Attentive Fusion Enhanced Audio-Visual Encoding for Transformer Based Robust Speech Recognition
APSIPA(2020)
摘要
Audio-visual information fusion enables a performance improvement in speech recognition performed in complex acoustic scenarios, e.g., noisy environments. It is required to explore an effective audio-visual fusion strategy for audiovisual alignment and modality reliability. Different from the previous end-to-end approaches where the audio-visual fusion is performed after encoding each modality, in this paper we propose to integrate an attentive fusion block into the encoding process. It is shown that the proposed audio-visual fusion method in the encoder module can enrich audio-visual representations, as the relevance between the two modalities is leveraged. In line with the transformer-based architecture, we implement the embedded fusion block using a multi-head attention based audiovisual fusion with one-way or two-way interactions. The proposed method can sufficiently combine the two streams and weaken the over-reliance on the audio modality. Experiments on the LRS3-TED dataset demonstrate that the proposed method can increase the recognition rate by 0.55%, 4.51% and 4.61% on average under the clean, seen and unseen noise conditions, respectively, compared to the state-of-the-art approach.
更多查看译文
关键词
performance improvement,audio-visual fusion strategy,audio-visual information fusion,transformer based robust speech recognition,fusion enhanced audio-visual encoding,recognition rate,audio modality,audiovisual fusion,multihead attention,embedded fusion block,transformer-based architecture,audio-visual representations,encoder module,audio-visual fusion method,encoding process,attentive fusion block,previous end-to-end approaches,audiovisual alignment
AI 理解论文
溯源树
样例
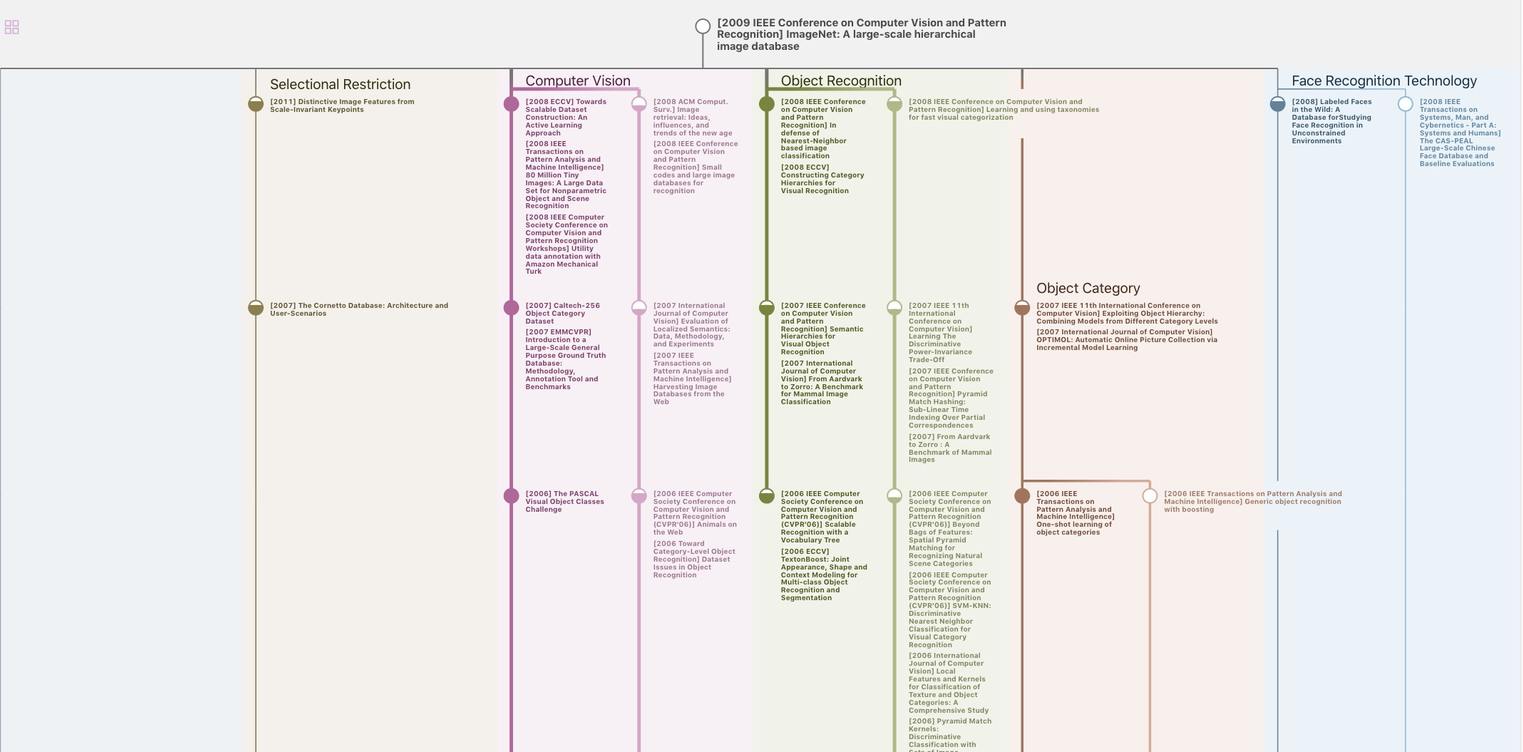
生成溯源树,研究论文发展脉络
Chat Paper
正在生成论文摘要