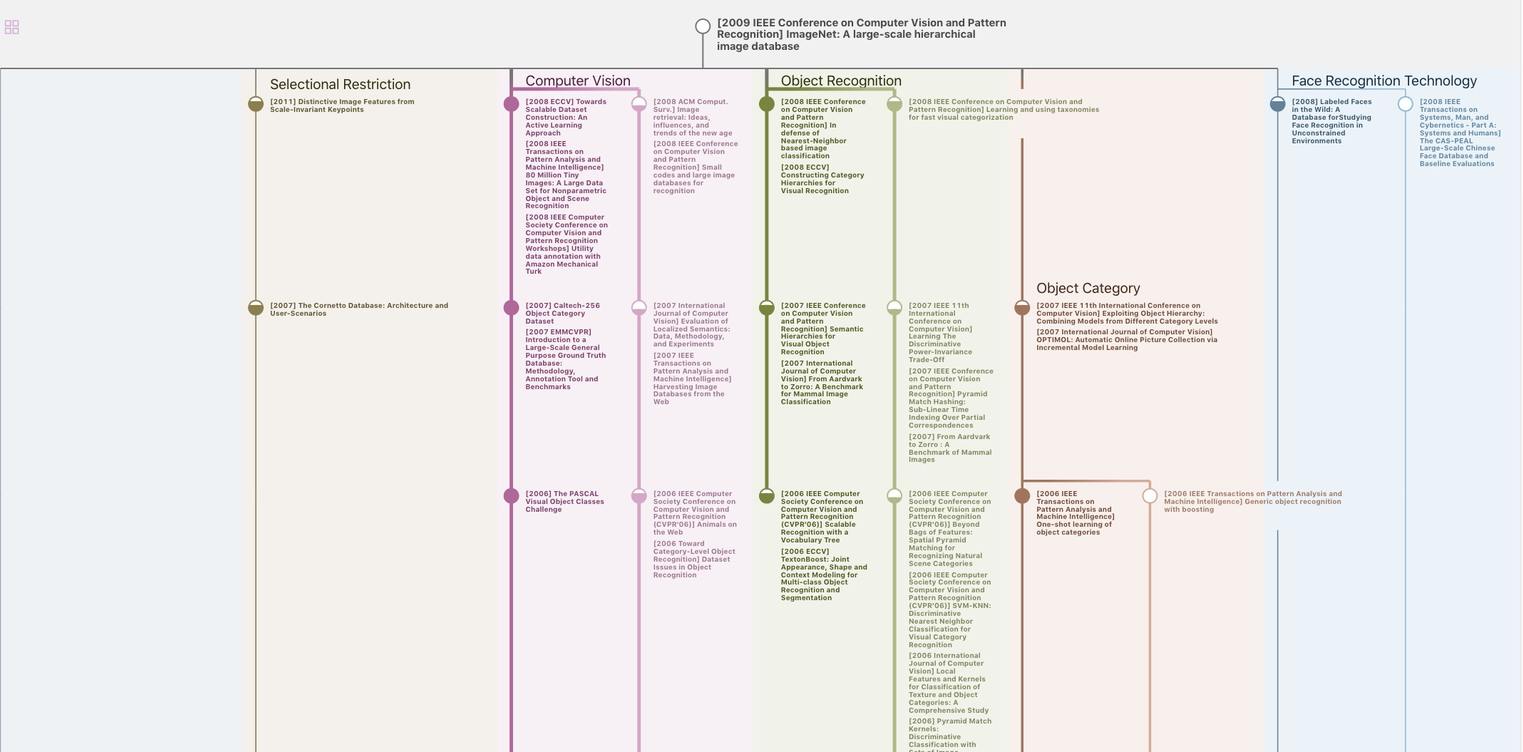
生成溯源树,研究论文发展脉络
Chat Paper
正在生成论文摘要