Single-Stage Intake Gesture Detection Using CTC Loss and Extended Prefix Beam Search
IEEE Journal of Biomedical and Health Informatics(2021)
摘要
Accurate detection of individual intake gestures is a key step towards automatic dietary monitoring. Both inertial sensor data of wrist movements and video data depicting the upper body have been used for this purpose. The most advanced approaches to date use a two-stage approach, in which (i) frame-level intake probabilities are learned from the sensor data using a deep neural network, and then (ii) sparse intake events are detected by finding the maxima of the frame-level probabilities. In this study, we propose a single-stage approach which directly decodes the probabilities learned from sensor data into sparse intake detections. This is achieved by weakly supervised training using Connectionist Temporal Classification (CTC) loss, and decoding using a novel extended prefix beam search decoding algorithm. Benefits of this approach include (i) end-to-end training for detections, (ii) simplified timing requirements for intake gesture labels, and (iii) improved detection performance compared to existing approaches. Across two separate datasets, we achieve relative F
1
score improvements between 1.9% and 6.2% over the two-stage approach for intake detection and eating/drinking detection tasks, for both video and inertial sensors.
更多查看译文
关键词
Algorithms,Gestures,Neural Networks, Computer,Pattern Recognition, Automated,Wrist
AI 理解论文
溯源树
样例
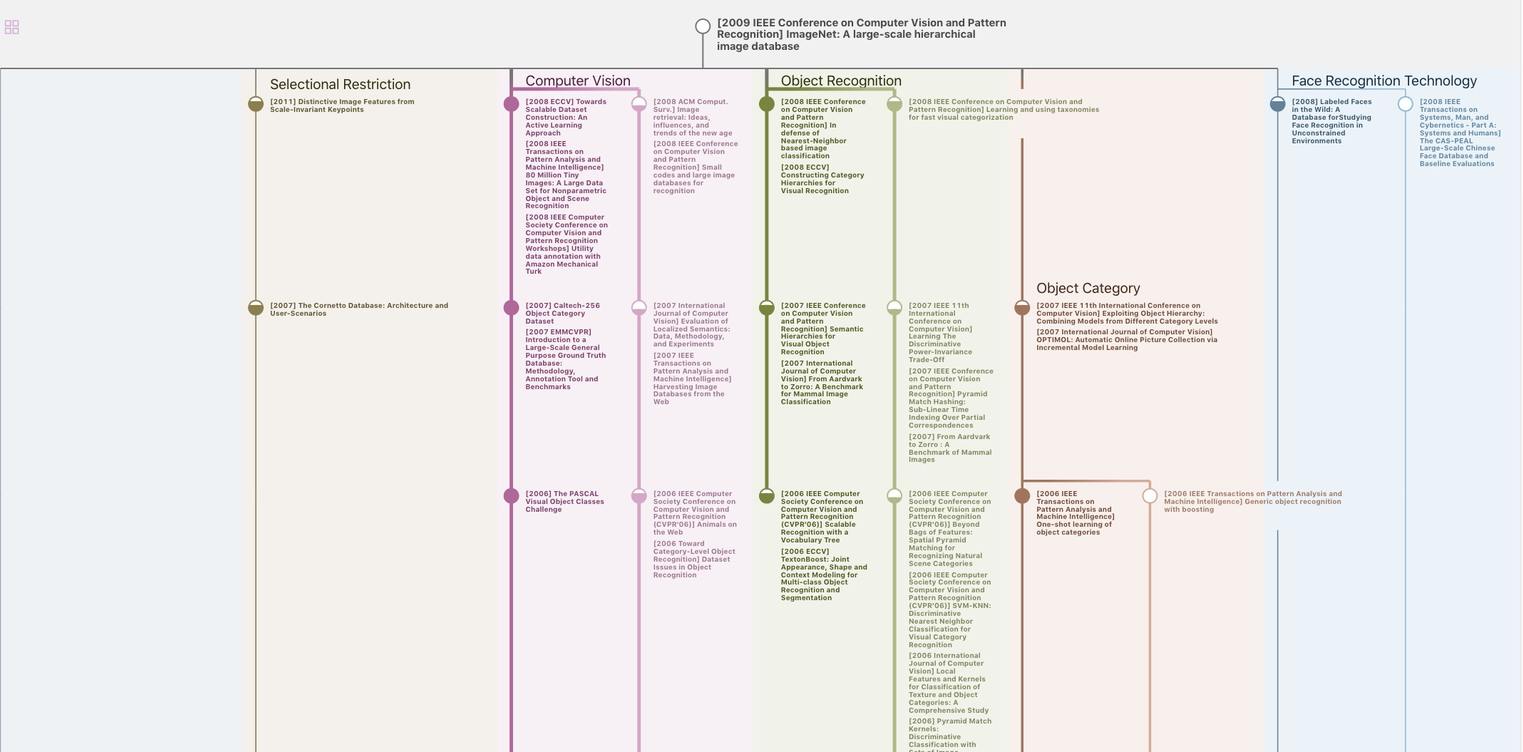
生成溯源树,研究论文发展脉络
Chat Paper
正在生成论文摘要