Deep Learning Segmentation Of General Interventional Tools In Two-Dimensional Ultrasound Images
MEDICAL PHYSICS(2020)
摘要
Purpose: Many interventional procedures require the precise placement of needles or therapy applicators (tools) to correctly achieve planned targets for optimal diagnosis or treatment of cancer, typically leveraging the temporal resolution of ultrasound (US) to provide real-time feedback. Identifying tools in two-dimensional (2D) images can often be time-consuming with the precise position difficult to distinguish. We have developed and implemented a deep learning method to segment tools in 2D US images in near real-time for multiple anatomical sites, despite the widely varying appearances across interventional applications.Methods: A U-Net architecture with a Dice similarity coefficient (DSC) loss function was used to perform segmentation on input images resized to 256 x 256 pixels. The U-Net was modified by adding 50% dropouts and the use of transpose convolutions in the decoder section of the network. The proposed approach was trained with 917 images and manual segmentations from prostate/gynecologic brachytherapy, liver ablation, and kidney biopsy/ablation procedures, as well as phantom experiments. Real-time data augmentation was applied to improve generalizability and doubled the dataset for each epoch. Postprocessing to identify the tool tip and trajectory was performed using two different approaches, comparing the largest island with a linear fit to random sample consensus (RANSAC) fitting.Results: Comparing predictions from 315 unseen test images to manual segmentations, the overall median [first quartile, third quartile] tip error, angular error, and DSC were 3.5 [1.3, 13.5] mm, 0.8 [0.3, 1.7]degrees, and 73.3 [56.2, 82.3]%, respectively, following RANSAC postprocessing. The predictions with the lowest median tip and angular errors were observed in the gynecologic images (median tip error: 0.3 mm; median angular error: 0.4 degrees) with the highest errors in the kidney images (median tip error: 10.1 mm; median angular error: 2.9 degrees). The performance on the kidney images was likely due to a reduction in acoustic signal associated with oblique insertions relative to the US probe and the increased number of anatomical interfaces with similar echogenicity. Unprocessed segmentations were performed with a mean time of approximately 50 ms per image.Conclusions: We have demonstrated that our proposed approach can accurately segment tools in 2D US images from multiple anatomical locations and a variety of clinical interventional procedures in near real-time, providing the potential to improve image guidance during a broad range of diagnostic and therapeutic cancer interventions. (C) 2020 American Association of Physicists in Medicine
更多查看译文
关键词
automatic general needle segmentation, convolutional neural network, interventional procedures, real-time, ultrasound image guidance
AI 理解论文
溯源树
样例
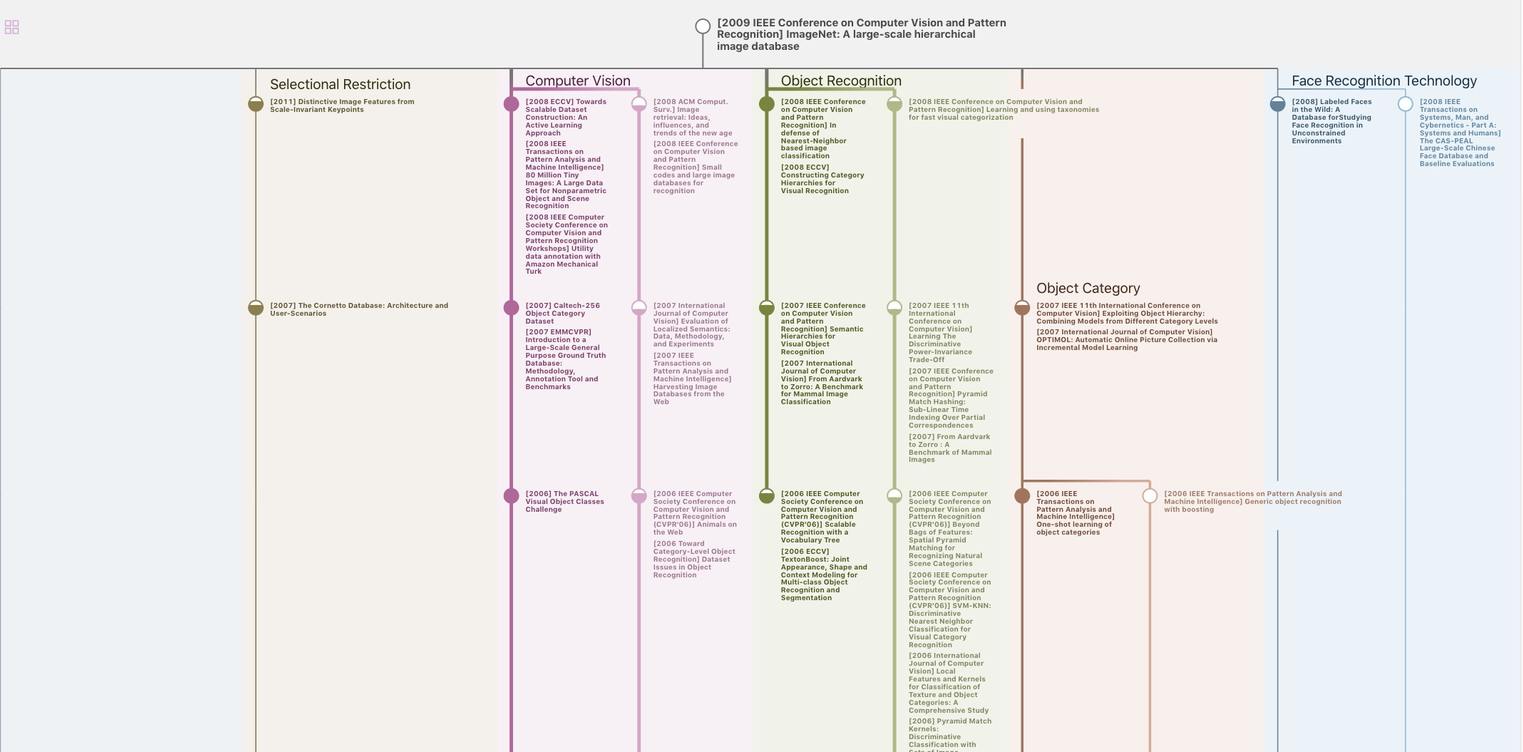
生成溯源树,研究论文发展脉络
Chat Paper
正在生成论文摘要