Brr-Net: A Tandem Architectural Cnn-Rnn For Automatic Body Region Localization In Ct Images
MEDICAL PHYSICS(2020)
摘要
Purpose Automatic identification of consistently defined body regions in medical images is vital in many applications. In this paper, we describe a method to automatically demarcate the superior and inferior boundaries for neck, thorax, abdomen, and pelvis body regions in computed tomography (CT) images. Methods For any three-dimensional (3D) CT imageI, following precise anatomic definitions, we denote the superior and inferior axial boundary slices of the neck, thorax, abdomen, and pelvis body regions byNS(I),NI(I),TS(I),TI(I),AS(I),AI(I),PS(I), andPI(I), respectively. Of these, by definition,AI(I) = PS(I), and so the problem reduces to demarcating seven body region boundaries. Our method consists of a two-step approach. In the first step, a convolutional neural network (CNN) is trained to classify each axial slice inIinto one of nine categories: the seven body region boundaries, plus legs (defined as all axial slices inferior toPI(I)), and the none-of-the-above category. This CNN uses a multichannel approach to exploit the interslice contrast, providing the neural network with additional visual context at the body region boundaries. In the second step, to improve the predictions for body region boundaries that are very subtle and that exhibit low contrast, a recurrent neural network (RNN) is trained on features extracted by CNN, limited to a flexible window about the predictions from the CNN. Results The method is evaluated on low-dose CT images from 442 patient scans, divided into training and testing sets with a ratio of 70:30. Using only the CNN, overall absolute localization error forNS(I),NI(I),TS(I),TI(I),AS(I),AI(I), andPI(I)expressed in terms of number of slices (nS) is (mean +/- SD): 0.61 +/- 0.58, 1.05 +/- 1.13, 0.31 +/- 0.46, 1.85 +/- 1.96, 0.57 +/- 2.44, 3.42 +/- 3.16, and 0.50 +/- 0.50, respectively. Using the RNN to refine the CNN's predictions for select classes improved the accuracy ofTI(I)andAI(I)to: 1.35 +/- 1.71 and 2.83 +/- 2.75, respectively. This model outperforms the results achieved in our previous work by 2.4, 1.7, 3.1, 1.1, and 2 slices, respectively forTS(I),TI(I),AS(I),AI(I) = PS(I), andPI(I)classes with statistical significance. The model trained on low-dose CT images was also tested on diagnostic CT images forNS(I),NI(I),andTS(I)classes; the resulting errors were: 1.48 +/- 1.33, 2.56 +/- 2.05, and 0.58 +/- 0.71, respectively. Conclusions Standardized body region definitions are a prerequisite for effective implementation of quantitative radiology, but the literature is severely lacking in the precise identification of body regions. The method presented in this paper significantly outperforms earlier works by a large margin, and the deviations of our results from ground truth are comparable to variations observed in manual labeling by experts. The solution presented in this work is critical to the adoption and employment of the idea of standardized body regions, and clears the path for development of applications requiring accurate demarcations of body regions. The work is indispensable for automatic anatomy recognition, delineation, and contouring for radiation therapy planning, as it not only automates an essential part of the process, but also removes the dependency on experts for accurately demarcating body regions in a study.
更多查看译文
关键词
automatic anatomy recognition, body region identification, computed tomography (CT), deep learning
AI 理解论文
溯源树
样例
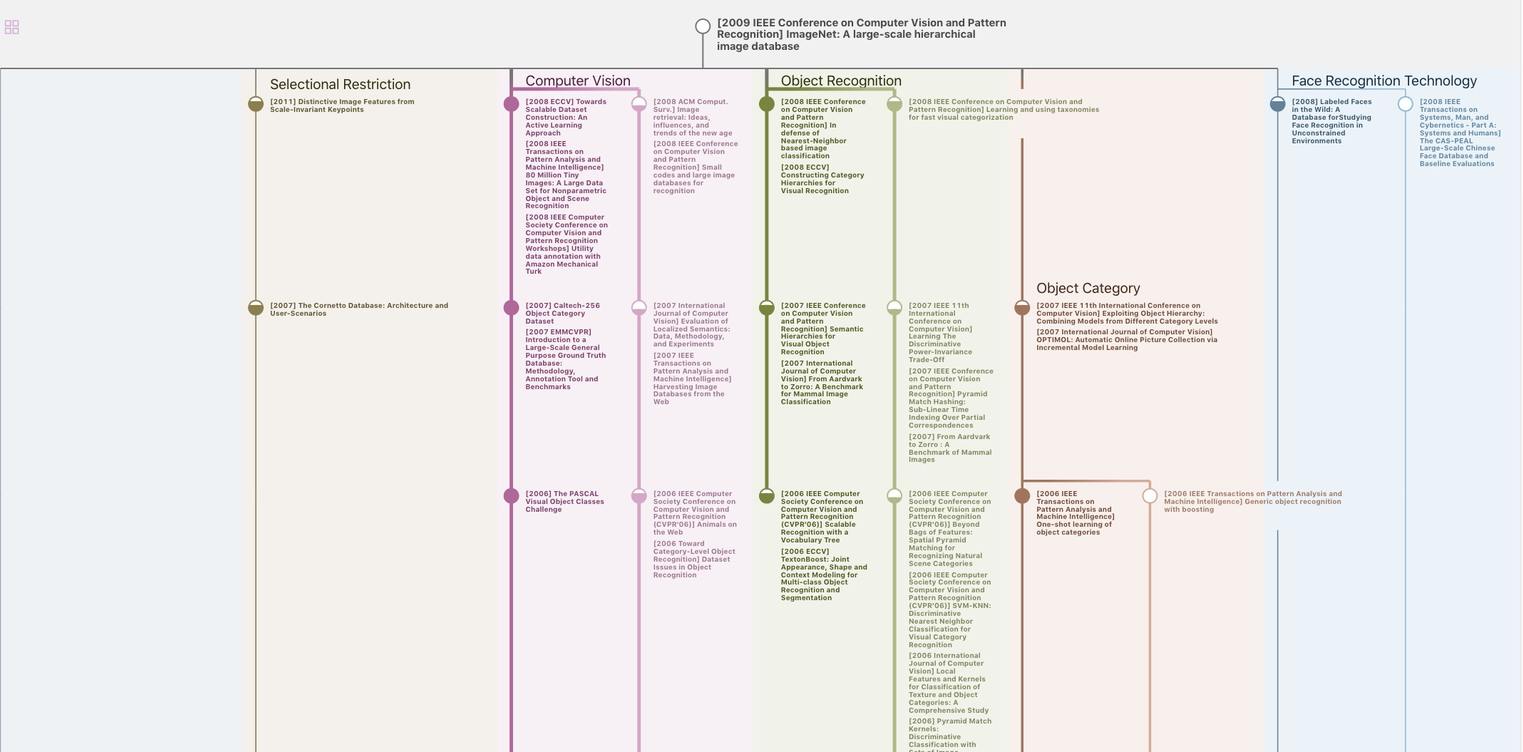
生成溯源树,研究论文发展脉络
Chat Paper
正在生成论文摘要