RPT: Learning Point Set Representation for Siamese Visual Tracking
ECCV Workshops(2020)
摘要
While remarkable progress has been made in robust visual tracking, accurate target state estimation still remains a highly challenging problem. In this paper, we argue that this issue is closely related to the prevalent bounding box representation, which provides only a coarse spatial extent of object. Thus an effcient visual tracking framework is proposed to accurately estimate the target state with a finer representation as a set of representative points. The point set is trained to indicate the semantically and geometrically significant positions of target region, enabling more fine-grained localization and modeling of object appearance. We further propose a multi-level aggregation strategy to obtain detailed structure information by fusing hierarchical convolution layers. Extensive experiments on several challenging benchmarks including OTB2015, VOT2018, VOT2019 and GOT-10k demonstrate that our method achieves new state-of-the-art performance while running at over 20 FPS.
更多查看译文
关键词
siamese visual tracking,point set representation,learning
AI 理解论文
溯源树
样例
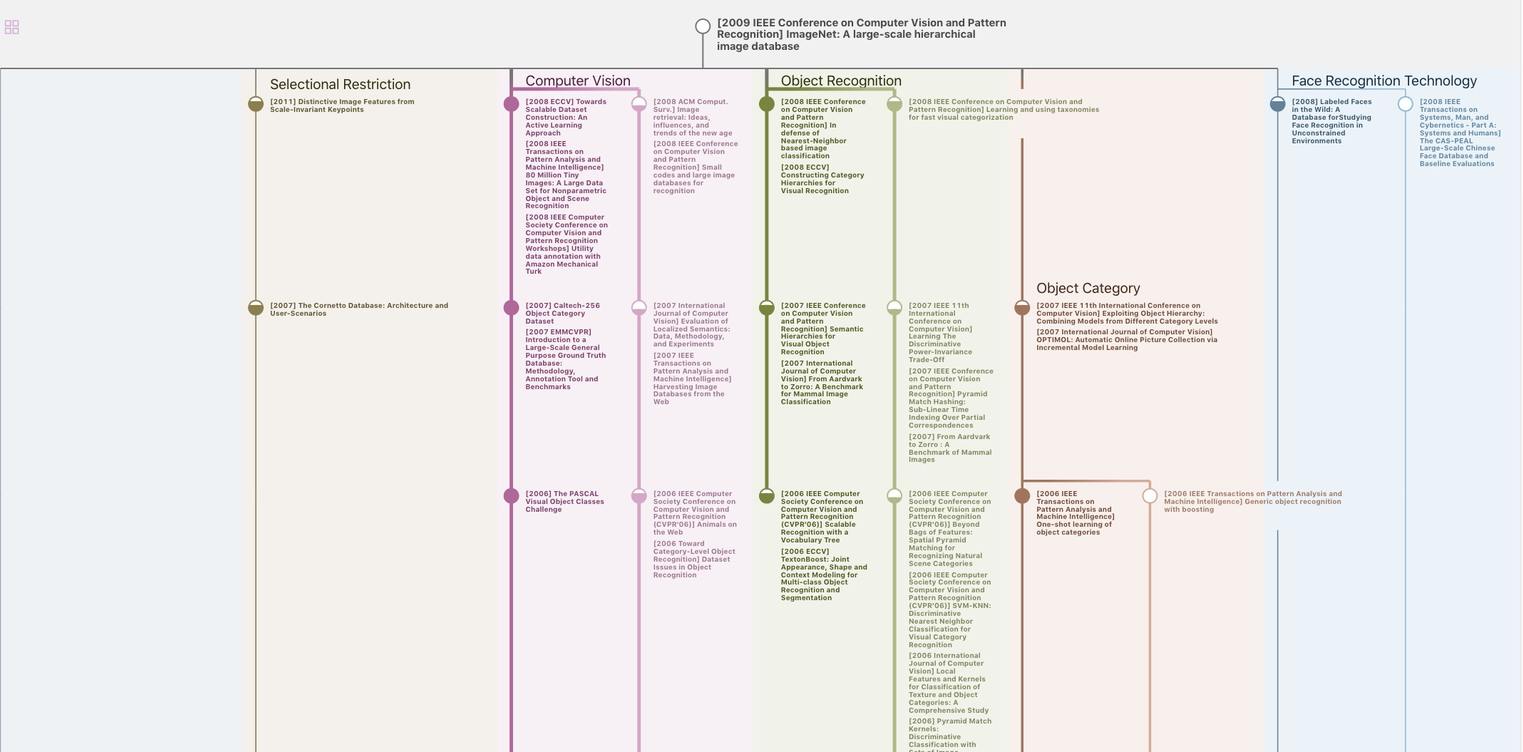
生成溯源树,研究论文发展脉络
Chat Paper
正在生成论文摘要